- 1Department of Pharmacy, First Affiliated Hospital of Xinjiang Medical University, Urumqi, China
- 2Bioinformatics Research Lab, Center for Research Innovation and Development (CRID), Dhaka, Bangladesh
- 3School of Pharmacy, China Pharmaceutical University, Nanjing, China
- 4Institute of Food Science and Technology, Bangladesh Council of Scientific and Industrial Research (BCSIR), Dhaka, Bangladesh
Background: Previous studies revealed that colonic cancer-associated fibroblasts (CAFs) are associated with the modulation of the colon tumor microenvironment (TME). However, identification of key transcriptomes and their correlations with the survival prognosis, immunosuppression, tumor progression, and metastasis in colon cancer remains lacking.
Methods: We used the GSE46824, GSE70468, GSE17536, GSE35602, and the cancer genome atlas (TCGA) colon adenocarcinoma (COAD) datasets for this study. We identified the differentially expressed genes (DEGs), Kyoto Encyclopedia of Genes and Genomes (KEGG) pathways, hub genes, and survival-associated genes in colon cancer. Finally, we investigated the correlation of key genes with the survival prognosis, immunosuppression, and metastasis.
Results: We identified 246 common DEGs between the GSE46824 and GSE70468 datasets of colonic CAFs, which included 72 upregulated and 174 downregulated genes. The upregulated pathways are mainly involved with cancers and cellular signaling, and downregulated pathways are involved with immune regulation and cellular metabolism. The search tool for the retrieval of interacting genes (STRING)-based analysis identified 15 hub genes and 9 significant clusters in colonic CAFs. The upregulation of CTHRC1, PDGFC, PDLIM3, NTM, and SLC16A3 and downregulation of FBN2 are correlated with a shorter survival time in colon cancer. The CTHRC1, PDGFC, PDLIM3, and NTM genes are positively correlated with the infiltration of tumor-associated macrophages (TAM), macrophages, M2 macrophages, the regulatory T cells (Tregs), T cell exhaustion, and myeloid-derived suppressor cells (MDSCs), indicating the immunosuppressive roles of these transcriptomes in colon cancer. Moreover, the CTHRC1, PDGFC, PDLIM3, NTM, and SLC16A3 genes are gradually increased from normal tissue to the tumor and tumor to the metastatic tumor, and FBN2 showed the reverse pattern. Furthermore, the CTHRC1, FBN2, PDGFC, PDLIM3, and NTM genes are positively correlated with the metastatic scores in colon cancer. Then, we revealed that the expression value of CTHRC1, FBN2, PDGFC, PDLIM3, NTM, and SLC16A3 showed the diagnostic efficacy in colonic CAFs. Finally, the expression level of CTHRC1, PDGFC, and NTM genes are consistently altered in colon tumor stroma as well as in the higher CAFs-group of TCGA COAD patients.
Conclusion: The identified colonic CAFs-derived key genes are positively correlated with survival prognosis, immunosuppression, tumor progression, and metastasis.
Background
Cancer is a complex ecosystem comprising several cellular components including stromal cancer-associated fibroblasts (CAFs) [1]. CAFs are dominant components of the tumor microenvironment (TME) to control the colon cancer cells [2]. In the TME, CAFs promote the development, invasion, progression, and metastasis of colon cancer cells [2]. The increasing number of CAFs in the TME is correlated with poor prognosis and recurrence of colorectal cancer (CRC) [3]. Colonic CAFs-derived transcriptomes are associated with tumor angiogenesis, metastasis, and prognosis of human colon cancer [4]. In adenomatous colorectal polyps and primary tumors, accumulation of CAFs is associated with inferior prognosis and recurrence of disease [5]. CAFs of colonic mucosa provide information on functional heterogeneity and are associated with the prognosis [6]. The CAFs-associated markers are increased at the invasive front of the tumor and it is critically correlated with the tumor-stroma ratio in CRC [7].
CAFs are predominantly associated with the secretion of several inflammatory mediators which included chemokines, cytokines, transcription factors, proteases, and growth factors, thereby promoting the immunosuppressive TME [8]. These immunosuppressive mediators strictly control the reactivity of tumor and stroma cell communication in the TME [9, 10]. CAFs are associated with the modulation of the immune system and it is correlated with immune evasion and poor immunotherapy responses [11]. Moreover, CAFs- derived gene expression signatures are associated with poor clinical outcomes in CRC [12, 13]. Hang Zheng et al. identified the CAFs-associated transcriptomes that influence the prognosis and therapeutic responses in CRC [14]. In CRC, the higher CAF infiltration scores predicted poor disease-free survival (DFS) outcomes [15]. CAFs- expressed transcription factors (TFs) are correlated with epithelial-to-mesenchymal transition (EMT) which ultimately controls the cellular stemness and fate [16]. Also, TFs are related to the infiltration of CAFs and the independent prognostic factors which ultimately associated with cancer cell invasion, proliferation, and progression [17]. These studies provide the clue that the CAFs-derived transcriptomes are associated with cancer initiation, invasion, migration, and metastasis.
Herein, we identified the deregulated transcriptional signatures of human colonic CAFs. Then we analyzed the association of CAFs-derived DEGs with the enrichment of pathways. Moreover, we identified the key hub genes and significant clusters from the protein-protein interaction (PPI) network. Furthermore, we investigated the correlation of key genes with survival prognosis, immunosuppression, tumor progression, and metastasis.
Methods
Datasets
We searched the NCBI gene expression omnibus (GEO) database (https://www.ncbi.nlm.nih.gov/geo/) using the keywords “colon cancer fibroblast,” “cancer-associated fibroblast,” “CAFs” “stroma cells,” “colon tumor stroma cells” and “tumor stroma,” and identified two cancer-associated fibroblasts gene expression datasets GSE46824 (n = 34) [18] and GSE70468 (n = 14) [19] of colonic CAFs. In GSE70468, we selected the 7 CAFs samples and 7 colon normal fibroblasts samples and removed the other samples from this dataset. Besides, we downloaded the TCGA COAD cohort and normalized the data into log2-base transformation (https://gdc-portal.nci.nih.gov/) [20]. For analyzing the survival differences in the TCGA COAD dataset (https://gdc-portal.nci.nih.gov/), we used the gene expression profiling interactive analysis (GEPIA) [21] tool (http://gepia2.cancer-pku.cn/#index). Furthermore, we used PrognoScan-based [22] colon cancer data, the GSE17536 (n = 177) [23], for validating the survival-associated genes found in TCGA data. Finally, we used the colon tumor-stromal dataset GSE35602 [24] for verifying the expression levels of key genes. The summary of the GEO datasets is presented in Table 1.
Identification of Differentially Expressed Genes
The differential expression was screened by using GEO2R (http://www.ncbi.nlm.nih.gov/geo/geo2r), which is an interactive web tool to identify the DEGs. GEO2R tool identified the significant DEGs by utilizing the GEOquery [25] and limma R packages [26] from the Bioconductor project (http://www.bioconductor.org/). The R package “limma” was employed for identifying the significant DEGs between CAFs and normal colonic fibroblasts (NCFs) [26]. We identified the DEGs with a threshold absolute value of fold change (LogFC) > 0.585 and a p-value ≤0.05 [27, 28]. Finally, we identified the common DEGs between both datasets. We utilized the online tool “Calculate and draw custom Venn diagrams” (http://bioinformatics.psb.ugent.be/webtools/Venn/) for identifying common DEGs in both datasets.
Gene-Set Enrichment Analysis
We performed a gene-set enrichment analysis of the DEGs by using the gene-set enrichment analysis (GSEA) [29]. We inputted all significant commonly found upregulated and downregulated DEGs into the GSEA tool for identifying deregulated pathways. In the GSEA, we selected the KEGG pathways panel to identify the significant pathways. The KEGG [30] pathways significantly associated with the upregulated DEGs and the downregulated DEGs were identified, respectively. The p-value <0.05 was considered significant when separating the pathways.
Identifying Hub Genes and Modular Analysis From Protein-Protein Interaction Network of Differentially Expressed Genes
To better know the relationship among these identified DEGs, the PPI network was established using the STRING-based analysis [31]. To identify the rank of hub genes, we used Cytoscape plug-in tool cytoHubba [32]. Hub genes were identified based on the degree of interactions with neighbour genes. We set the minimum required interaction score as 0.40 for identifying the PPI of DEGs. Hub genes were defined as a gene that was connected to a minimum of 10 other DEGs in the PPI. We visualize the PPI networks by utilizing the Cytoscape 3.6.1 software [33]. A Cytoscape plug-in molecular complex detection (MCODE) was employed to detect the modules from the PPI network [34]. This is a computational method for searching molecular complexes in large protein interaction networks. We identified the significant modules based on the MCODE score and node number. The threshold of the MCODE was node score cut-off: 0.2, haircut: true, k-core: 2, and maximum depth from Seed: 100.
Survival Analysis of Differentially Expressed Genes by Using the Gene Expression Profiling Interactive Analysis and PrognoScan
We compared the overall survival (OS) and the DFS of colon cancer patients. Kaplan-Meier survival curves were used to show the survival differences between the high expression group and low expression groups. The survival significance of all DEGs in the TCGA COAD cohort was analysed using GEPIA [21] databases. GEPIA is a web-based tool to deliver fast and customizable functionalities based on TCGA data. Furthermore, we used PrognoScan-based [22] GSE17536 (n = 177) [23] for validating the survival-associated genes found in the TCGA COAD dataset. PrognoScan is a database for meta-analysis of the prognostic value of genes. Cox regression p-value <0.05 was considered as significant when comparing the survival between the two groups.
The ESTIMATE Algorithm for Quantifying Immune Score and Stromal Score
ESTIMATE is an algorithmic tool based on the R package for predicting tumor purity, immune score (predicting the infiltrations of immune cells), and stromal score (predicting the infiltrations of stromal cells) which uses the gene expression profiles of 141 immune genes and 141 stromal genes [35]. The presence of infiltrated immune cells and stromal cells in tumor tissues were calculated using related gene expression matrix data, represented by immune score and stromal score, respectively [35]. Then we calculated the correlations of key genes with immune scores and stromal scores. The threshold value of correlation is R > 0.30, and p-value is not less than 0.001 (Spearman’s correlation test).
Single-Sample Gene-Set Enrichment Analysis and Correlation of Immune Signatures With Survival-Associated Genes
One of the extension packages of GSEA, single-sample gene-set enrichment analysis (ssGSEA) was used to identify the enrichment scores of immune cells for each pairing of a sample and gene set in the tumor samples [36]. We collected the marker gene set for immune signatures and utilized each gene set to quantify the ssGSEA scores of specific immune signatures [37–40]. We identified the ssGSEA scores of CD8+ T cells, CD4+ regulatory T cells, NK cells, TAM, macrophages, M2 macrophages, Tregs, T cell exhaustion, myeloid-derived suppressor cells (MDSCs), and CAFs. Moreover, we used the metastasis-promoting gene set for identifying the ssGSEA scores of metastasis-promoting genes [40, 41]. All of the marker genes are displayed in Supplementary Table S1. Then we investigated Spearman’s correlation between the ssGSEA scores and specific survival-associated genes. The absolute value of this correlation was greater than 0.30 with a p-value less than 0.001.
Diagnostic Efficacy Evaluation and Expression Validation for Survival-Associated Key Genes
To assess diagnostic values of the survival-associated genes, the receiver operating characteristic (ROC) curve was plotted and the area under the ROC curve (AUC) was calculated using the “pROC” R package [42] to evaluate the capability of distinguishing cancer-associated fibroblasts and normal tissues. The “pROC” R package can visualize, smooth, and compare ROC curves. The AUC can be compared with statistical tests based on U-statistics or bootstrap. A greater AUC value of individual genes indicated the differences between tumor and normal samples, and the key gene of AUC > 0.5 in the CAFs datasets was defined as a diagnostic efficiency of the gene [43]. To verify the survival-associated genes in a colorectal cancer stromal tissue, we selected GSE35602 [24] to distinguish the expression levels of key genes between non-tumor and tumor samples. We identified the ssGSEA scores (prediction of the content) of CAFs by using the marker genes (Supplementary Table S1) of CAFs. Then, we divided the TCGA COAD samples into the high-CAFs group versus the low-CAFs group based on the median value of ssGSEA scores of CAFs.
Statistical Analysis
We used the R software version 4.0.1 for all statistical analysis. In the Log-rank test, p < 0.05 was considered statistically significant for survival analysis. To investigate the correlation of survival-associated genes, Spearman’s correlation between the ssGSEA scores and specific survival-associated genes was performed (p < 0.001). We used Welch’s t-test for identifying the significance of specific genes between the two groups of samples (p ≤ 0.05). We utilized the R package ggplot2 for the graphical presentation of the Heatmap and correlation graph.
Results
Identification of Differentially Expressed Genes in Colonic Cancer-Associated Fibroblasts
We identified 246 commonly differentially expressed genes (DEGs) between the GSE46827 and GSE70468 datasets. The principal component analysis (PCA) plot of samples is shown in Figures 1A,B and the commonly upregulated and downregulated genes in CAFs and normal colonic fibroblasts (NCFs) are shown in Figures 1C,D respectively. We found 72 upregulated (Figure 1C; Supplementary Table S2) and 174 downregulated (Figure 1D; Supplementary Table S3) genes in the CAFs when compared with NCFs. CDH13, DSP, EFNB2, IL7R, KIAA1217, KRT19, NTM, PDLIM3, SRGN, SYNPO2L, TGFB2, and TNFSF4 were the most significantly upregulated genes in colon CAFs (Supplementary Table S2), while A2M, AOC3, ADH1B, ASPA, CHRDL1, COL14A1, CYP24A1, FBN2, FBLN1, GREM2, IL33, NOVA1, PRKCH, SEPP1, and SMOC2 are the most downregulated genes over the selected datasets (Supplementary Table S3).
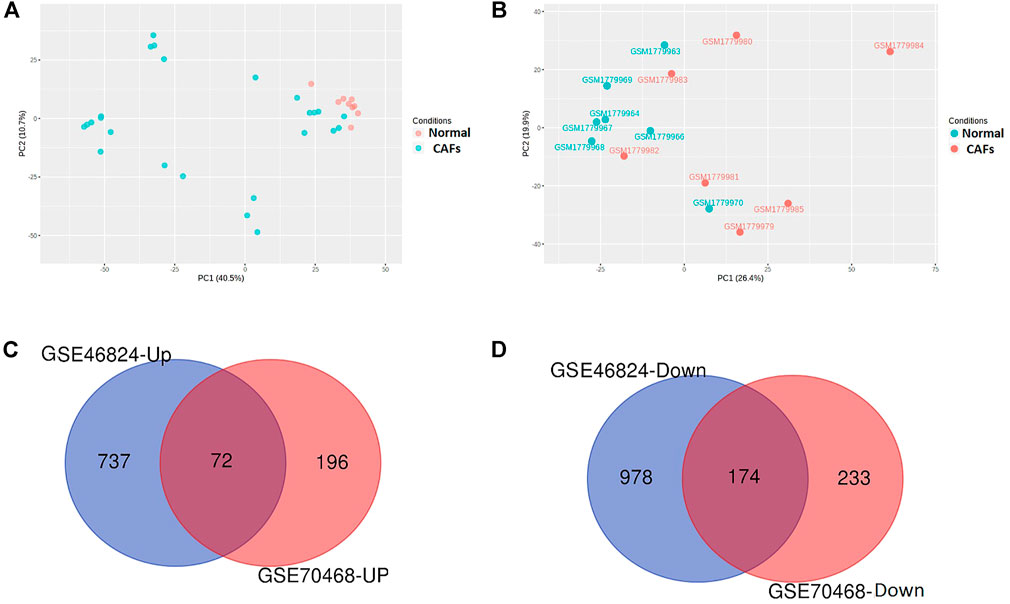
FIGURE 1. Identification of DEGs in the colonic CAFs. (A) The PCA analysis of samples in the GSE46824 dataset comprehensively summarizes the data (B). The PCA analysis of samples in the GSE70468 dataset comprehensively summarizes the data. (C) Identifying commonly found 72 upregulated genes in both datasets. (D) Identifying commonly found 174 downregulated genes in both datasets. CAFs: cancer-associated colonic fibroblasts; Normal: normal colonic fibroblasts; GSE46824-Up: Upregulated gene in GSE46824; GSE46824-Down: Downregulated gene in GSE46824; GSE70468-Up: Upregulated gene in GSE70468, GSE70468-Down: Downregulated gene in GSE70468.
CAFs-Derived Transcriptomes Are Associated With the Enrichment of Kyoto Encyclopedia of Genes and Genomes Pathways
The enriched upregulated and downregulated biological pathways were identified by using the GSEA tool (Figures 2, 3). GSEA identified 28 KEGG pathways significantly associated with common upregulated 72 DEGs (Supplementary Table S4). The pathways are mainly involved with cancer or malignant tumor (colorectal cancer, pancreatic cancer, pathways in cancer, melanoma, chronic myeloid leukemia, small cell lung cancer, and prostate cancer) and cellular signaling and developments (regulation of actin cytoskeleton, TGF-beta signaling pathway, focal adhesion, p53 signaling pathway, adherens junction, gap junction, MAPK signaling pathway, cell cycle, cell adhesion molecules (CAMs), and Wnt signaling pathway) (Figure 2).
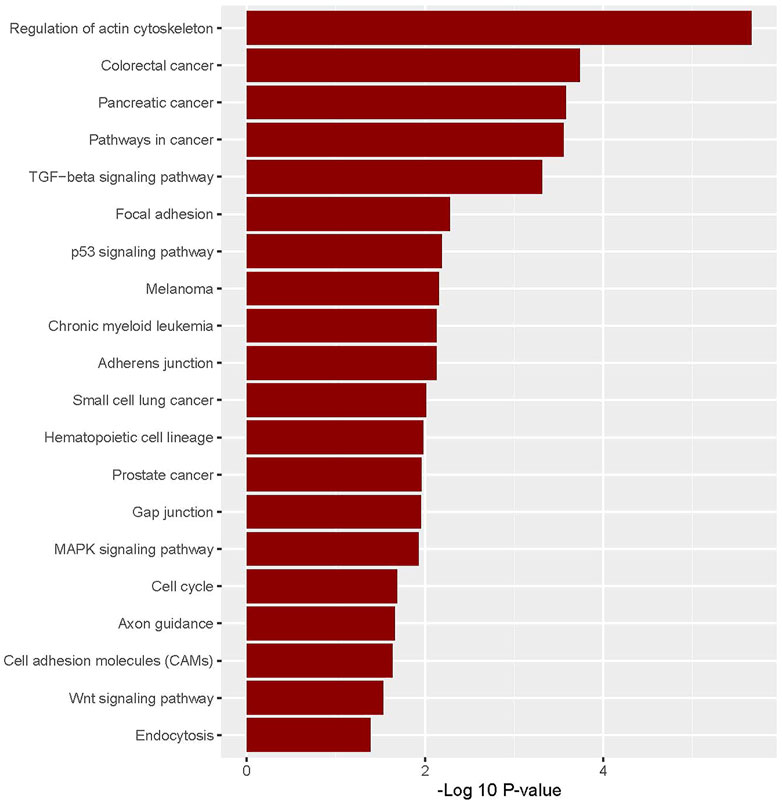
FIGURE 2. Significantly enriched KEGG pathways that are associated with upregulated colonic CAFs-derived DEGs.
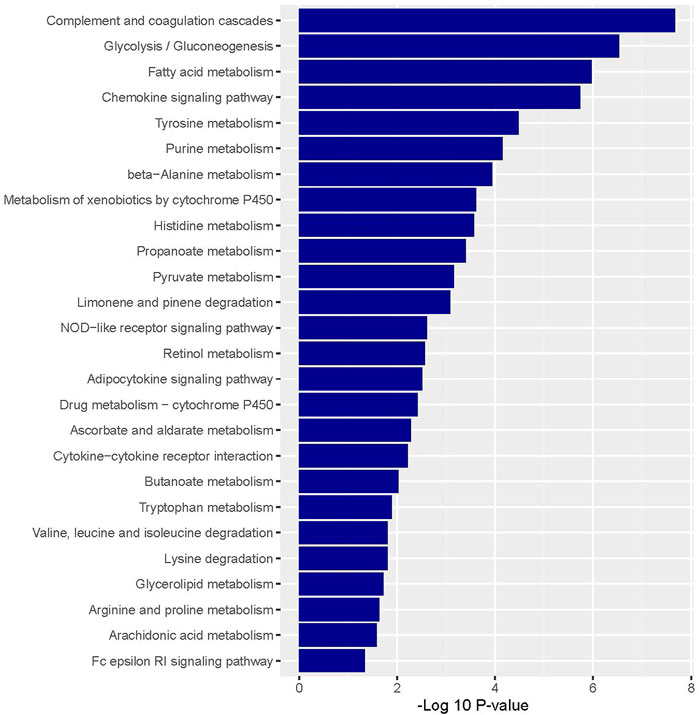
FIGURE 3. Significantly enriched KEGG pathways that are associated with downregulated colonic CAFs-derived DEGs.
Besides, we identified the 54 KEGG pathways that are significantly linked with commonly found downregulated 174 DEGs (Supplementary Table S5). The pathways are involved with immune regulation (such as complement and coagulation cascades, chemokine signaling pathway, and cytokine-cytokine receptor interaction) and cellular metabolism (glycolysis/gluconeogenesis, fatty acid metabolism, tyrosine metabolism, purine metabolism, beta-Alanine metabolism, metabolism of xenobiotics by cytochrome P450, histidine metabolism, propanoate metabolism, pyruvate metabolism, limonene and pinene degradation, retinol metabolism, drug metabolism—cytochrome P450, ascorbate and aldarate metabolism, butanoate metabolism, tryptophan metabolism, lysine degradation, valine, leucine and isoleucine degradation, glycerolipid metabolism, arginine and proline metabolism, and arachidonic acid metabolism) (Figure 3).
STRING-Based Protein-Protein Interaction Analysis Identified CAFs-Derived Hub Genes and Significant Modules in Colon Cancer
We investigated the PPI of all significant CAFs-derived DEGs. The PPI information of STRING is inputted into the Cytoscape for identifying and visualizing the hub genes and significant clusters. We identified 15 hub genes (minimum degree of interaction is 10 with other DEGs) which included 3 upregulated hub genes (PLAUR, RAC2, and TGFB2) and 12 downregulated hub genes (A2M, ADCY3, ADCY4, ADCY9, BIN1, CFD, CLU, CXCL12, LDLR, PPARG, PTK2B, and VTN) (Table 2).
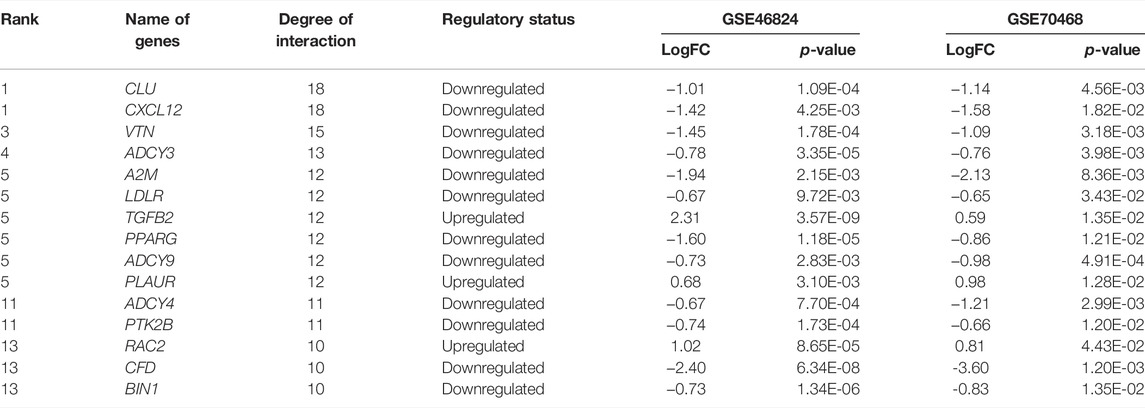
TABLE 2. Identification of the 15 hub genes and their degree of interaction with differential expression between the CAFs and NCFs in two datasets.
We found that 183 DEGs were involved in PPI (Supplementary Table S6). The other DEGs were not involved in the PPI network. We investigated the significant cluster-based analysis of this PPI network. The MCODE-based analysis identified 9 clusters from the original PPI networks. The description of MCODE-derived clusters is illustrated in Table 3. The top significant cluster 1 included 7 nodes and 21 edges (Table 3). We identified the significantly enriched KEGG pathways for the identified 9 clusters by using the GSEA. Interestingly, we found that seven clusters are associated with the enrichment of KEGG pathways (p < 0.05). Gene set of cluster 1 is associated with the enrichment of complement and coagulation cascades (p = 1.67e-7). Gene set of cluster 2 is mainly involved with immune regulation and cellular signaling (Table 3). In addition, the gene set of cluster 3 is mainly associated with the regulation of metabolism (Table 3). Also, Cluster 4, Cluster 6, Cluster 8, and Cluster 9 are associated with the enrichment of KEGG pathways (Table 3).
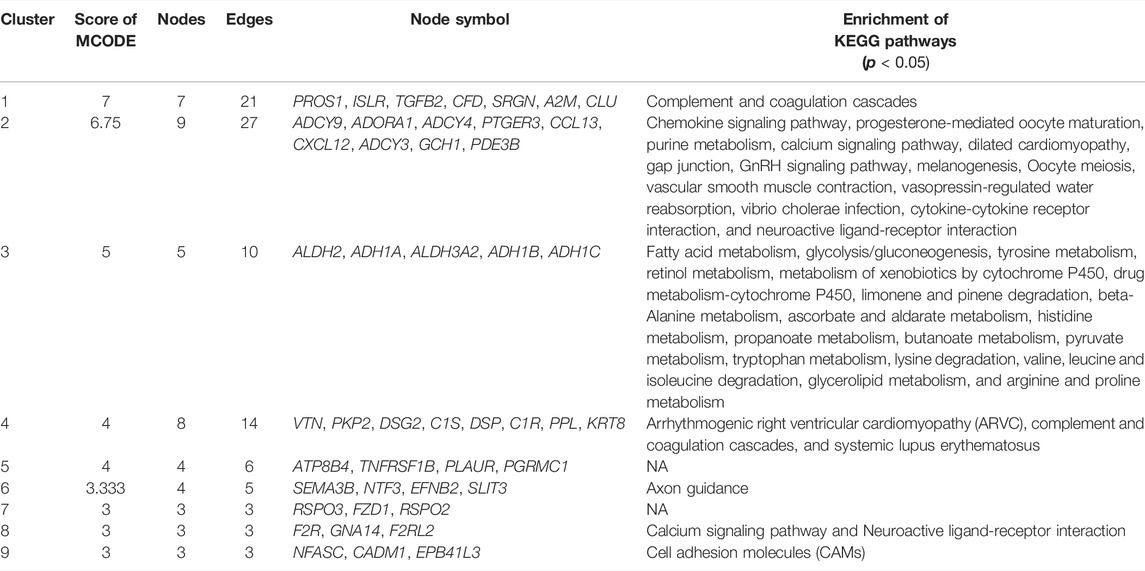
TABLE 3. MCODE identified significant 9 clusters from the PPI networks of DEGs and the GSEA-based analysis identified the enriched KEGG pathways (p < 0.05) for a specific gene set of individual clusters. NA: KEGG pathways not found for a specific cluster.
Cancer-Associated Fibroblasts Derived Transcriptomes Are Correlated With Survival Prognosis in Colon Cancer
We investigated the survival significance of the CAFs-derived all significant common DEGs (72 upregulated and 174 downregulated genes) in TCGA COAD data. Our analysis revealed that the upregulated group of CAFs-derived upregulated genes included CTHRC1, EMB, FOXS1, LYPD1, NTM, PDGFC, PDLIM3, SLC16A3, SYNPO2L, and TNFSF4 (Figure 4A) and the lower expression group of downregulated genes included ABCA5, FBN2, IMPA2, and TIMP4 (Figure 4B) are significantly correlated with shorter survival time of colon cancer patients (Figure 4). Moreover, we verified this result in a GEO dataset GSE17536 (n = 177) of colon cancer. We found that upregulated group of CTHRC1, NTM, PDGFC, PDLIM3, and SLC16A3 genes are consistently correlated with the shorter survival of colon cancer patients in GSE17536 (Figure 4C). In contrast, the downregulated group of FBN2 gene is consistently correlated with the shorter survival of colon cancer patients in GSE17536 (Figure 4C), indicating the tumor-suppressive role of FBN2 in colon cancer.
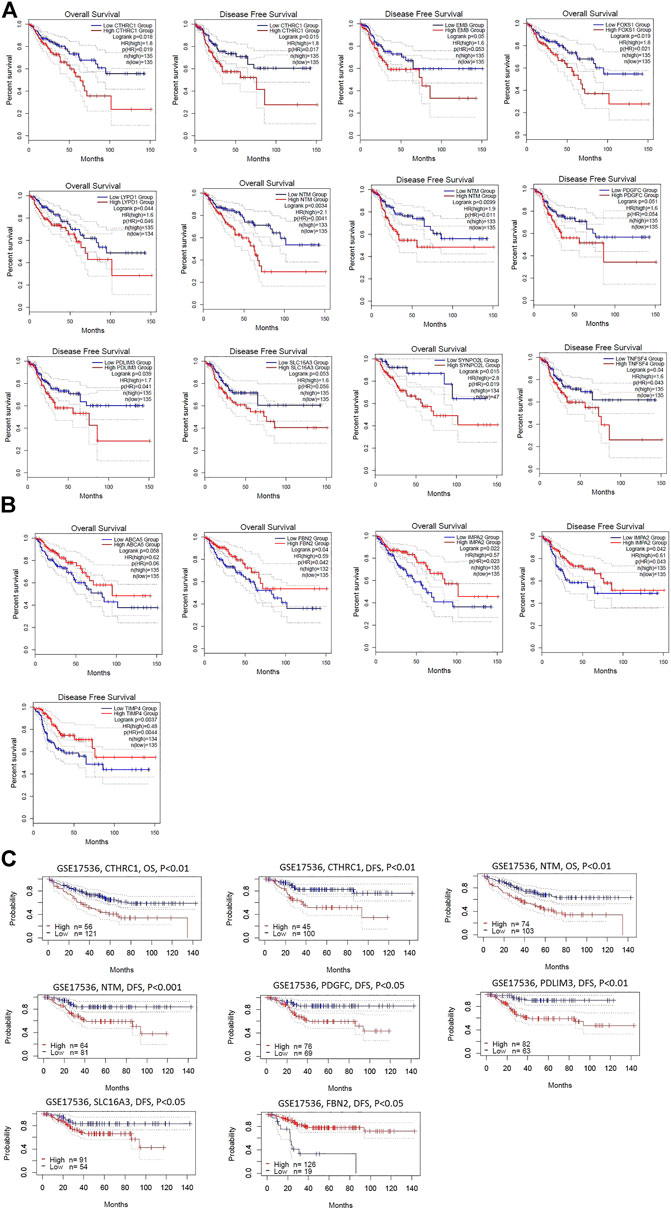
FIGURE 4. Identification of survival-associated DEGs in colonic CAFs. (A) The higher expression group of upregulated genes that included CTHRC1, EMB, FOXS1, LYPD1, NTM, PDGFC, PDLIM3, SLC16A3, SYNPO2L, and TNFSF4 are significantly correlated with shorter survival time in the TCGA COAD cohort. (B) The lower expression group of downregulated genes that included ABCA5, FBN2, IMPA2, and TIMP4 are significantly correlated with shorter survival time in the TCGA COAD cohort (C). The higher group of CTHRC1, NTM, PDGFC, PDLIM3, and SLC16A3 genes are consistently correlated with the shorter survival of colon cancer patients in GSE17536. In contrast, the lower group of FBN2 gene is consistently correlated with the shorter survival of colon cancer patients in GSE17536.
Association of Survival-Associated Genes With Immune Score and Stroma Score in Colon Cancer
Since the upregulated group of CTHRC1, NTM, PDGFC, PDLIM3, and SLC16A3 and the downregulated group of FBN2 genes are consistently correlated with the shorter survival time in TCGA and GEO datasets, we investigated the association of these genes with immune score and stromal scores in colon cancer. Interestingly, we found that the expression levels (log2 transformation) of CTHRC1, NTM, PDGFC, and PDLIM3 are moderately correlated with immune scores (Spearman’s correlation test, p < 0.001) (Figure 5A). In addition, the expression levels (log2 transformation) of CTHRC1, NTM, PDGFC, PDLIM3, and FBN2 are strongly correlated with the stromal score (Spearman’s correlation test, p < 0.001) (Figure 5B). In contrast, SLC16A3 is not correlated with immune scores and stromal scores in the TCGA-COAD cohort (R > 0.30 and p < 0.01). This indicated that the expression levels of CTHRC1, FBN2, NTM, PDGFC, and PDLIM3 genes are associated with the modulation of immune and stromal activity in the colon cancer tumor microenvironment.
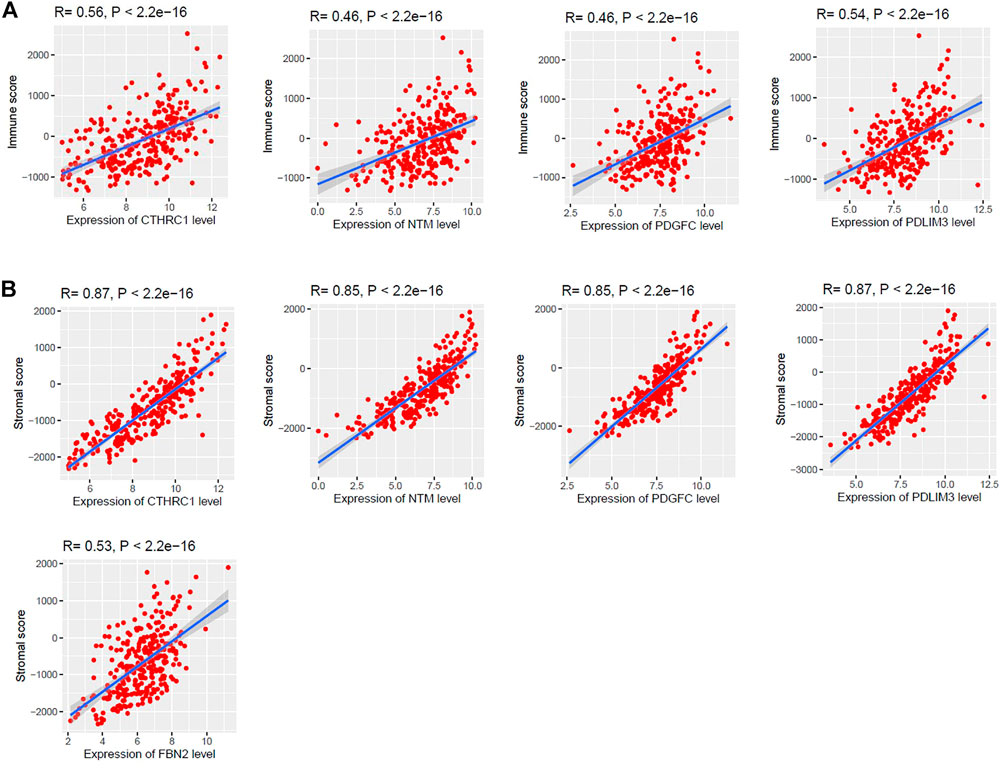
FIGURE 5. Association of CTHRC1, NTM, PDGFC, PDLIM3, and FBN2 expression levels with the regulation of tumor microenvironment (TME) in colon cancer. (A) The expression levels (log2 transformation) of CTHRC1, NTM, PDGFC, and PDLIM3 are moderately correlated with the immune score. (B) Strong positive correlation between CTHRC1, NTM, PDGFC, PDLIM3, and FBN2 with stromal scores. (R is Spearman’s correlation coefficient and P is the p-value). We used the TCGA COAD cohort (n = 287) to identify the correlation.
Cancer-Associated Fibroblasts Derived Survival-Associated Genes Are Associated With Immunosuppression in Colon Cancer
Since survival time of patients is correlated with immunological responses in human cancers [44], we investigated the correlation of six survival-associated genes (CTHRC1, NTM, PDGFC, PDLIM3, SLC16A3, and FBN2) with the several immune stimulatory and inhibitory signatures including CD8+ T cells, CD4+ regulatory T cells, NK cells, TAM, macrophages, M2 macrophages, Tregs, T cell exhaustion, and MDSCs. We found that the expression levels of upregulated CTHRC1, NTM, PDGFC, and PDLIM3 are positively correlated with ssGSEA scores of TAMs, macrophages, M2 macrophages, Tregs, T cell exhaustion, and MDSCs (Spearman’s correlation test, p < 0.001) (Figure 6). Besides, SLC16A3 is positively correlated with the infiltration of MDSCs (Spearman’s correlation test, p < 0.001) (Figure 6). In addition, the expression level of FBN2 is correlated with ssGSEA scores of TAMs, macrophages, M2 macrophages, Tregs, and MDSCs (Spearman’s correlation test, p < 0.001). Interestingly, the CD8+ T cells, CD4+ regulatory T cells, and NK cells are not significantly correlated with the expression levels of CTHRC1, NTM, PDGFC, PDLIM3, SLC16A3, and FBN2.
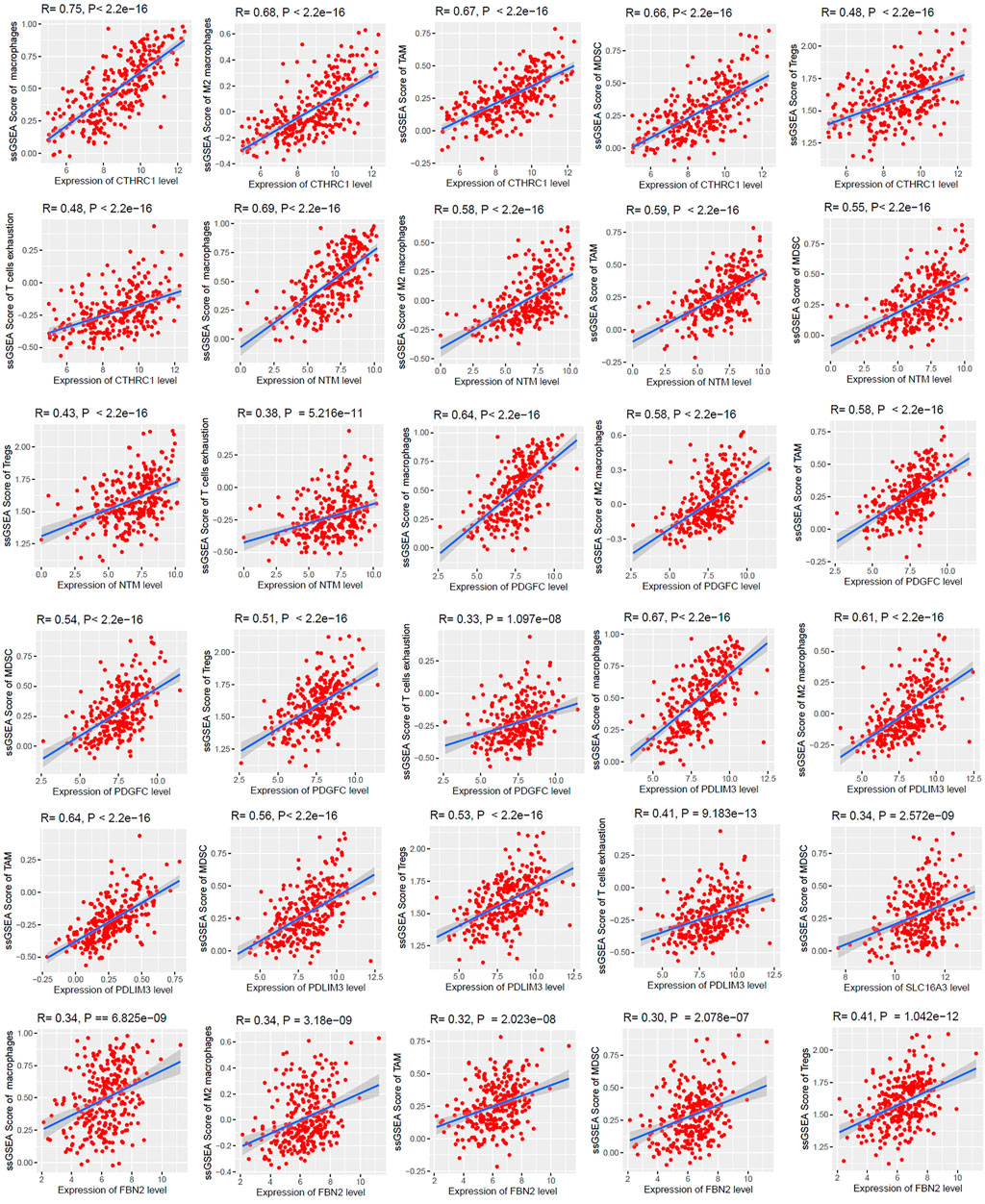
FIGURE 6. The expression levels of CTHRC1, NTM, PDGFC, PDLIM3, SLC16A3, and FBN2 are positively correlated with ssGSEA scores of immune signatures in colon cancer (Spearman’s correlation test, p < 0.001). We used the TCGA COAD cohort (n = 287) to identify the correlations.
Cancer-Associated Fibroblasts Derived Survival-Associated Genes Are Associated With Tumor Progression and Metastasis in Colon Cancer
We analysed the expression level differences of five shorter survival-associated genes (CTHRC1, NTM, PDGFC, PDLIM3, and SLC16A3) and one longer survival-associated gene (FBN2) among the normal fibroblast (n = 9), primary tumor fibroblast (n = 14), and metastatic tumor fibroblast (n = 11) samples of GSE46824 [18]. Interestingly, we found that CTHRC1, NTM, PDGFC, PDLIM3, and SLC16A3 are gradually upregulated from normal to a metastatic tumor, indicating the association of these genes in tumor progression (Welch’s t-test, p < 0.05) (Figure 7). Besides, the expression of FBN2 is gradually downregulated from normal to the metastatic tumor, indicating that the downregulation of this gene is associated with tumor progression (Welch’s t-test, p < 0.05) (Figure 7). Since the expression levels of CTHRC1, FBN2, NTM, PDGFC, PDLIM3, and SLC16A3 are significantly differentiated among the normal samples, primary tumor, and metastatic tumor, we anticipated that these genes are associated with metastasis in colon cancer.
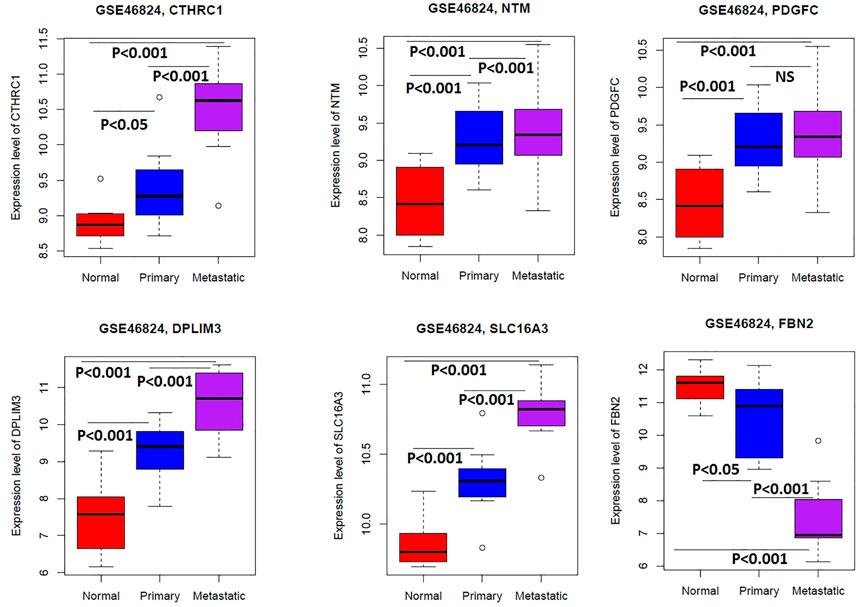
FIGURE 7. The expression levels of CTHRC1, NTM, PDGFC, PDLIM3, SLC16A3, and FBN2 are significantly differentiated among the normal samples, primary tumor, and metastatic tumor of colonic fibroblast (Welch’s t-test, p < 0.05). We used the GSE46824 to compare the expression level of genes in the normal samples, primary tumor, and metastatic tumor of colonic fibroblast.
To prove this hypothesis, we investigated the correlation of these genes with the metastasis-promoting gene set scores. We identified the ssGSEA scores of metastasis-promoting genes and identified the correlation with the expression levels of these six gene signatures (Spearman’s correlation test, p < 0.001). We found that the expression levels of CTHRC1, FBN2, NTM, PDGFC, and PDLIM3 are positively correlated with the ssGSEA scores of metastasis-promoting genes in the TCGA COAD cohort (Figure 8).
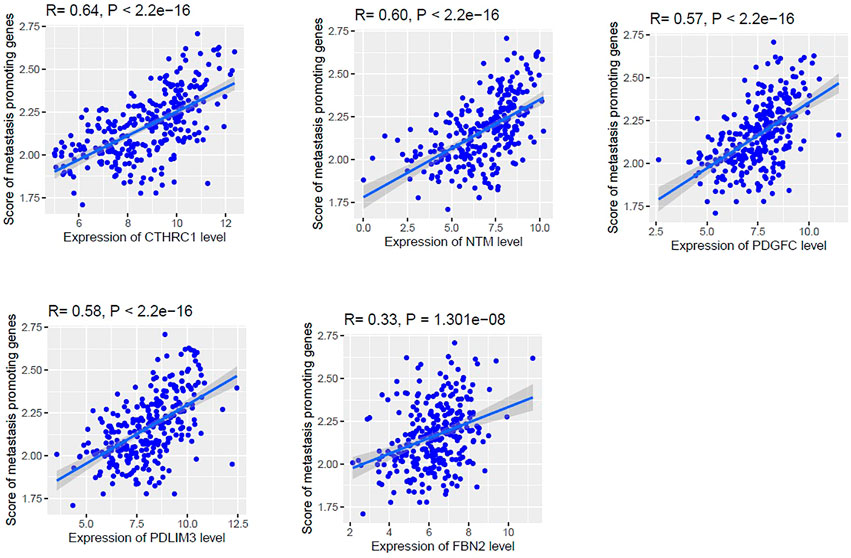
FIGURE 8. The expression levels of CTHRC1, NTM, PDGFC, PDLIM3, and FBN2 are positively correlated with the ssGSEA scores of metastasis-promoting genes in the TCGA COAD cohort (Spearman’s correlation test, p < 0.001).
Diagnostic Efficacy Evaluation in Fibroblast Dataset and Expression Validation of Key Survival-Associated Genes in Colon Tumor Stroma
We speculate that these six genes (CTHRC1, FBN2, NTM, PDGFC, PDLIM3, and SLC16A3) have diagnostic value in colonic CAFs. We used the GSE46824 dataset to validate our hypothesis, and the results showed that the ROC curve of the expression levels of these six genes (CTHRC1, FBN2, NTM, PDGFC, PDLIM3, and SLC16A3) showed excellent diagnostic value for colonic CAFs and normal colonic fibroblast (Figure 9A). Since CAFs are major regulatory components of tumor stroma [45], we validated the expression levels of survival-associated key genes between colon tumor stroma and normal colon stroma. Interestingly, we found that three upregulated survival-associated genes were also upregulated in colon tumor stroma versus normal colon stroma (p < 0.05) (Figure 9B). In addition, downregulated FBN2 is consistently downregulated in colon tumor stroma (Figure 9B). Moreover, we divided the TCGA COAD samples into the higher-CFAs group versus the lower-CAFs group to identify the expression level of CTHRC1, FBN2, NTM, PDGFC, and PDLIM3. Interestingly, we revealed that the expression of CTHRC1, FBN2, NTM, PDGFC, and PDLIM3 was highly expressed in the higher-CAFs group (Figure 9C). However, SLC16A3 is not significantly altered between the higher-CFAs group versus the lower-CAFs group. It indicates that the aberrant expression of CAFs-derived CTHRC1, NTM, and PDGFC is critically involved in the pathogenesis of CRC.
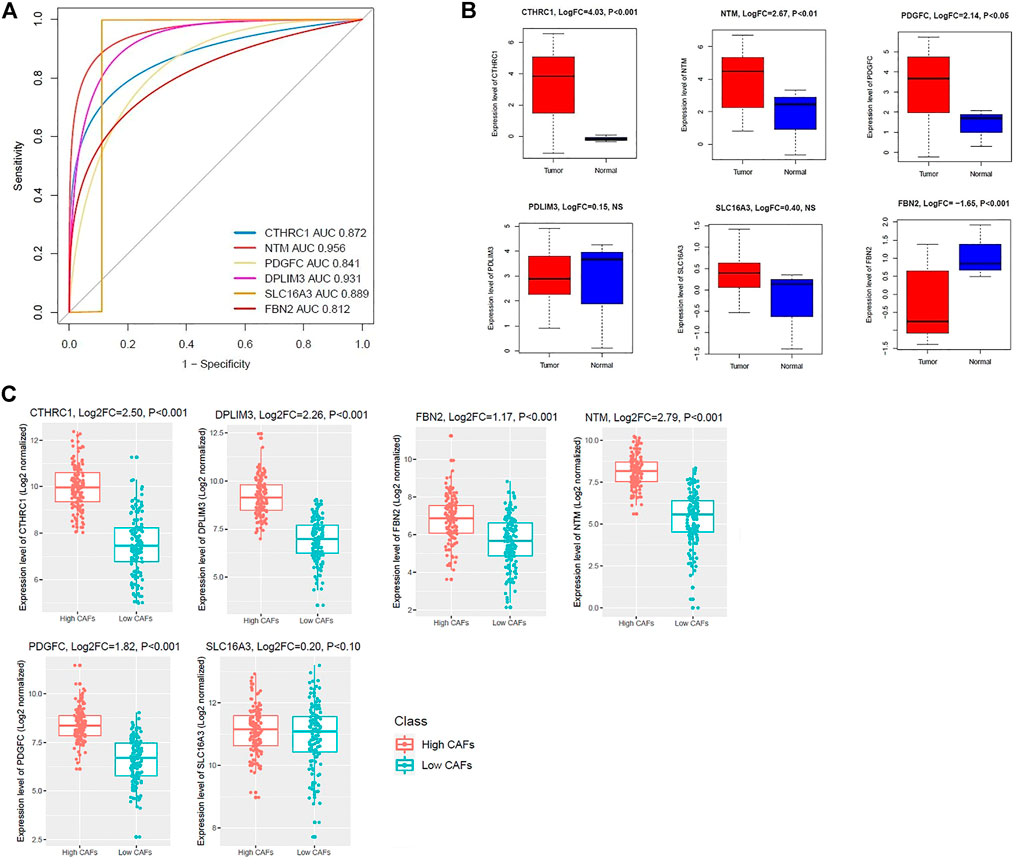
FIGURE 9. Evaluation of diagnostic efficacy and expression validation of key survival-associated genes. (A) The receiver operating characteristic (ROC) curve of survival-associated genes in colonic CAFs and normal fibroblast (GSE46824 dataset). (B) Expression validation of shorter survival-associated genes in colon tumor-stromal GSE35602 dataset. NS: Non-significant. (C) The shorter survival-associated genes are consistently deregulated in the high-CAFs group versus the low-CAFs group in the TCGA COAD dataset.
Discussion
Since CAFs are associated with tumor initiation, invasion, migration, metastasis, and inhibiting immunotherapy response [8–11], identifying key transcriptomes that are correlated with cellular signaling, survival prognosis, immunosuppression, tumor progression, and metastasis should be elucidated. To do this, we identified colonic CAFs-derived transcriptomes which included 72 commonly upregulated (Supplementary Table S2) and 174 commonly downregulated (Supplementary Table S3) DEGs. We used the two datasets, including GSE46824 and GSE70468. The GSE46824 was derived from the patient-derived colon normal fibroblasts and laser micro-dissected CAFs from the surgical colon cancer specimen. On the other hand, the GSE70468 was derived from the primary cultures of patient-derived colon normal fibroblasts (NFs) and cancer-associated fibroblasts (CAFs). We selected the commonly deregulated transcriptomes from both of the datasets (Figures 1C, D), indicating that these transcriptomes are associated with CAFs development in colon cancer tissues, and also involved with the development of CAFs in culture medium. Previous studies showed that CAFs-derived transcriptomes are associated with colon cancer growth, development, and progression [46–50]. For example, the TNFSF4 gene is associated with epigenetic regulation in colon cancer [49]. Xu et al. reported that SRGN can stimulate the metastasis of CRC as a key downstream target of HIF1-A [48]. TGFB2 is frequently mutated in colon cancer and associated with carcinogenesis [50]. The reduced level of SEPP1 is correlated with M2 macrophage polarization and its downregulation is linked with stemness and proliferation of cells [51]. AOC3, another downregulated gene in CAFs, is associated with the enrichment of pathways in colon cancer [52]. Altogether, it indicates that the CAFs derived deregulated transcriptomes are associated with the prognosis of colon cancer.
Then, we investigated the deregulated pathways which are associated with CAFs-derived DEGs. We found that the upregulated DEGs are associated with the enrichment of KEGG pathways that are mainly involved with cancer and cellular signaling and development (Supplementary Table S4). In contrast, CAFs-derived downregulated DEGs are associated with the enrichment of immune regulation and metabolism-associated pathways (Supplementary Table S5). Uddin et al. also found that many of these pathways including pathways in cancer, small cell lung cancer, ECM-receptor interaction, focal adhesion, TGF-beta signaling pathway, and cell adhesion molecules (CAMs) are enriched in colon tumor stroma [53, 54]. It indicates that the CAFs-derived transcriptomes are associated with the stimulation of cancerous and depression of immunological and metabolic pathways in colon cancer.
Since CAFs-derived transcriptomes [4] are crucially related to cancer pathogenesis and cancer therapy [55, 56], we identified key hub genes and survival-associated genes for finding their relationship with survival prognosis, immunosuppression, and metastasis. The PPI-based analysis identified key hub transcriptomes in colonic CAFs. Previous studies consistently found the relevance of these key genes with the pathogenesis of colorectal cancer. For example, the dysregulated FOXM1-PLAUR signaling axis is significantly associated with the progression and metastasis of human colon cancer [57]. In the Rac2−/− mice, a marked reduction in regional colonic lymph node metastasis was found, suggesting a substantial role for Rac2 in controlling spontaneous lymph node metastasis [58]. TGFB2, one of the major members of TGF-β signaling, is critically associated with the progression and susceptibility of colorectal cancer [59]. P Mazzarelli et al. reported that the serum level of CLU could represent a diagnostic marker for colon cancer screening [60]. Also, we identified 9 clusters that are associated with the enrichment of KEGG pathways (Table 3). The complement components are associated with the regulation and remodeling of the tumor microenvironment [61]. The chemokines are critically promoting the invasion and metastasis of colorectal cancer [62]. Altogether, PPI-based identification of key hub genes and clusters are involved in the regulation of colon cancer.
The expression level of survival-associated genes is significantly correlated with the shorter survival time of colon cancer patients (Figure 4). The elevated expression of CTHRC1 correlated with poor prognosis in patients with CRC [63]. It was reported that the overexpression of SLC16A3 is correlated with prognosis in pancreatic cancer [64]. Collectively, these results indicate that the CAFs-derived genes are associated with the poor prognosis of CRC patients. We selected these survival-associated genes (upregulated CTHRC1, NTM, PDGFC, PDLIM3, and SLC16A3, and downregulated FBN2) for finding the correlations (Spearman’s correlation test, p < 0.001) with various immune signatures (Figures 5, 6). Since TAM, macrophages, M2 macrophages, Tregs, T cell exhaustion, and MDSCs are immune suppressive components of TME [45,65,66], the positive correlation of these immune signatures with survival-associated genes indicates the immunosuppressive roles of these genes in the colon TME.
Furthermore, we investigated the expression level of CTHRC1, FBN2, NTM, PDGFC, PDLIM3, and SLC16A3 among the normal, primary tumor, and metastatic tumors in a colonic fibroblast dataset and revealed that these genes are gradually deregulated among the three groups, indicating their roles in tumor progression (Welch’s t-test, p < 0.05) (Figure 7). Finally, we investigated the correlation of CTHRC1, FBN2, NTM, PDGFC, PDLIM3, and SLC16A3 with the metastatic scores in colon cancer data. Interestingly, we found that the expression levels of CTHRC1, FBN2, NTM, PDGFC, and PDLIM3 are positively correlated with the ssGSEA scores of metastatic-promoting genes in colon cancer (Spearman’s correlation test, p < 0.001) (Figure 8). Shujuan Ni et al. reported that the elevated level of CTHRC1 is associated with the progression of CRC [63]. PDGFC is correlated with early diagnosis, cancer grading, and metastatic disease of CRC [67]. In CRC patients with hepatic metastasis, the methylated FBN2 was detected in the patient’s serum [68]. In summary, these results indicated that the expression of CTHRC1, FBN2, NTM, PDGFC, and PDLIM3 is associated with tumor progression and metastasis of colon cancer cells. Furthermore, we validated the diagnostic efficacy of these key genes in colonic CAFs and found that the CTHRC1, NTM, PDGFC, PDLIM3, SLC16A3, and FBN2 genes have diagnostic efficiency in colonic fibroblast (Figure 9A). It indicates that the expression value of these key genes could be used in the diagnostic process. Finally, we validated the expression levels of these key genes in the colon tumor stroma dataset, and we revealed that the CTHRC1, NTM, PDGFC, and FBN2 genes are consistently altered in colon tumor stroma (Figure 9B). Also, CTHRC1, NTM, PDGFC, and PDLIM3 are consistently deregulated when compared the high-CAFs group with the low-CAFs group in the TCGA COAD cohort (Figure 9C). It indicates that stromal CAFs-derived transcriptomes may have a crucial contribution to colon cancer. However, the expression levels of PDLIM3 and SLC16A3 are not significantly altered in colon tumor stroma (p < 0.05) and the expression level of FBN2 showed the opposite trend in the high-CAFs group (Figure 9C). As far as we know, FBN2 is a protein-coding gene, which is associated with tissue microfibrils and may be involved in elastic fiber assembly [69]. It has been reported that FBN2 could have excellent diagnostic value for smooth muscle sarcoma and rhabdomyosarcoma [70, 71]. Besides, it also has been proved that FBN2 might both have tumor-suppressive effects and is a typical basement membrane marker in several types of cancers [72]. Previous studies have found that FBN2 harbour cancer-specific promoter methylation in human colorectal cancer [73]. Furthermore, clinical research has found that FBN2 gene methylation exhibited in 63% of tumor samples of patients with primary colorectal carcinoma, and FBN2 might be an early and frequent event in precancerous and cancerous lesions of the colon and rectum [68]. Although little is known about FBN2 biological function regarding epigenetic changes in human cancers, the methylation of these genes has great potential to detect early-stage colon cancer [74]. FBN2 annotation research showed that high expression of FBN2 was mainly enriched in extracellular matrix (ECM) receptor interaction and epithelial-mesenchymal transition (EMT) pathway [75]. In contrast, high expression of FBN2 was found as a risk factor in lung and gastric cancer [76, 77]. In our research, we found that FBN2 is highly expressed in normal fibroblasts, and downregulated FBN2 is consistently correlated with the shorter survival of colon cancer patients, this result gives rise to the idea to study the role of FBN2 in a deeper way. Since we divided COAD samples into the high-CAFs group versus the low-CAFs group and found a higher level of FBN2 in the high-CAFs group, suggesting that the tumor cell contributed to the higher expression of FBN2 but stromal CAFs contributed to the lower expression level of FBN2. Furthermore, in the TCGA COAD cohort, we found that the expression level of FBN2 is associated with the infiltrations of immune cells (Figure 6), indicating that tumor cells contributed to the higher expression level of FBN2 and stromal CAFs contributed to the lower level of FBN2, which ultimately associated with lowering survival time and increasing the immune infiltrations in colon cancer patients.
Altogether, these results indicate the expression of CAFs-derived key transcriptomes (CTHRC1, NTM, and PDGFC) is associated with poor survival prognosis, immunosuppression, tumor progression, and metastasis in human colon cancer. Numerous evidence indicated that immunohistochemistry is a highly effective substantial tool to predict survival prognosis in patients with various cancer types [78]. Since laser-capture micro-dissected stromal FFPE tissue can be utilized to identify molecular proteomic and transcriptomic profiling [79], measuring the key genes with immunohistochemistry in the laser micro-dissected CAFs may lead the clinicians to taking decisions in diagnosis and specific gene-oriented targeted therapy of colon cancer patients. However, for using these findings in clinical and therapeutic applications, further experimental and clinical verification would be necessary.
Conclusion
The identification of colonic CAFs-derived key transcriptomes may provide insight into the association of these key genes with survival prognosis, immunosuppression, tumor progression, and metastasis.
Data Availability Statement
The GEO datasets GSE46824, GSE70468, GSE17536, and GSE35602 were used in this study and are available in The National Biotechnology Information Centre Gene (NCBI-Gene) database (https://www.ncbi.nlm.nih.gov/gene). The TCGA-COAD cohort was downloaded from The Cancer Genome Atlas (TCGA) database (https://portal.gdc.cancer.gov/).
Author Contributions
All authors have contributed to the research concept, design, and interpretation of the data. RA and MS downloaded and analyzed the data. MU and JW conceived, drafted, wrote, and reviewed the final manuscript. All authors read and approved the final version of the document.
Conflict of Interest
The authors declare that the research was conducted in the absence of any commercial or financial relationships that could be construed as a potential conflict of interest.
Supplementary Material
The Supplementary Material for this article can be found online at: https://www.por-journal.com/articles/10.3389/pore.2022.1610350/full#supplementary-material
References
1. Valkenburg, KC, de Groot, AE, and Pienta, KC. Targeting the Tumour Stroma to Improve Cancer Therapy. Nat Rev Clin Oncol (2018) 15:366–81. doi:10.1038/s41571-018-0007-1
2. Zhang, R, Qi, F, Shao, S, Li, G, and Feng, Y. Human Colorectal Cancer-Derived Carcinoma Associated Fibroblasts Promote CD44-Mediated Adhesion of Colorectal Cancer Cells to Endothelial Cells by Secretion of HGF. Cancer Cel Int (2019) 19:192. doi:10.1186/s12935-019-0914-y
3. Musa, M, and Ali, A. Cancer-associated Fibroblasts of Colorectal Cancer and Their Markers: Updates, Challenges and Translational Outlook. Future Oncol (2020) 16:2329–44. doi:10.2217/fon-2020-0384
4. Unterleuthner, D, Neuhold, P, Schwarz, K, Janker, L, Neuditschko, B, Nivarthi, H, et al. Cancer-associated Fibroblast-Derived WNT2 Increases Tumor Angiogenesis in colon Cancer. Angiogenesis (2020) 23:159–77. doi:10.1007/s10456-019-09688-8
5. Wu, J, Liang, C, Chen, M, and Su, W. Association between Tumor-Stroma Ratio and Prognosis in Solid Tumor Patients: A Systematic Review and Meta-Analysis. Oncotarget (2016) 7:68954–65. doi:10.18632/oncotarget.12135
6. Berdiel-Acer, M, Sanz-Pamplona, R, Calon, A, Cuadras, D, Berenguer, A, Sanjuan, X, et al. Differences between CAFs and Their Paired NCF from Adjacent Colonic Mucosa Reveal Functional Heterogeneity of CAFs, Providing Prognostic Information. Mol Oncol (2014) 8:1290–305. doi:10.1016/j.molonc.2014.04.006
7. Sandberg, TP, Stuart, MPME, Oosting, J, Tollenaar, RAEM, Sier, CFM, and Mesker, WE. Increased Expression of Cancer-Associated Fibroblast Markers at the Invasive Front and its Association with Tumor-Stroma Ratio in Colorectal Cancer. BMC Cancer (2019) 19:284. doi:10.1186/s12885-019-5462-2
8. Xing, F, Saidou, J, and Watabe, K. Cancer Associated Fibroblasts (CAFs) in Tumor Microenvironment. Front Biosci (2010) 15:166–79. doi:10.2741/3613
9. Gascard, P, and Tlsty, TD. Carcinoma-associated Fibroblasts: Orchestrating the Composition of Malignancy. Genes Dev (2016) 30:1002–19. doi:10.1101/gad.279737.116
10. Cirri, P, and Chiarugi, P. Cancer-associated-fibroblasts and Tumour Cells: A Diabolic Liaison Driving Cancer Progression. Cancer Metastasis Rev (2012) 31:195–208. doi:10.1007/s10555-011-9340-x
11. Liu, T, Han, C, Wang, S, Fang, P, Ma, Z, Xu, L, et al. Cancer-associated Fibroblasts: an Emerging Target of Anti-cancer Immunotherapy. J Hematol Oncol (2019) 12:86. doi:10.1186/s13045-019-0770-1
12. Isella, C, Terrasi, A, Bellomo, SE, Petti, C, Galatola, G, Muratore, A, et al. Stromal Contribution to the Colorectal Cancer Transcriptome. Nat Genet (2015) 47:312–9. doi:10.1038/ng.3224
13. Becht, E, de Reyniès, A, Giraldo, NA, Pilati, C, Buttard, B, Lacroix, L, et al. Immune and Stromal Classification of Colorectal Cancer Is Associated with Molecular Subtypes and Relevant for Precision Immunotherapy. Clin Cancer Res (2016) 22:4057–66. doi:10.1158/1078-0432.CCR-15-2879
14. Zheng, H, Liu, H, Ge, Y, and Wang, X. Integrated Single-Cell and Bulk RNA Sequencing Analysis Identifies a Cancer Associated Fibroblast-Related Signature for Predicting Prognosis and Therapeutic Responses in Colorectal Cancer. Cancer Cel Int (2021) 21:552. doi:10.1186/s12935-021-02252-9
15. Dienstmann, R, Villacampa, G, Sveen, A, Mason, MJ, NieDzwiecki, D, NesbAkken, A, et al. Relative Contribution of Clinicopathological Variables, Genomic Markers, Transcriptomic Subtyping and Microenvironment Features for Outcome Prediction in Stage II/III Colorectal Cancer. Ann Oncol (2019) 30:1622–9. doi:10.1093/annonc/mdz287
16. Baulida, J. Epithelial-to-mesenchymal Transition Transcription Factors in Cancer-Associated Fibroblasts. Mol Oncol (2017) 11:847–59. doi:10.1002/1878-0261.12080
17. Liu, B, Pan, S, Liu, J, and Kong, C. Cancer-associated Fibroblasts and the Related Runt-Related Transcription Factor 2 (RUNX2) Promote Bladder Cancer Progression. Gene (2021) 775:145451. doi:10.1016/j.gene.2021.145451
18.NCBI. GEO Accession Viewer (2019). Available at: https://www.ncbi.nlm.nih.gov/geo/query/acc.cgi?acc=GSE46824 (Accessed Feb 13, 2019).
19. Ferrer-Mayorga, G, Gómez-López, G, Barbáchano, A, Fernandez-Barral, A, Pena, C, Pisano, DG, et al. Vitamin D Receptor Expression and Associated Gene Signature in Tumour Stromal Fibroblasts Predict Clinical Outcome in Colorectal Cancer. Gut (2017) 66:1449–62. doi:10.1136/gutjnl-2015-310977
20.Cancer Genome Atlas Network. Comprehensive Molecular Characterization of Human colon and Rectal Cancer. Nature (2012) 487:330–7. doi:10.1038/nature11252
21. Tang, Z, Li, C, Kang, B, Gao, G, and Zhang, Z. GEPIA: A Web Server for Cancer and normal Gene Expression Profiling and Interactive Analyses. Nucleic Acids Res (2017) 45:W98–W102. doi:10.1093/nar/gkx247
22. Mizuno, H, Kitada, K, Nakai, K, and Sarai, A. PrognoScan: A New Database for Meta-Analysis of the Prognostic Value of Genes. BMC Med Genomics (2009) 2:18. doi:10.1186/1755-8794-2-18
23. Smith, JJ, Deane, NG, Wu, F, Merchant, NB, Zhang, B, Jiang, A, et al. Experimentally Derived Metastasis Gene Expression Profile Predicts Recurrence and Death in Patients with colon Cancer. Gastroenterology (2010) 138:958–68. doi:10.1053/j.gastro.2009.11.005
24. Nishida, N, Nagahara, M, Sato, T, Mimori, K, Sudo, T, Tanaka, F, et al. Microarray Analysis of Colorectal Cancer Stromal Tissue Reveals Upregulation of Two Oncogenic miRNA Clusters. Clin Cancer Res (2012) 18:3054–70. doi:10.1158/1078-0432.CCR-11-1078
25. Davis, S, and Meltzer, PS. GEOquery: a Bridge between the Gene Expression Omnibus (GEO) and BioConductor. Bioinformatics (2007) 23:1846–7. doi:10.1093/bioinformatics/btm254
26. Ritchie, ME, Phipson, B, Wu, D, Hu, Y, Law, CW, Shi, W, et al. Limma powers Differential Expression Analyses for RNA-Sequencing and Microarray Studies. Nucleic Acids Res (2015) 43:e47. doi:10.1093/nar/gkv007
27. Wang, J, Uddin, MN, Akter, R, and Wu, Y. Contribution of Endothelial Cell-Derived Transcriptomes to the colon Cancer Based on Bioinformatics Analysis. Math Biosci Eng (2021) 18:7280–300. doi:10.3934/mbe.2021360
28. Kim, N, Kim, HK, Lee, K, Hong, Y, Cho, JH, Choi, JW, et al. Single-cell RNA Sequencing Demonstrates the Molecular and Cellular Reprogramming of Metastatic Lung Adenocarcinoma. Nat Commun (2020) 11:2285. doi:10.1038/s41467-020-16164-1
29. Subramanian, A, Tamayo, P, Mootha, VK, Mukherjee, S, Ebert, BL, Gillette, MA, et al. Gene Set Enrichment Analysis: A Knowledge-Based Approach for Interpreting Genome-wide Expression Profiles. Proc Natl Acad Sci U S A (2005) 102:15545–50. doi:10.1073/pnas.0506580102
30. Kanehisa, M, Furumichi, M, Tanabe, M, Sato, Y, and Morishima, K. KEGG: New Perspectives on Genomes, Pathways, Diseases and Drugs. Nucleic Acids Res (2017) 45:D353–D361. doi:10.1093/nar/gkw1092
31. Szklarczyk, D, Gable, AL, Lyon, D, Junge, A, Wyder, S, Huerta-Cepas, J, et al. STRING V11: Protein–Protein Association Networks with Increased Coverage, Supporting Functional Discovery in Genome-wide Experimental Datasets. Nucleic Acids Res (2019) 47:D607–D613. doi:10.1093/nar/gky1131
32. Chin, C-H, Chen, S-H, Wu, H-H, Ho, CW, Ko, MT, and Lin, CY. cytoHubba: Identifying Hub Objects and Sub-networks from Complex Interactome. BMC Syst Biol (2014) 8:S11. doi:10.1186/1752-0509-8-S4-S11
33. Shannon, P, Markiel, A, Ozier, O, Baliga, NS, Wang, JT, Ramage, D, et al. Cytoscape: A Software Environment for Integrated Models of Biomolecular Interaction Networks. Genome Res (2003) 13:2498–504. doi:10.1101/gr.1239303
34. Bader, GD, and Hogue, CW. An Automated Method for Finding Molecular Complexes in Large Protein Interaction Networks. BMC Bioinformatics (2003) 4:2. doi:10.1186/1471-2105-4-2
35. Yoshihara, K, Shahmoradgoli, M, Martínez, E, Vegesna, R, Kim, H, Torres-Garcia, W, et al. Inferring Tumour Purity and Stromal and Immune Cell Admixture from Expression Data. Nat Commun (2013) 4:2612. doi:10.1038/ncomms3612
36. Hänzelmann, S, Castelo, R, and Guinney, J. GSVA: Gene Set Variation Analysis for Microarray and RNA-Seq Data. BMC Bioinformatics (2013) 14:7. doi:10.1186/1471-2105-14-7
37. Tirosh, I, Izar, B, Prakadan, SM, Wadsworth, MH, Treacy, D, Trombetta, JJ, et al. Dissecting the Multicellular Ecosystem of Metastatic Melanoma by Single-Cell RNA-Seq. Science (2016) 352:189–96. doi:10.1126/science.aad0501
38. Aran, D, Hu, Z, and Butte, AJ. xCell: Digitally Portraying the Tissue Cellular Heterogeneity Landscape. Genome Biol (2017) 18:220. doi:10.1186/s13059-017-1349-1
39. Davoli, T, Uno, H, Wooten, EC, and Elledge, SJ. Tumor Aneuploidy Correlates with Markers of Immune Evasion and with Reduced Response to Immunotherapy. Science (2017) 355:eaaf8399. doi:10.1126/science.aaf8399
40. Liu, Z, Li, M, Jiang, Z, and Wang, X. A Comprehensive Immunologic Portrait of Triple-Negative Breast Cancer. Transl Oncol (2018) 11:311–29. doi:10.1016/j.tranon.2018.01.011
41. van der Weyden, L, Arends, MJ, Campbell, AD, Bald, T, Wardle-Jones, H, Griggs, N, et al. Genome-wide In Vivo Screen Identifies Novel Host Regulators of Metastatic Colonization. Nature (2017) 541:233–6. doi:10.1038/nature20792
42. Robin, X, Turck, N, Hainard, A, Tiberti, N, Lisacek, F, Sanchez, JC, et al. pROC: An Open-Source Package for R and S+ to Analyze and Compare ROC Curves. BMC Bioinformatics (2011) 12:77. doi:10.1186/1471-2105-12-77
43. Yang, Q, Wang, R, Wei, B, Peng, C, Wang, L, Hu, G, et al. Candidate Biomarkers and Molecular Mechanism Investigation for Glioblastoma Multiforme Utilizing WGCNA. Biomed Res Int (2018) 2018:e4246703. doi:10.1155/2018/4246703
44. Liu, Y. Survival Correlation of Immune Response in Human Cancers. Oncotarget (2019) 10:6885–97. doi:10.18632/oncotarget.27360
45. Uddin, MN, and Wang, X. The Landscape of Long Non-coding RNAs in Tumor Stroma. Life Sci (2021) 264:118725. doi:10.1016/j.lfs.2020.118725
46. Mousavi Ardehaie, R, Hashemzadeh, S, Behrouz Sharif, S, Ghojazadeh, M, Teimoori-Toolabi, L, and Sakhinia, E. Aberrant Methylated EDNRB Can Act as a Potential Diagnostic Biomarker in Sporadic Colorectal Cancer while KISS1 Is Controversial. Bioengineered (2017) 8:555–64. doi:10.1080/21655979.2017.1283458
47. Gao, D, Han, Y, Yang, Y, Herman, JG, Linghu, E, Zhan, Q, et al. Methylation of TMEM176A Is an Independent Prognostic Marker and Is Involved in Human Colorectal Cancer Development. Epigenetics (2017) 12:575–83. doi:10.1080/15592294.2017.1341027
48. Xu, Y, Xu, J, Yang, Y, Zhu, L, Li, X, and Zhao, W. SRGN Promotes Colorectal Cancer Metastasis as a Critical Downstream Target of HIF-1α. Cell Physiol Biochem (2018) 48:2429–40. doi:10.1159/000492657
49. Tang, J, Jiang, W, Liu, D, Luo, J, Wu, X, Pan, Z, et al. The Comprehensive Molecular Landscape of the Immunologic Co-stimulator B7 and TNFR Ligand Receptor Families in Colorectal Cancer: Immunotherapeutic Implications with Microsatellite Instability. OncoImmunology (2018) 7:e1488566. doi:10.1080/2162402X.2018.1488566
50. Jung, B, Staudacher, JJ, and Beauchamp, D. Transforming Growth Factor β Superfamily Signaling in Development of Colorectal Cancer. Gastroenterology (2017) 152:36–52. doi:10.1053/j.gastro.2016.10.015
51. Barrett, CW, Reddy, VK, Short, SP, Motley, AK, Lintel, MK, Bradley, AM, et al. Selenoprotein P Influences Colitis-Induced Tumorigenesis by Mediating Stemness and Oxidative Damage. J Clin Invest (2015) 125:2646–60. doi:10.1172/JCI76099
52. Li, D, Guo, J, Wang, S, Zhu, L, and Shen, Z. Identification of Novel Methylated Targets in Colorectal Cancer by Microarray Analysis and Construction of Co-expression Network. Oncol Lett (2017) 14:2643–8. doi:10.3892/ol.2017.6506
53. Uddin, MN, Li, M, and Wang, X. Identification of Transcriptional Signatures of Colon Tumor Stroma by a Meta-Analysis. J Oncol (2019) 2019:8752862. doi:10.1155/2019/8752862
54. Uddin, MN, Li, M, and Wang, X. Identification of Transcriptional Markers and microRNA–mRNA Regulatory Networks in Colon Cancer by Integrative Analysis of mRNA and microRNA Expression Profiles in Colon Tumor Stroma. Cells (2019) 8:E1054. doi:10.3390/cells8091054
55. Bhagwat, AS, and Vakoc, CR. Targeting Transcription Factors in Cancer. Trends Cancer (2015) 1:53–65. doi:10.1016/j.trecan.2015.07.001
56. Vishnoi, K, Viswakarma, N, Rana, A, and Rana, B. Transcription Factors in Cancer Development and Therapy. Cancers (2020) 12:E2296. doi:10.3390/cancers12082296
57. Li, D, Wei, P, Peng, Z, Huang, C, Tang, H, Jia, Z, et al. The Critical Role of Dysregulated FOXM1-PLAUR Signaling in Human colon Cancer Progression and Metastasis. Clin Cancer Res (2013) 19:62–72. doi:10.1158/1078-0432.CCR-12-1588
58. Joshi, S, Singh, AR, Zulcic, M, Bao, L, Messer, K, Ideker, T, et al. Rac2 Controls Tumor Growth, Metastasis and M1-M2 Macrophage Differentiation In Vivo. PLoS ONE (2014) 9:e95893. doi:10.1371/journal.pone.0095893
59. Xu, Y, and Pasche, B. TGF-β Signaling Alterations and Susceptibility to Colorectal Cancer. Hum Mol Genet (2007) 16:R14–20. doi:10.1093/hmg/ddl486
60. Mazzarelli, P, Pucci, S, and Spagnoli, LG. CLU and colon Cancer. The Dual Face of CLU: from normal to Malignant Phenotype. Adv Cancer Res (2009) 105:45–61. doi:10.1016/S0065-230X(09)05003-9
61. O’Brien, RM, Cannon, A, Reynolds, JV, Lysaght, J, and Lynam-Lennon, N. Complement in Tumourigenesis and the Response to Cancer Therapy. Cancers (2021) 13:1209. doi:10.3390/cancers13061209
62. Itatani, Y, Kawada, K, Inamoto, S, Yamamoto, T, Ogawa, R, Taketo, MM, et al. The Role of Chemokines in Promoting Colorectal Cancer Invasion/Metastasis. Int J Mol Sci (2016) 17:643. doi:10.3390/ijms17050643
63. Ni, S, Ren, F, Xu, M, Tan, C, Weng, W, Huang, Z, et al. CTHRC1 Overexpression Predicts Poor Survival and Enhances Epithelial mesenchymal Transition in Colorectal Cancer. Cancer Med (2018) 7:5643–54. doi:10.1002/cam4.1807
64. Yu, S, Wu, Y, Li, C, Qu, Z, Lou, G, Guo, X, et al. Comprehensive Analysis of the SLC16A Gene Family in Pancreatic Cancer via Integrated Bioinformatics. Sci Rep (2020) 10:7315. doi:10.1038/s41598-020-64356-y
65. Lu, C, Rong, D, Zhang, B, Zheng, W, Wang, X, Chen, Z, et al. Current Perspectives on the Immunosuppressive Tumor Microenvironment in Hepatocellular Carcinoma: Challenges and Opportunities. Mol Cancer (2019) 18:130. doi:10.1186/s12943-019-1047-6
66. Lorenzo-Sanz, L, and Muñoz, P. Tumor-Infiltrating Immunosuppressive Cells in Cancer-Cell Plasticity, Tumor Progression and Therapy Response. Cancer Microenviron (2019) 12:119–32. doi:10.1007/s12307-019-00232-2
67. Manzat Saplacan, RM, Balacescu, L, Gherman, C, Chira, RI, Craiu, A, Mircea, PA, et al. The Role of PDGFs and PDGFRs in Colorectal Cancer. Mediators Inflamm (2017) 2017:e4708076. doi:10.1155/2017/4708076
68. Hibi, K, Mizukami, H, Saito, M, Kigawa, G, Nemoto, H, and Sanada, Y. FBN2 Methylation Is Detected in the Serum of Colorectal Cancer Patients with Hepatic Metastasis. Anticancer Res (2012) 32:4371–4.
69. Zhang, H, Apfelroth, SD, Hu, W, Davis, EC, Sanguineti, C, Bonadio, J, et al. Structure and Expression of Fibrillin-2, a Novel Microfibrillar Component Preferentially Located in Elastic Matrices. J Cel Biol (1994) 124:855–63. doi:10.1083/jcb.124.5.855
70. Lian, X, Bond, JS, Bharathy, N, Boudko, SP, Pokidysheva, E, Shern, JF, et al. Defining the Extracellular Matrix of Rhabdomyosarcoma. Front Oncol (2021) 11:601957. doi:10.3389/fonc.2021.601957
71. Gu, H-Y, Zhang, C, Guo, J, Yang, M, Zhong, HC, Jin, W, et al. Risk Score Based on Expression of Five Novel Genes Predicts Survival in Soft Tissue Sarcoma. Aging (2020) 12:3807–27. doi:10.18632/aging.102847
72. Chu, L, Qu, Y, An, Y, Hou, L, Li, J, Li, W, et al. Induction of Senescence-Associated Secretory Phenotype Underlies the Therapeutic Efficacy of PRC2 Inhibition in Cancer. Cell Death Dis (2022) 13:155. doi:10.1038/s41419-022-04601-6
73. Yi, JM, Dhir, M, Guzzetta, AA, Iacobuzio-Donahue, CA, Heo, K, Yang, KM, et al. DNA Methylation Biomarker Candidates for Early Detection of colon Cancer. Tumour Biol (2012) 33:363–72. doi:10.1007/s13277-011-0302-2
74. Yi, JM. DNA Methylation Change Profiling of Colorectal Disease: Screening towards Clinical Use. Life (2021) 11:412. doi:10.3390/life11050412
75. Yan, M, Sun, H, Li, G, Chen, Q, Xie, Q, Zhang, W, et al. miR-139-5p Was Identified as Biomarker of Different Molecular Subtypes of Breast Carcinoma. Front Oncol (2022) 12:857714. doi:10.3389/fonc.2022.857714
76. Song, W, Bai, Y, Zhu, J, Zeng, F, Yang, C, Hu, B, et al. A Novel Prognostic Model Based on Epithelial-Mesenchymal Transition-Related Genes Predicts Patient Survival in Gastric Cancer. World J Surg Oncol (2021) 19:216. doi:10.1186/s12957-021-02329-9
77. Zhang, S, Zeng, X, Lin, S, Liang, M, and Huang, H. Identification of Seven-Gene Marker to Predict the Survival of Patients with Lung Adenocarcinoma Using Integrated Multi-Omics Data Analysis. J Clin Lab Anal (2022) 36:e24190. doi:10.1002/jcla.24190
78. Meng, J, Zhang, J, Xiu, Y, Jin, Y, Xiang, J, Nie, Y, et al. Prognostic Value of an Immunohistochemical Signature in Patients with Esophageal Squamous Cell Carcinoma Undergoing Radical Esophagectomy. Mol Oncol (2018) 12:196–207. doi:10.1002/1878-0261.12158
79. Pöschel, A, Beebe, E, Kunz, L, Amini, P, Guscetti, F, Malbon, A, et al. Identification of Disease-Promoting Stromal Components by Comparative Proteomic and Transcriptomic Profiling of Canine Mammary Tumors Using Laser-Capture Microdissected FFPE Tissue. Neoplasia (2021) 23:400–12. doi:10.1016/j.neo.2021.03.001
Keywords: colon cancer, survival time, immunosuppressive roles, metastatic scores, cancer-associated fibroblasts
Citation: Wang J, Akter R, Shahriar MF and Uddin MN (2022) Cancer-Associated Stromal Fibroblast-Derived Transcriptomes Predict Poor Clinical Outcomes and Immunosuppression in Colon Cancer. Pathol. Oncol. Res. 28:1610350. doi: 10.3389/pore.2022.1610350
Received: 07 February 2022; Accepted: 15 July 2022;
Published: 04 August 2022.
Edited by:
Anna Sebestyén, Semmelweis University, HungaryCopyright © 2022 Wang, Akter, Shahriar and Uddin. This is an open-access article distributed under the terms of the Creative Commons Attribution License (CC BY). The use, distribution or reproduction in other forums is permitted, provided the original author(s) and the copyright owner(s) are credited and that the original publication in this journal is cited, in accordance with accepted academic practice. No use, distribution or reproduction is permitted which does not comply with these terms.
*Correspondence: Md. Nazim Uddin, bmF6aW1iaW9AeWFob28uY29t