- 1Department of Pathology, Instituto Nacional de Enfermedades Neoplasicas, Lima, Peru
- 2Academic Department of Medical Microbiology, Universidad Nacional Mayor de San Marcos, Lima, Peru
- 3Department of Infectious Diseases, Instituto Nacional de Enfermedades Neoplasicas, Lima, Peru
- 4Academic Department of Medical Technologist, Universidad Nacional Mayor de San Marcos, Lima, Peru
- 5Department of Radiodiagnosis, Instituto Nacional de Enfermedades Neoplasicas, Lima, Peru
- 6Department of Critical Care Medicine, Instituto Nacional de Enfermedades Neoplasicas, Lima, Peru
- 7Professional School of Human Medicine, Universidad Privada San Juan Bautista, Lima, Peru
- 8Professional School of Human Medicine, Universidad de Piura, Lima, Peru
- 9Department of Medical Oncology, Instituto Nacional de Enfermedades Neoplasicas, Lima, Peru
Abstract
Introduction: The role of the type, stage and status of cancer in the outcome of COVID-19 remains unclear. Moreover, the characteristic pathological changes of severe COVID-19 reveled by laboratory and radiological findings are similar to those due to the development of cancer itself and antineoplastic therapies.
Objective: To identify potential predictors of mortality of COVID-19 in cancer patients.
Materials and methods: A retrospective and cross-sectional study was carried out in patients with clinical suspicion of COVID-19 who were confirmed for COVID-19 diagnosis by RT-PCR testing at the National Institute of Neoplastic Diseases between April and December 2020. Demographic, clinical, laboratory and radiological data were analyzed. Statistical analyses included area under the curve and univariate and multivariate logistic regression analyses.
Results: A total of 226 patients had clinical suspicion of COVID-19, the diagnosis was confirmed in 177 (78.3%), and 70/177 (39.5%) died. Age, active cancer, leukocyte count ≥12.8 × 109/L, urea ≥7.4 mmol/L, ferritin ≥1,640, lactate ≥2.0 mmol/L, and lung involvement ≥35% were found to be independent predictors of COVID-19 mortality.
Conclusion: Active cancer represents the main prognosis factor of death, while the role of cancer stage and type is unclear. Chest CT is a useful tool in the prognosis of death from COVID-19 in cancer patients. It is a challenge to establish the prognostic utility of laboratory markers as their altered values it could have either oncological or pandemic origins.
Introduction
COVID-19 disease leads to severe pneumonia, metabolic acidosis, coagulation dysfunction, multiple organ failure, and eventually, death [1–4]. Cancer patients usually have a compromised immune system with a higher risk of death in COVID-19 compared to those without cancer [5–8], but it is not clear if the type, stage and status of cancer play a role in the outcome of the disease.
COVID-19 diagnosis is based on clinical evaluation and confirmed by the detection of viral RNA in respiratory samples [9]. Furthermore, certain blood laboratory parameters and chest computed tomography (CT) findings could reveal characteristic pathological changes and the clinical course of COVID-19 in oncology patients. Altered levels of C-reactive protein (CRP), neutrophil and lymphocyte counts, ferritin, D-dimer, and lactate dehydrogenase [10–12], as well as a high CO-RADS score and the presence of some abnormal chest CT findings have been associated with the presentation of severe complications of COVID-19 [13, 14].
However, malignant neoplasms lead to similar laboratory and radiological alteration findings due to pathological events in the development of cancer itself (acute renal failure, disseminated intravascular coagulation, impaired cellular immunity, organ failure, respiratory failure) [15–21] and antineoplastic therapies (immunosuppression, hepatotoxicity) [22, 23]. Therefore, it is unknown whether these potential predictors are useful for detecting the clinical course of the disease in cancer patients.
We aimed to identify predictors of mortality of COVID-19 in cancer patients by the joint study of the clinical characteristics, laboratory and radiological findings, and their association with a higher risk of a fatal outcome.
Materials and methods
Study design and population
This retrospective and cross-sectional study was carried out in patients hospitalized at the Instituto Nacional de Enfermedades Neoplasicas (INEN) in Lima—Peru between April and December 2020. The study sample included patients who presented with a clinical suspicion of COVID-19 and were confirmed for COVID-19 diagnosis by RT-PCR testing. Patients with a clinical or radiological diagnosis of COVID-19 without a positive RT-PCR test were excluded. When patients had two positive results for COVID-19 by RT-PCR, only the first one was considered.
Clinical, laboratory, radiological and outcome data
Demographic, clinical, laboratory, radiological and outcomes data were collected blind from the medical records and the INEN informatic system. Blood sample tests and chest CT scans were performed within 24 h after sampling for the COVID-19 molecular diagnosis. While clinical outcome, mortality or survival, was considered until 30 days after the molecular study was performed. A specialist physician from the Department of Infectious Diseases evaluated the records of the clinical presentation, while two specialist physicians from the Department of Medical Oncology evaluated the stage and status of cancer in the patients. Two specialist physicians from the Department of Radiodiagnosis evaluated the chest CT images to determine the presence of abnormal findings, the percentage of affected lung, and the COVID-19 Reporting and Data System (CO-RADS) score classification system.
To determine the percentage of affected lung, each of the five lung lobes was scored visually on a scale from 0 to 5, (where 0 indicates no involvement; 1, less than 5%; 2, 5%–25%; 3, 26%–49%; 4, 50%–75%; and 5, more than 75%). The total CT score was the sum of the individual lobar scores and ranged from 0 (no involvement) to 25 (maximum involvement) [24]. The CO-RADS system assessed lung damage on a chest CT to predict the likelihood of COVID-19 pneumonia using a scale from 1 (very low) to 5 (very high). A high CO-RADS score indicates a high probability of COVID-19; thus, the grouped frequency of COVID-19 in categories 1, 2, 3, 4 and 5 corresponds to 8.8%, 11.1%, 24.6%, 61.9% and 89.6% involvement, respectively) [25].
Definitions
Cases with clinical suspicion of COVID-19: Patients present any of the following symptoms: fever, cough, fatigue, headache, dyspnea, myalgia, diarrhea, tachypnea, chest pain, anosmia, and ageusia.
Confirmed cases of COVID-19: Patients who tested positive for COVID-19 in the molecular study (PCR-RT) from nasopharyngeal swab samples.
Active cancer: Presence of cancer progression or recurrence after treatment.
Advanced cancer: Presence of distant metastatic disease.
Clinical scenario: Clinical presentation of patients according to WHO [26].
Compliance with ethics guidelines
All the cases in this study were part of the medical care routine of the INEN. No informed consent from any patient was obtained since this study used laboratory test registers, radiological reports of the INEN informatic system, and patient medical records in obtaining data which were used protecting the identity of the patients. The protocol was presented to the Research Committee of the INEN and approved for its implementation with designated protocol number INEN 20-49.
Statistical analysis
Categorical variables were analyzed using the chi-squared or Fisher’s exact test, as appropriate, and presented as frequencies and percentages. Quantitative variables were described as means and standard deviation or medians and interquartile ranges, depending on the distribution of the data. Quantitative variables were evaluated using the Student’s t-test when there was a normal distribution of the data and, when not, using the Mann-Whitney U test. Receiver operating characteristic (ROC) curve analysis provided the sensitivity and specificity of the laboratory tests and radiological findings for the prediction of mortality from COVID-19. In addition, it allowed us to obtain the best cut-off points for the categorization of these variables according to the Youden Index. Finally, the univariate and multivariate regression analyses were performed to obtain the odds ratio (OR) as a measure of association between the variables and the mortality prediction of COVID-19, considering only the associated variables in the bivariate analysis for building the final multivariate model. For all analyses, statistical significance was set at p < 0.05. All analyses were performed using Stata version 14.0.
Results
During the study period, 226 patients had clinical suspicion of COVID-19 from which 177 (78.3%) were confirmed. Of the 177 positive cases of COVID-19, most patients were between 16 and 59 years old (84, 47.5%), male (96, 54.2%), had solid tumors (95, 53.7%), non-advanced stage (104, 58.8%) and active status cancer (111, 62.7%). Of these, 70 (39.5%) died, one-third of the deaths of patients with COVID-19 occurred within the first 5 days (24/70, 34.4%), and the vast majority (52/70, 74.3%) had died by day 15 after molecular study sampling.
Prediction of COVID-19 mortality
The deceased patients were older (62.5 vs. 42.0; p ≤ 0.001), had severe-critical clinical scenario (56.6% vs. 26.7%; p ≤ 0.001) and active cancer (48.7% vs. 24.2%; p = 0.001) (Table 1).
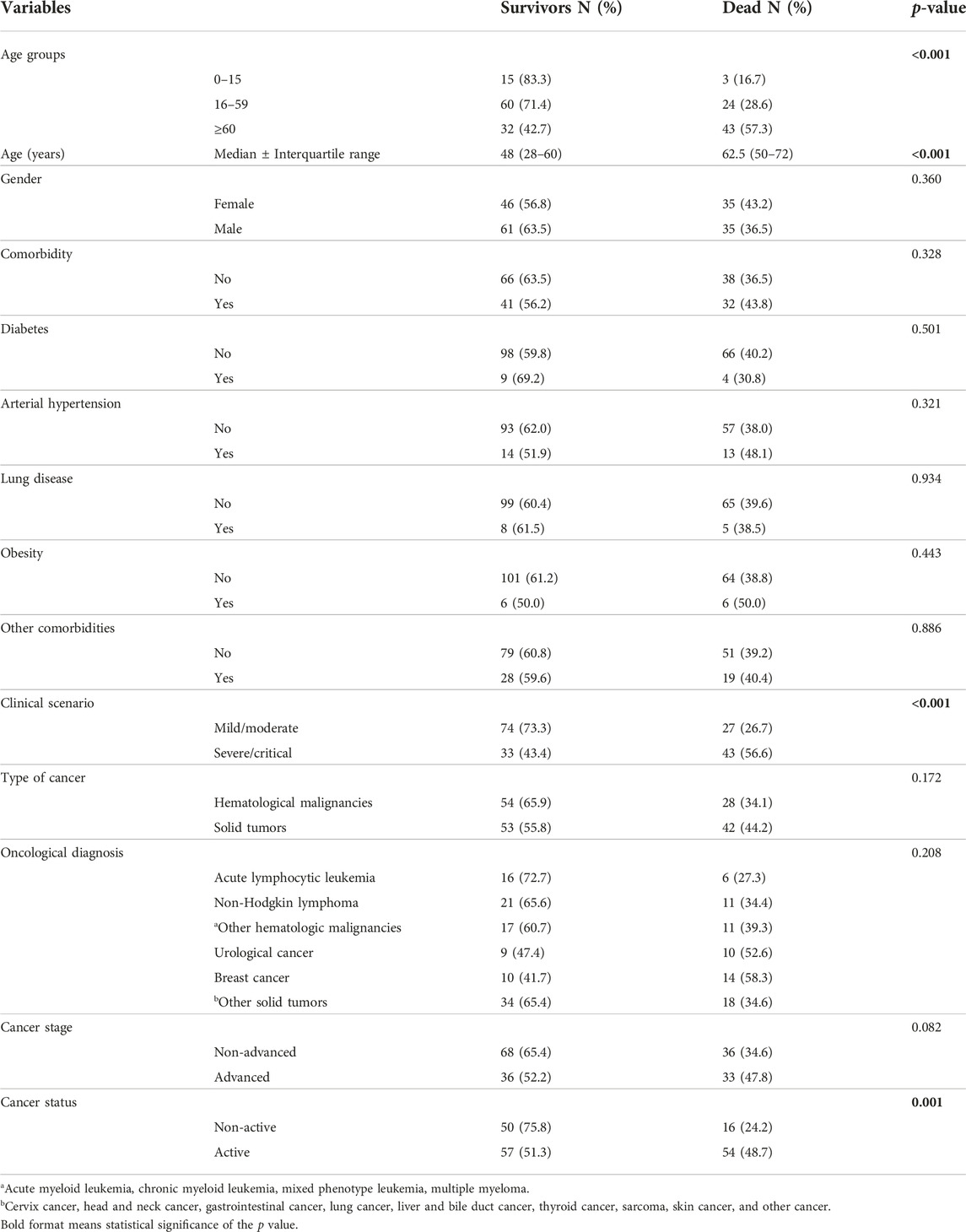
TABLE 1. Demographic, clinical and oncological characteristics of 177 cases of cancer patients with COVID-19 according to mortality.
Likewise, these patients had a higher leukocyte count (10.4×109/L vs. 6.6×109/L; p = 0.001), neutrophil count (8.63 × 109/L vs. 5.26 × 109/L; p = 0.001), RNL (15.1 vs. 7.8; p = 0.001), D-dimer (2,136 ng/mL vs. 1,034 ng/mL; p = 0.002), LDH (329 U/L vs. 249 U/L; p = 0.004), urea (6.0 mmol/L vs. 4.0 mmol/L; p ≤ 0.001), creatinine (56 umol/L vs. 48 umol/L; p = 0.018, lactate (1.9 mmol/L vs. 1.3 mmol/L; p ≤ 0.001), lung involvement (40% vs. 20%; p ≤ 0.001) and crazy paving (56.3% vs. 32.3%) (Table 2).
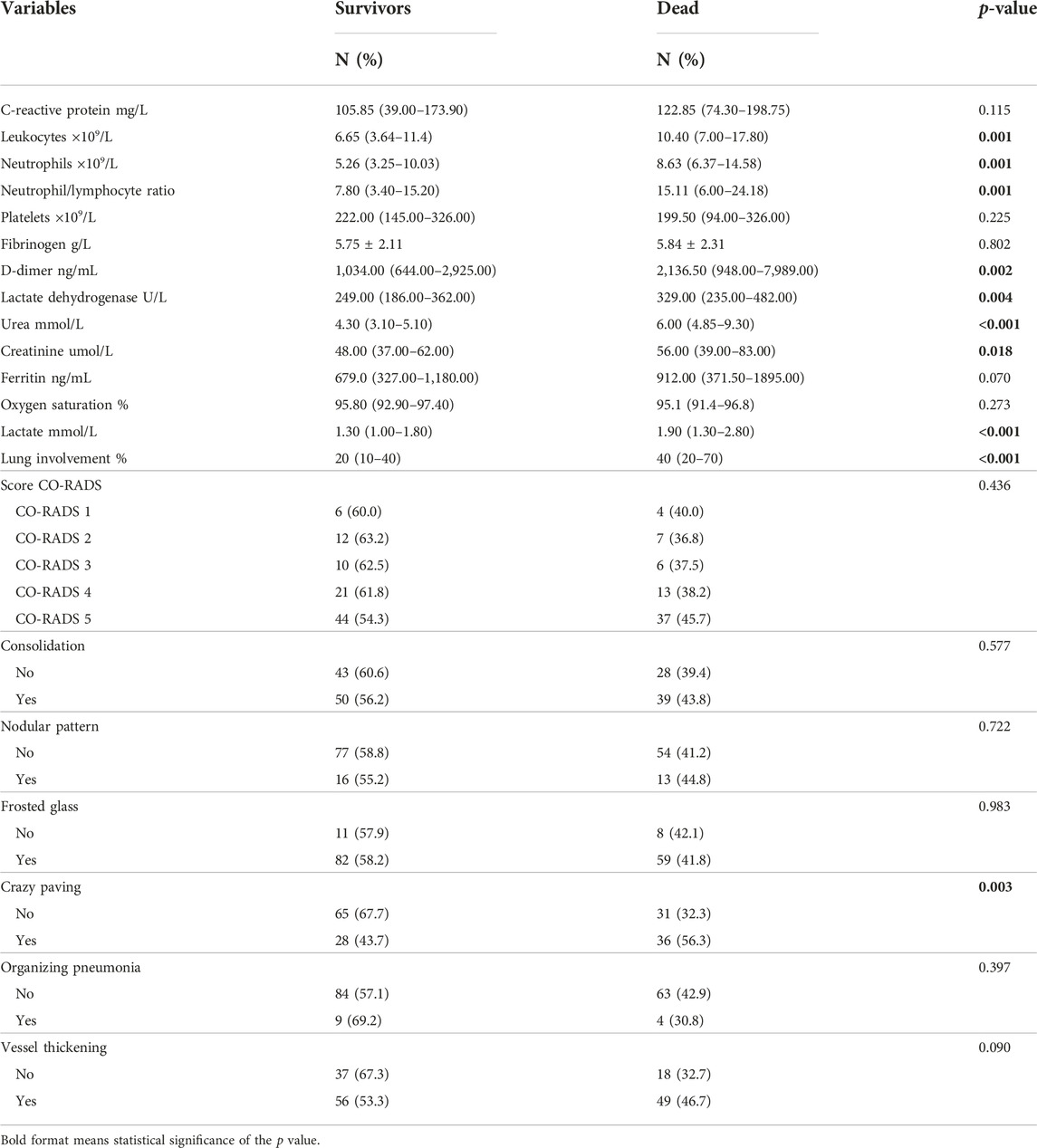
TABLE 2. Comparison of laboratory tests and CT findings in 177 cancer patients with COVID-19 according to mortality.
The evaluation of the area under the curve showed that urea ≥7.4 mmol/L had the highest ability to predict death, with AUC 0.751 (S = 40.63% and E = 91.75%), followed by lactate ≥2.0 mmol/L, AUC 0.686 (S = 47.54% and E = 86.49%) and lung involvement ≥35%, AUC 0.662 (S = 59.70%, E = 66.67%) (Table 3).
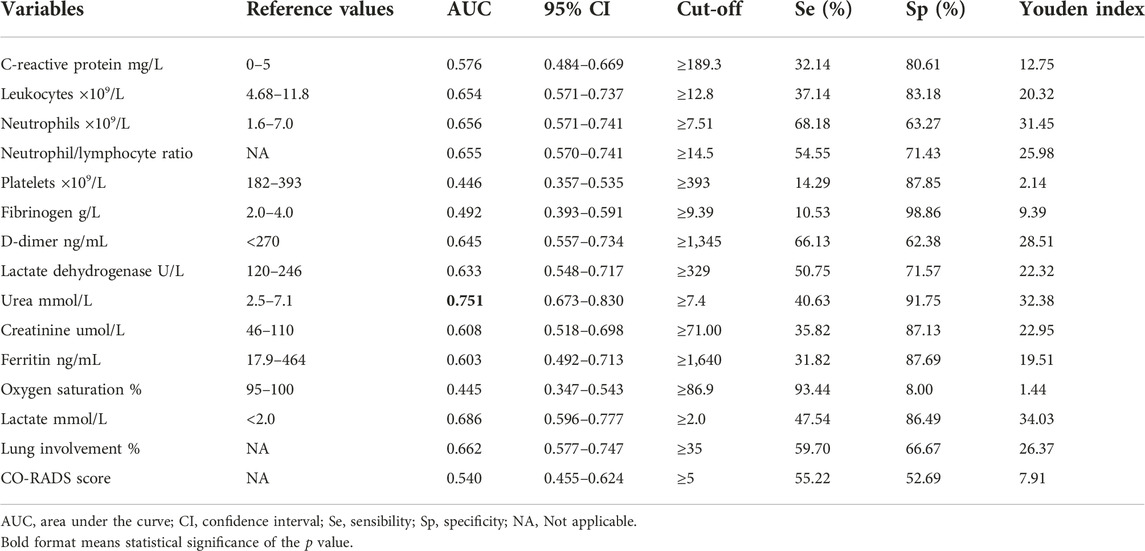
TABLE 3. Prognostic capacity of death of laboratory tests and CT findings in cancer patients with COVID-19.
Finally, in the bivariate regression analysis, a higher probability of death was found in patients over 60 years of age (OR 6.72; p = 0.005) compared to those under 16 years of age and with increasing age per year (OR 1.04; p ≤ 0.001), severe-critical clinical scenario (OR 3.57; p ≤ 0.001), active cancer (OR 2.96; p = 0.002), advanced cancer (OR 1.61; p = 0.082), leukocyte count ≥12.8 × 109/L (OR 2.92; p = 0.003), neutrophil count ≥7.51 × 109/L (OR 3.55; p ≤ 0.001), RNL ≥14.5 (OR 3.00; p = 0.001), fibrinogen ≥9.39 g/L (OR 9.94; p = 0.035), D-dimer ≥1,345 ng/mL (OR 2.57; p = 0.003), LDH ≥329 U/L (OR 2.54; p = 0.004), urea ≥7.4 mmol/L (OR 7.31; p ≤ 0.001), creatinine ≥71 umol/L (OR 3.77; p = 0.001), ferritin ≥1,640 (OR 3.09; p = 0.017), lactate ≥2.0 mmol/L (OR 6.86; p ≤ 0.001), lung involvement ≥35% (OR 3.27; p ≤ 0.001), crazy paving (OR 2.58; p = 0.001) and vessel thickening (OR 1.91; p = 0.011), and a lower probability in patients with oxygen saturation ≥86.9% (OR 0.45; p = 0.029). Multivariate analysis found an association with a higher probability of death with increasing age per year (OR 1.04; p = 0.001), active cancer (OR 7.56; p≤0.001), leukocyte count ≥12.8 × 109/L (OR 3.00; p = 0.022), urea ≥7.4 mmol/L (OR 3.20; p = 0.034), ferritin ≥1,640 (OR 7.22; p = 0.005), lactate ≥2.0 mmol/L (OR 4.79; p = 0.002) and lung involvement ≥35% (OR 4.34; p = 0.002) (Table 4).
Discussion
The mortality rate in our population during the first wave of the pandemic was higher than that found in cancer patients with COVID-19, whose mortality rates were highly variable [27]. It could be related to underlying clinical conditions. We emphasize that our study was conducted only in hospitalized patients, most of whom had active cancer. In addition, numerous deaths occurred shortly after the diagnosis of COVID-19, which shows delayed medical care resulting from the impact of the pandemic on hospital capacity in our country [28]. The high in-hospital mortality rate was related to the critical baseline condition of the patients.
Oncological characteristics could play a role in the outcome of COVID-19 in our population. The strong association between active cancer stage and an increased risk of death coincides with the findings of some studies [10, 29–32]. Active cancer leads to prothrombotic and proinflammatory conditions that concur with alterations in the immune system due to the development of cancer and antineoplastic treatments [33, 34]. Moreover, SARS-CoV-2 infection results in immune alterations and thrombotic events. Therefore, this could lead to an unfavorable prognosis in patients with cancer and COVID-19 [35, 36]. Advanced or metastatic cancer has been associated with an increased risk of death [37]. In our study, we observed this association, although it was not significant. Findings in this regard are contradictory; some studies have found it to be a risk factor for death in COVID-19 [10, 11, 27, 32], while others have not [12, 38–40]. In contrast, the non-association between the type of cancer, solid tumor or hematological neoplasm, and the probability of death in COVID-19 patients coincides with several studies [10–12, 27, 30–32, 39].
Active cancer represents the main vulnerability of cancer patients in the pandemic, otherwise there is still a need to better understand the possible role of the cancer stage and the type of neoplasia in the outcome of COVID-19.
Mortality in cancer patients with COVID-19 increases with each year of age. Older age leads to a deficiency in the immune response, resulting in a higher risk of death in COVID-19 patients [41]. The strength and direction of the association between age and COVID-19 mortality have been consistently found across multiple studies [10–12, 27, 29–32, 38–40].
The alteration of some laboratory parameters could be a manifestation of the pathophysiology of the disease, but it could also be due to cancer itself.
The high serum concentrations of lactate and, in particular, of ferritin could be the result of a sustained and hyperactive inflammatory response in the COVID-19 patient [3]. Increasing concentrations of serum ferritin, according to the proposed cut-off values, were strongly associated with the mortality of COVID-19 in our population. Serum ferritin levels increase because of cell damage and severe uncontrolled inflammatory conditions [41, 42] including malignant diseases and their progression [43, 44]. The threshold value evaluated (≥1,640 ng/mL) represent a significant increase in serum concentration and correlate with a hyperinflammatory state and a severe clinical course in COVID-19 [45, 46]. Moreover, the increase in serum ferritin has been associated with the severity and mortality of COVID-19 in oncology population, even with lower threshold values [11]. In contrast, a slight increase in serum lactate was associated with mortality. Lactate is a metabolite that is increased in the blood during tissue hypoxia and hypermetabolism, which results in tissue damage and organ failure [47, 48]. Hyperlactatemia (lactate >2.0 mmol/L) is associated with septic shock and mortality in cancer patients with a high incidence of sepsis-related morbidity and mortality [49]. Also, increased values of this analyte in blood, similar to the threshold evaluated in our study (≥2.0 mmol/L), have been found to be a predictor of mortality for COVID-19 in cancer patients, although with a higher threshold value [11]. Serum urea level was another biochemical marker of mortality in the present study. Measurement of uremia is routinely performed to evaluate renal function and its increase as an indicator of renal insufficiency [50, 51]. Although renal insufficiency is a frequent clinical condition in patients with cancer [16, 18], with the consequent increase in serum urea, it is also an important sequela of COVID-19 [52, 53]. The study of blood urea had a good prognostic capacity and association with mortality, although with a slightly higher threshold value (≥7.4 mmol/L). This could indicate the beginning of the manifestation of kidney damage and could also be due to the presence of renal comorbidity in some patients. Increased serum levels of urea (in the form of BUN) have been associated with higher mortality for COVID-19 in cancer patients [54]. Monitoring the evolution of uremia and the study of other complementary tests could contribute to a better understanding of this prognostic factor.
In the hematological study, the leukocyte count seems to be an unspecified marker. An increased leukocyte count represents an inflammatory state resulting from the innate immune response to infection [55, 56]. Likewise, leukocytes are also increased in malignant neoplasms due to the close relationship between the development and progression of cancer and a state of systemic inflammation [57–59]. The increased leukocyte count would indicate a severe inflammatory reaction as a consequence of COVID-19 in cancer patients, even with lower threshold values [12]. However, this finding must be evaluated by considering the oncological context in which many neoplasms frequently maintain leukocytosis, as a subclinical proinflammatory state. In contrast, the total leukocyte count may be affected by the toxicity of antineoplastic therapy in patients under treatment [60, 61]. Contrary to the well-known association found between coagulation markers and COVID-19, we observed no such association. The values of D-dimer, fibrinogen and platelets are frequently altered due to the complication of cancer and its treatment, especially when the disease is active [62, 63].
Although we found some laboratory markers associated with mortality, establishing the prognostic utility of these parameters requires considering that their increased concentration may be due to the underlying neoplasm and/or to the manifestation of the severity of COVID-19.
Chest CT showed a prognostic association with death in COVID-19 through the evaluation of the percentage of affected lungs. To the best of our knowledge there is no association studies between chest CT score system and COVID-19 in oncology patients. However, chest CT can be useful in the study of COVID-19 in cancer patients in which it can show atypical images with few or solitary abnormal findings; therefore, rare or subtle patterns can characterize SARS-CoV-2 infection [13].
CT scoring could help to stratify patient risk and predict short-term outcome in COVID-19 pneumonia [64]. The expression of global pulmonary involvement, regardless of the alteration type, allows us to predict clinical evolution [65], since with the semiquantitative scoring system to estimate lung involvement, all the abnormalities present in the CT are taken into account based on the affected area. Lung involvement score on chest CT in the general population associated with mortality were ≥15 (lung involvement ≥60%) [66, 67] and ≥12.5 [68]. However, in our study, a lung CT score of ≥8.75 (lung involvement ≥35%) was associated with a higher probability of death, which could have been due to limited immune function, especially in patients with hematological neoplasms receiving antineoplastic treatment and with leukopenia [13]. The extent of lung involvement observed on CT, even at a low percentage, could help identify cancer patients at a high risk of death.
Although, there is limited data on chest CT for the diagnosis of COVID-19 pneumonia with a focus on cancer populations [14], in evaluating multiple and non-specific findings, chest CT is a useful tool in the prognosis of death from COVID-19 in cancer patients.
In summary, our clinical study attempted to clarify the role of cancer in the fatality of COVID-19 infection. We found several serum markers and imaging patterns of mortality, but mainly we identified active cancer, cancer progression or its recurrence after treatment, as a critical variable. Our findings derive from a comprehensive clinical, laboratory, and imaging analysis, highlighting the complex interplay of oncologic features and the pathophysiology of COVID-19 to predict the fatal outcome of the disease.
Limitations
Our study had some limitations that do not invalidate the results; on the contrary, they lead to the proposal of further evaluations to overcome them. First, the small size of the cohort, which probably prohibited to clarify or detect associations due to insufficient statistical power, as we only had access to the complete information of patients with COVID-19 for the study period of time. Second, recognized markers were not evaluated in the COVID-19 study, such as interleukin 6 and procalcitonin, which were not available or were economically unfeasible for developing countries such as ours. Third, neoplastic treatment, which can influence the total leukocyte count, was not analyzed in the study, although the toxic effect of this therapy occurs in all cell types. Finally, cases that had incomplete information could have generated some bias in the results, although the missing data were expected to be undifferentiated for the groups analyzed.
Conclusions
• Active cancer represents the main prognosis factor of death, while the role of cancer stage and type is unclear.
• Chest CT is a useful tool in the prognosis of death from COVID-19 in cancer patients.
• It is a challenge to establish the prognostic utility of laboratory markers as the alteration of their values can have either oncological or pandemic origins.
• Clinical, laboratory and radiological correlations can help improve the prognosis of death in cancer patients with pulmonary involvement due to COVID-19.
Data availability statement
The raw data supporting the conclusion of this article will be made available by the authors, without undue reservation.
Ethics statement
All the cases in this study were part of the medical care routine of the INEN. No informed consent from any patient was obtained since this study used laboratory test registers and patient medical records in obtaining data which were used protecting the identity of the patients. The protocol was presented to the Research Committee of the INEN and approved for its implementation with designated protocol number INEN 20-49.
Author contributions
FV-C and JV conceived and designed the study. JV, RR, AB, ML, TS, DP, YB, and MG acquired data. FV-C, RR, YB, CF, and MG analyzed the data, and FV-C, RR, AB, ML, TS, DP, YB, and MG-N wrote manuscript. All authors contributed to the article and approved the submitted version.
Conflict of interest
The authors declare that the research was conducted in the absence of any commercial or financial relationships that could be construed as a potential conflict of interest.
References
1. Guan, WJ, Niyi, Z, Hu, Y, Liang, WH, Ou, CQ, He, JX, et al. Clinical characteristics of coronavirus disease 2019 in China. New Engl J Med (2020) 382(18):1708–20. doi:10.1056/nejmoa2002032
2. Shi, M, Chen, L, Yang, Y, Zhang, J, Xu, J, Xu, G, et al. Analysis of clinical features and outcomes of 161 patients with severe and critical COVID-19: a multicenter descriptive study. J Clin Lab Anal (2020) 34(9):e23415. doi:10.1002/jcla.23415
3. Wang, D, Hu, B, Hu, C, Zhu, F, Liu, X, Zhang, J, et al. Clinical characteristics of 138 hospitalized patients with 2019 novel coronavirus-infected pneumonia in Wuhan, China. JAMA - J Am Med Assoc (2020) 323(11):1061–9. doi:10.1001/jama.2020.1585
4. Yakovenko, OK, Khanin, OG, Lotysh, VV, and Gryf, SL. Clinical features of severe COVID-19 with lethal outcome in volyn region residents. Ukrainian Pulmonology J (2021) 29(2):16–24. doi:10.31215/2306-4927-2021-29-2-16-24
5. Yang, L, Chai, P, Yu, J, and Fan, X. Effects of cancer on patients with COVID-19: a systematic review and meta-analysis of 63,019 participants. Cancer Biol Med (2021) 18:298–307. doi:10.20892/j.issn.2095-3941.2020.0559
6. Bernard, A, Cottenet, J, Bonniaud, P, Piroth, L, Arveux, P, Tubert-Bitter, P, et al. Comparison of cancer patients to non-cancer patients among COVID-19 inpatients at a national level. Cancers (Basel) (2021) 13(6):1436–15. doi:10.3390/cancers13061436
7. Rajeev Nadkarni, A, Vijayakumaran, SC, Gupta, S, and Divatia, JV. Mortality in cancer patients with COVID-19 who are admitted to an ICU or who have severe COVID-19: a systematic review and meta-analysis. JCO Glob Oncol (2021) 7:1286–305. doi:10.1200/go.21.00072
8. Wang, L, Sun, Y, Yuan, Y, Mei, Q, and Yuan, X. Clinical challenges in cancer patients with COVID-19: aging, immunosuppression, and comorbidities. Aging (2020) 12:24462–74. doi:10.18632/aging.104205
9. Health Organization, W. Guideline clinical management of COVID-19 patients: living guideline (2021). Available from: http://apps.who.int/bookorders (Accessed November 23, 2021).
10. Nader Marta, G, Colombo Bonadio, R, Nicole Encinas Sejas, O, Watarai, G, Mathias Machado, MC, Teixeira Frasson, L, et al. Outcomes and prognostic factors in a large cohort of hospitalized cancer patients with COVID-19. JCO Glob Oncol (2021) 7:1084–92. doi:10.1200/GO.21.00087
11. Mina, A, Galvez, C, Karmali, R, Mulcahy, M, Mi, X, Kocherginsky, M, et al. Outcomes of cancer patients with COVID-19 in a hospital system in the Chicago metropolitan area. Cancers (Basel) (2022) 14(9):2209. doi:10.3390/cancers14092209
12. Benderra, MA, Aparicio, A, Leblanc, J, Wassermann, D, Kempf, E, Galula, G, et al. Clinical characteristics, care trajectories and mortality rate of SARS-CoV-2 infected cancer patients: a multicenter cohort study. Cancers (Basel). (2021) 13(19):4749. doi:10.3390/cancers13194749
13. Katal, S, Aghaghazvini, L, and Gholamrezanezhad, A. Chest-CT findings of COVID-19 in patients with pre-existing malignancies; a pictorial review. Clin Imaging (2020) 67:121–9. doi:10.1016/j.clinimag.2020.06.004
14. Bourdoncle, S, Eche, T, McGale, J, Yiu, K, Partouche, E, Yeh, R, et al. Investigating of the role of CT scan for cancer patients during the first wave of COVID-19 pandemic. Res Diagn Interv Imaging (2022) 1:100004. doi:10.1016/j.redii.2022.100004
15. Levi, M. Disseminated intravascular coagulation in cancer patients. Best Pract Res Clin Haematol (2009) 22:129–36. doi:10.1016/j.beha.2008.12.005
16. Humphreys, BD, Soiffer, RJ, and Magee, CC. Renal failure associated with cancer and its treatment: an update. J Am Soc Nephrol (2005) 16:151–61. doi:10.1681/ASN.2004100843
17. Levi, M. Disseminated intravascular coagulation in cancer: an update. Semin Thromb Hemost (2019) 45(4):342–7. doi:10.1055/s-0039-1687890
18. Lameire, NH, Flombaum, CD, Moreau, D, and Ronco, C. Acute renal failure in cancer patients. Ann Med (2005) 37:13–25. doi:10.1080/07853890510007205
19. Kavanaugh, DY, and Carbone, DP. Immunologic dysfunction in cancer. Oncology (Williston Park) (1996) 10:927–51. doi:10.1016/s0889-8588(05)70376-2
20. Cedervall, J, Zhang, Y, and Olsson, AK. Tumor-induced NETosis as a risk factor for metastasis and organ failure. Cancer Res (2016) 76:4311–5. doi:10.1158/0008-5472.CAN-15-3051
21. Nava, S, and Cuomo, AM. Acute respiratory failure in the cancer patient: the role of non-invasive mechanical ventilation. Crit Rev Oncology/Hematology (2004) 51:91–103. doi:10.1016/j.critrevonc.2004.04.004
22. Vial, T, and Descotes, J. Immunosuppressive drugs and cancer. Toxicology (2003) 185(3):229–40. doi:10.1016/s0300-483x(02)00612-1
23. Ngo, D, Jia, JB, Green, CS, Gulati, AT, and Lall, C. Cancer therapy related complications in the liver, pancreas, and biliary system: an imaging perspective. Insight Imaging (2015) 6:665–77. doi:10.1007/s13244-015-0436-7
24. Pan, F, Ye, T, Sun, P, Gui, S, Liang, B, Li, L, et al. Time course of lung changes at chest CT during recovery from Coronavirus disease 2019 (COVID-19). Radiology (2020) 295(3):715–21. doi:10.1148/radiol.2020200370
25. Prokop, M, van Everdingen, W, van Rees Vellinga, T, van Ufford, HQ, Stöger, L, Beenen, L, et al. CO-RADS: a categorical CT assessment scheme for patients suspected of having COVID-19-definition and evaluation. Radiology (2020) 296(2):E97–104. doi:10.1148/radiol.2020201473
26. World Health Organization. Clinical management of severe acute respiratory infection when novel coronavirus (nCoV) infection is suspected: interim guidance 2 1. In: Triage: early recognition of patients with SARI associated with nCoV infection (2020). Available from: https://apps.who.int/iris/bitstream/handle/10665/331446/WHO-2019-nCoV-clinical-2020.4-eng.pdf?sequence=1&isAllowed=y (Accessed March 13, 2020).
27. Ferrari, BL, Carlos, , , Ferreira, G, Menezes, M, de Marchi, P, Canedo, J, et al. Determinants of COVID-19 mortality in patients with cancer from a community oncology practice in Brazil. JCO Glob Oncol (2021) 7:46–55. doi:10.1200/GO.20.00444
28. Meza Riquelme, MJS, Condori Pereyra, AR, and Encalada Carbajal, DA. Análisis de políticas públicas en el Perú ante la crisis derivada de la COVID-19. Semestre Económico (2020) 23(55):113–38. doi:10.22395/seec.v23n55a5
29. Zorzi, M, Guzzinati, S, Avossa, F, Fedeli, U, Calcinotto, A, and Rugge, M. SARS-CoV-2 infection in cancer patients: a population-based study. Front Oncol (2021) 11:730131. doi:10.3389/fonc.2021.730131
30. Kuderer, NM, Choueiri, TK, Shah, DP, Shyr, Y, Rubinstein, SM, Rivera, DR, et al. Clinical impact of COVID-19 on patients with cancer (CCC19): a cohort study. The Lancet (2020) 395(10241):1907–18. doi:10.1016/S0140-6736(20)31187-9
31. Borno, HT, Kim, MO, Hong, JC, Yousefi, S, Lin, A, Tolstykh, I, et al. COVID-19 outcomes among patients with cancer: observations from the university of California cancer consortium COVID-19 project outcomes registry. Oncologist (2022) 27(5):398–406. doi:10.1093/oncolo/oyac038
32. Pinato, DJ, Scotti, L, Gennari, A, Colomba-Blameble, E, Dolly, S, Loizidou, A, et al. Determinants of enhanced vulnerability to coronavirus disease 2019 in UK patients with cancer: a European study. Eur J Cancer (2021) 150:190–202. doi:10.1016/j.ejca.2021.03.035
33. Schreiber, RD, Old, LJ, and Smyth, MJ. Cancer immunoediting: integrating immunity’s roles in cancer suppression and promotion. Science (2011) 331(6024):1565–70. doi:10.1126/science.1203486
34. Khorana, AA, Mackman, N, Falanga, A, Pabinger, I, Noble, S, Ageno, W, et al. Cancer-associated venous thromboembolism. Nat Rev Dis Primers (2022) 8(1):11–8. doi:10.1038/s41572-022-00336-y
35. Connors, JM, and Levy, JH. Thromboinflammation and the hypercoagulability of COVID-19. J Thromb Haemost (2020) 18:1559–61. doi:10.1111/jth.14849
36. Mangalmurti, N, and Hunter, CA. Cytokine storms understanding COVID-19. Immunity (2020) 53(1):19–25. doi:10.1016/j.immuni.2020.06.017
37. Owusuaa, C, Dijkland, SA, Nieboer, D, van der Heide, A, and van der Rijt, CCD. Predictors of mortality in patients with advanced cancer—a systematic review and meta-analysis. Cancers (2022) 14:328. doi:10.3390/cancers14020328
38. Ullgren, H, Camuto, A, Rosas, S, Pahnke, S, Ginman, B, Enblad, G, et al. Clinical characteristics and factors associated with COVID-19-related death and morbidity among hospitalized patients with cancer: a Swedish cohort study. Acta Oncologica (2021) 60(11):1459–65. doi:10.1080/0284186X.2021.1958005
39. Liu, Y, Lu, H, Wang, W, Liu, Q, and Zhu, C. Clinical risk factors for mortality in patients with cancer and COVID-19: a systematic review and meta-analysis of recent observational studies. Expert Rev Anticancer Ther (2021) 21(1):107–19. doi:10.1080/14737140.2021.1837628
40. Fattore, GL, Olivos, NSA, Olalla, JEC, Gomez, L, Marucco, AF, and Mena, MPR. Mortality in patients with cancer and SARS-CoV-2 infection: results from the argentinean network of hospital-based cancer registries. Cancer Epidemiol (2022) 79:102200. doi:10.1016/j.canep.2022.102200
41. Jergović, M, Coplen, CP, Uhrlaub, JL, and Nikolich-Žugich, J. Immune response to COVID-19 in older adults. J Heart Lung Transplant (2021) 40(10):1082–9. doi:10.1016/j.healun.2021.04.017
42. Kernan, KF, and Carcillo, JA. Hyperferritinemia and inflammation. Int Immunol (2017) 29(9):401–9. doi:10.1093/intimm/dxx031
43. Matzner, Y, Konijn, AM, and Hershko, C. Serum ferritin in hematologic malignancies. Am J Hematol (1980) 9:13–22. doi:10.1002/ajh.2830090103
44. Aulbert, E, and Steffens, O. Ferritin im serum-ein «tumormarker» bei malignen lymphomen? Oncology Research and Treatment (1990) 13(2):102–8. doi:10.1159/000216735
45. Qeadan, F, Tingey, B, Gu, LY, Packard, AH, Erdei, E, and Saeed, AI. Prognostic values of serum ferritin and d-dimer trajectory in patients with covid-19. Viruses (2021) 13(3):419. doi:10.3390/v13030419
46. Depalma, RG, Hayes, VW, and O’Leary, TJ. Optimal serum ferritin level range: iron status measure and inflammatory biomarker. Metallomics (2021) 13(6):mfab030. doi:10.1093/mtomcs/mfab030
47. Bakker, J, Gris, P, Coffernils, M, Kahn, RJ, and Vincent, JL. Serial blood lactate levels can predict the development of multiple organ failure following septic shock. Am J Surg (1996) 171:221–6. doi:10.1016/S0002-9610(97)89552-9
48. Bakker, J, and Vincent, JL. The oxygen supply dependency phenomenon is associated with increased blood lactate levels. J Crit Care (1991) 6:152–9. doi:10.1016/0883-9441(91)90006-f
49. Nazer, LH, Rimawi, D, and Hawari, FI. Evaluating the predictive value of lactate in patients with cancer having septic shock. J Intensive Care Med (2020) 35(8):789–96. doi:10.1177/0885066618788821
50. Bagshaw, SM, and Gibney, RTN. Conventional markers of kidney function. Crit Care Med (2008) 36:S152–8. doi:10.1097/CCM.0b013e318168c613
51. Stevens, LA, and Levey, AS. Measurement of kidney function. Med Clin North America (2005) 89:457–73. doi:10.1016/j.mcna.2004.11.009
52. Peleg, Y, Kudose, S, D’Agati, V, Siddall, E, Ahmad, S, Nickolas, T, et al. Acute kidney injury due to collapsing glomerulopathy following COVID-19 infection. Kidney Int Rep (2020) 5(6):940–5. doi:10.1016/j.ekir.2020.04.017
53. Chueh, TI, Zheng, CM, Hou, YC, and Lu, KC. Novel evidence of acute kidney injury in COVID-19. J Clin Med (2020) 9(11):3547. doi:10.3390/jcm9113547
54. Nath, SS, Yadav, NU, Derkach, A, Perez-Johnston, R, Tachiki, L, Maguire, K, et al. Outcomes of patients with COVID-19 from a specialized cancer care emergency room. Cancer Invest (2022) 40(1):17–25. doi:10.1080/07357907.2021.1985134
55. Williams Donaldson, V. A clinical study of visualization on depressed white blood cell count in medical patient. Appl Psychophysiology Biofeedback (2000) 25(2):117–28. doi:10.1023/a:1009518925859
56. Kolaczkowska, E, and Kubes, P. Neutrophil recruitment and function in health and inflammation. Nat Rev Immunol (2013) 13:159–75. doi:10.1038/nri3399
57. Balkwill, F, Charles, KA, and Mantovani, A. Smoldering and polarized inflammation in the initiation and promotion of malignant disease. Cancer Cell (2005) 7:211–7. doi:10.1016/j.ccr.2005.02.013
58. Coussens, LM, and Werb, Z. Inflammation and cancer. Nature (2002) 420(6917):860–867.doi:10.1038/nature01322
59. Mantovani, A, Allavena, P, Sica, A, and Balkwill, F. Cancer-related inflammation. Nature (2008) 454:436–44. doi:10.1038/nature07205
60. Shitara, K, Matsuo, K, Oze, I, Mizota, A, Kondo, C, Nomura, M, et al. Meta-analysis of neutropenia or leukopenia as a prognostic factor in patients with malignant disease undergoing chemotherapy. Cancer Chemother Pharmacol (2011) 68(2):301–7. doi:10.1007/s00280-010-1487-6
61. Lowenthal, RM, and Eaton, K. Toxicity of chemotherapy. Hematology/Oncology Clinics (1996) 10(4):967–90. doi:10.1016/s0889-8588(05)70378-6
62. Kvolik, S, Jukic, M, Matijevic, M, Marjanovic, K, and Glavas-Obrovac, L. An overview of coagulation disorders in cancer patients. Surg Oncol (2010) 19:e33–46. doi:10.1016/j.suronc.2009.03.008
63. de Cicco, M. The prothrombotic state in cancer: pathogenic mechanisms. Crit Rev Oncology/Hematology (2004) 50:187–96. doi:10.1016/j.critrevonc.2003.10.003
64. Francone, M, Iafrate, F, Masci, GM, Coco, S, Cilia, F, Manganaro, L, et al. Chest CT score in COVID-19 patients: correlation with disease severity and short-term prognosis. Eur Radiol (2020) 30(12):6808–17. doi:10.1007/s00330-020-07033-y
65. Angeli, E, Dalto, S, Marchese, S, Setti, L, Bonacina, M, Galli, F, et al. Prognostic value of CT integrated with clinical and laboratory data during the first peak of the COVID-19 pandemic in northern Italy: a nomogram to predict unfavorable outcome. Eur J Radiol (2021) 137:109612. doi:10.1016/j.ejrad.2021.109612
66. Bayrak, V, Şentürk Durukan, N, Demirer Aydemir, F, Ergan, B, Gezer, NS, Eren Kutsoylu, OÖ, et al. Risk factors associated with mortality in ıntensive care COVID-19 patients: the importance of chest CT score and intubation timing as risk factors. Turk J Med Sci (2021) 51(4):1665–74. doi:10.3906/sag-2101-89
67. Li, K, Li, K, Chen, D, Chen, D, Chen, S, Chen, S, et al. Predictors of fatality including radiographic findings in adults with COVID-19. Respir Res (2020) 21(1):146. doi:10.1186/s12931-020-01411-2
Keywords: SARS-CoV-2, imaging study, biomarkers, death prognosis, active cancer
Citation: Villanueva-Cotrina F, Velarde J, Rodriguez R, Bonilla A, Laura M, Saavedra T, Portillo-Alvarez D, Bustamante Y, Fernandez C and Galvez-Nino M (2023) Active cancer as the main predictor of mortality for COVID-19 in oncology patients in a specialized center. Pathol. Oncol. Res. 29:1611236. doi: 10.3389/pore.2023.1611236
Received: 10 April 2023; Accepted: 11 August 2023;
Published: 07 September 2023.
Edited by:
Anna Sebestyén, Semmelweis University, HungaryCopyright © 2023 Villanueva-Cotrina, Velarde, Rodriguez, Bonilla, Laura, Saavedra, Portillo-Alvarez, Bustamante, Fernandez and Galvez-Nino. This is an open-access article distributed under the terms of the Creative Commons Attribution License (CC BY). The use, distribution or reproduction in other forums is permitted, provided the original author(s) and the copyright owner(s) are credited and that the original publication in this journal is cited, in accordance with accepted academic practice. No use, distribution or reproduction is permitted which does not comply with these terms.
*Correspondence: Freddy Villanueva-Cotrina, ZnJlZGR5dmlsbGFudWVAZ21haWwuY29t