- 1Institute of Cancer, Xinqiao Hospital, Third Military Medical University, Chongqing, China
- 2Chongqing Key Laboratory of Tumor Immunotherapy, Chongqing, China
- 3Department of Thoracic Surgery, The Third Affiliated Hospital of Kunming Medical University, Kunming, China
- 4Center of Growth, Metabolism and Aging, Key Laboratory of Bio-Resources and Eco-Environment, College of Life Sciences, Sichuan University, Chengdu, China
- 5Research Institute, GloriousMed Clinical Laboratory (Shanghai) Co., Ltd., Shanghai, China
Somatostatin receptor 2 (SSTR2), the most abundant receptor of somatostatin (SST), possesses immunoreactivity and is altered in many cancers. However, the association between SSTR2 and efficacy of immune checkpoint inhibitors (ICIs) has not yet been reported. Immunohistochemistry (IHC) information across 20 cancers was collected from the Human Protein Atlas (HPA) and used to analyze the expression of SSTR2. Immune signatures collected from public databases, such as BioCarta or Reactome, were used to investigate the association between SSTR2 and the tumor microenviroment in the Cancer Genome Atlas (TCGA). Data from cohorts treated with ICIs were collected to assess whether SSTR2 is associated with benefits from ICIs treatment. In the HPA, we found the SSTR2 IHC-positive rate of 13 cancers to be above 50%. Five types of cancer express SSTR2 mildly (positive rate: 25%–50%), while the remaining two types of cancer barely stained SSTR2-positive (positive rate: 0%–24%). In TCGA analysis, immune cell signatures and immune function pathways were enriched in high SSTR2 expression groups in most cancers. In each ICIs treated cohort, patients with high SSTR2 expression experienced numerically superior objective response rate (Braun: 14.8% vs 13.4%, p = 0.85; Gide: 69.4% vs 40.5%, p = 0.025; Mariathasan: 22.4% vs 16.7%, p = 0.233; Miao: 37.5% vs 11.8%; Riaz: 32.0% vs 7.7%, p = 0.067) and overall survival (Braun: HR (95%CI): 0.80 [0.62–1.04], p = 0.80; Gide: HR (95%CI): 0.61 [0.29–1.30], p = 0.20; Mariathasan: HR (95%CI): 0.83 [0.64–1.08], p = 0.16; Miao: HR (95%CI): 0.24 [0.086–0.65], p = 0.0028; Nathanson cohort: HR (95%CI): 0 [0-inf], p = 0.18; Riaz: HR (95%CI): 0.24 [0.086–0.65], p = 0.028) than patients with low SSTR2 expression. In pooled cohort, we found these differences were significant (Pool: 24.6% vs 16.7%, p = 0.0077; HR (95% CI): 0.77 [0.65–0.91], p = 0.0018). Our results suggest that SSTR2 is a potential predictive biomarker for response to ICIs.
Introduction
Immune checkpoint inhibitors (ICIs), which are mainly comprised of anti-programmed cell death (ligand)-1 (PD-1/PD-L1) and anti-cytotoxic T lymphocyte-associated antigen 4 (CTLA-4) drugs, have revolutionized the therapeutic landscape for many advanced cancers (1–4). However, limited response rate and occasional adverse reactions make it difficult to implement ICIs in clinical practice (5, 6). Biomarkers are helpful in identifying ICIs-sensitive patients, protecting them from unnecessary adverse reactions and reducing financial burden. Thus, further research regarding predictive biomarkers for ICIs is urgently needed (7, 8).
Recent studies have discovered various predictive biomarkers for ICIs, including PD-L1 immunohistochemistry (IHC), microsatellite instability (MSI), tumor mutation burden (TMB) and multiple gene signatures (9–14). However, there are limitations to their use in clinical practice. For example, some PD-L1 positive patients were not responsive to ICIs, while those that did not express PD-L1 were responsive to ICIs (15, 16). Additionally, TMB calculation lacked a standardized formula and unified cut-off value (17). Furthermore, only PD-L1 and MSI have been clinically validated (18). Thus, finding novel predictive biomarkers is beneficial for the clinical practice of ICIs.
Somatostatin receptor 2 (SSTR2), the most abundant somatostatin (SST) receptor, is a member of the G protein-coupled receptor family (19). In previous studies, SSTR2 was proven to be overexpressed in neuroendocrine neoplasms (20, 21). Recent studies show that SSTR2 is significantly methylated in colorectal cancer (22). SSTR2 was also proven to be associated with tumorigenesis in gastric cancer and breast cancer (23, 24). Additionally, researchers found that binding of SST and SSTR2 could inhibit immune cells cytokine release and have an effect on the tumor microenvironment (TME) (25, 26). However, the relationship between SSTR2 and TME and the association between SSTR2 and prognosis of ICIs have not yet been reported.
In this study, we investigate the expression of SSTR2 across multiple types of cancer by collecting SSTR2 IHC data from the Human Protein Atlas (HPA). RNA-seq information from the Cancer Genome Atlas (TCGA) database and immune signatures were used to analyze the underlying mechanism of the effect of SSTR2 on TME. Then, we collected the mutation and survival information of TCGA patients to investigate the association between SSTR2 alteration and conventional treatment prognosis. By using collected RNA-seq data and clinical information of patients treated with ICIs, we further investigated the association between SSTR2 and the efficacy of ICIs treatment.
Methods
Public Data Collection
The SSTR2 IHC results were obtained from the HPA (https://www.proteinatlas.org/). The antibody used in IHC was HPA007264, and the further information of antibody was provided in https://www.proteinatlas.org/ENSG00000180616-SSTR2/antibody. The “high,” “medium”, “low”, and “not detected” stain levels were defined by the HPA. Patients RNA-seq data across 33 cancers from the TCGA database were used to explore the underlying mechanism of the effect of SSTR2 on the immune microenvironment. Mutation information from TCGA was used to investigate the effect of SSTR2 alteration on prognosis. Because of the possible effects of a physiological barrier on immune cell infiltration LGG, GBM, TGCT, THYM, and UVM were excluded from our study. DLBC and LAML were excluded because they were non-solid cancers.
The clinical and RNA-seq data of one bladder cancer cohort (Mariathasan cohort: n = 348), three melanoma cohorts (Gide cohort: n = 73; Nathanson cohort: n = 9; Riaz cohort: n = 51), and two renal cell carcinoma cohorts (Braun cohort: n = 311; Miao cohort: n = 33) were collected and consolidated to investigate the possible effects of SSTR2 on ICIs treatment prognosis (27–32). All patients were treated with anti-PD-1/PD-L1, anti-CTLA4, or a combination of anti-PD-1/PD-L1 and anti-CTLA4 drugs. The ICIs treatment efficacy was defined by using Response Evaluation Criteria in Solid Tumors, version 1.1. When patients achieved complete response or partial response, they were considered objective response to ICIs. When patients achieved objective response, or were evaluated keeping in stable disease for longer than 6 months, they were noted responders of ICIs treatment. All patients were divided into an SSTR2-high group or SSTR2-low group based on the median SSTR2 expression.
Gene Set Enrichment Analysis
The immune cell (T cell, central memory CD8 T cell, activated CD8 T cell, effector memory CD8 T cell, type 1 T helper cell, central memory CD4 T cell, activated CD4 T cell, and effector memory CD4 T cell) signatures were collected from public studies (33, 34). The immune functional pathway signatures (interferon alpha/beta signature, T helper pathway, interleukin 15 signature, inflame pathway, interleukin 2 signature, T cytotoxic signature, and T cell receptor activation (TCRA) pathway) were extracted from the BioCarta or Reactome databases. Hallmark gene signatures (hallmark interferon-γ signature and hallmark inflammatory response signature) were collected from the gene set enrichment analysis (GSEA) hallmark gene set (https://www.gsea-msigdb.org/gsea/index.jsp). The GSEA method was described in published research (35). The Pearson correlation test was used to analyze the correlation between SSTR2 expression and immune signature scores. All signature scores were calculated through the use of a single-sample (ss) GSEA method in R package GSVA (https://www.bioconductor.org/packages/release/bioc/html/GSVA.html).
Statistical Analysis
The log-rank test and Kaplan–Meier KM method were used to compare overall survival (OS) between the SSTR2-high group and SSTR2-low group in cohorts treated with ICIs. Univariate Cox analysis was used to define high SSTR2 expression as protective (0 < HR < 1) or as a risk factor (HR > 1) for prognosis of ICIs treatment. Chi-Squared Test was used to compare objective response rate and responders percentage between SSTR2-high expression group and SSTR2-low expression group in ICIs treated cohorts with sufficiently high case numbers (case number >40). The results were considered significant when p value <0.05. All statistical analyses were performed using R version 4.0.0.
Results
Expression of SSTR2 Across Multiple Cancers
To investigate the expression of SSTR2 in different cancers, we consolidated the IHC data from the HPA. We found the SSTR2 IHC-positive rate of 13 cancers to be above 50% (Figure 1A). Five cancers express SSTR2 mildly (positive rate: 25%–50%) and two cancers barely expressed SSTR2 (positive rate: 0%–24%, Figure 1A). We found that patients with carcinoid and thyroid cancer have the highest positive rate of SSTR2 IHC among 20 types of cancer (100%, Figure 1B). The cancers with the next highest SSTR2 IHC-positive rates included colorectal cancer (91.7%), liver cancer (91.7%) and urothelial cancer (91.7%, Figure 1B). Additionally, one stomach cancer patient and one skin cancer patient showed a high staining of SSTR2 (Figure 1B). The lowest positive SSTR2 IHC rate was found in prostate cancer (16.7%, Figure 1B). Only prostate cancer and renal cancer SSTR2 IHC rates were below 25% (Figure 1B). Our findings suggested that SSTR2 expression varies and is widely distributed across multiple cancer types.
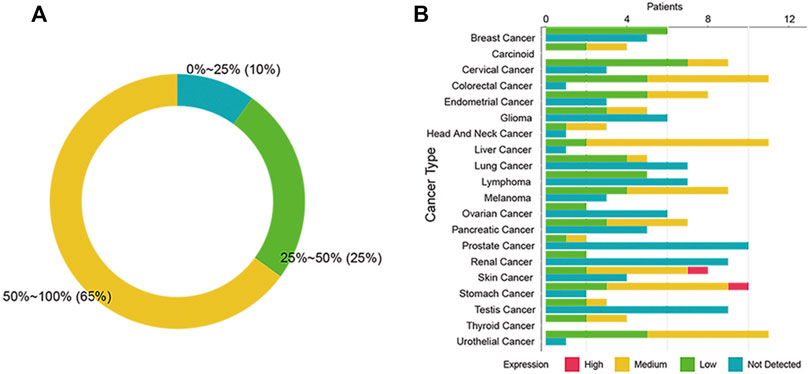
FIGURE 1. Expression of SSTR2 across 20 cancers in the human protein atlas. (A) The summary graph of the SSTR2 immunohistochemistry (IHC) positive rate in the human protein atlas. (B) The SSTR2 IHC results of 20 cancers.
SSTR2 is Associated With an Activated Immune Microenvironment
We investigated the association of SSTR2 expression and immune microenvironment through TCGA database. By using ssGSEA analysis, we found that T cell signature tends to enrich the tumor microenvironment in SSTR2-high groups in most cancers (96.15%, Figure 2A). Then, we analyzed the subpopulation of T cells. The SSTR2-high groups have a higher median of central memory CD8 T cell scores, activated CD8 T cell scores, and effector memory CD8 T cell scores in most cancers (central memory CD8 T cell scores: 96.5%; activated CD8 T cell scores: 96.15%; effector memory CD8 T cell scores: 96.5%; Figures 2B–D), suggesting better T cell infiltration may be possible in SSTR2-high groups. Type 1 helper cells and CD4 T cells exert a crucial effect on the anti-tumor environment. We also calculated the type 1 helper cell scores and CD4 T cell subpopulation scores of TCGA patients. Median type 1 helper cell scores in SSTR2-high groups were higher than those of SSTR2-low groups in all 26 cancers (Figure 2E). Additionally, SSTR2-high groups of most cancers had higher median central memory CD4 T cell scores, activated CD4 T cell scores, and effector memory CD4 T cell scores (96.15%, 80.77%, and 88.46%, respectively; Figures 2F–H). Our findings suggest that SSTR2-high groups may have better immune cell infiltration than SSTR2-low groups in various cancers.
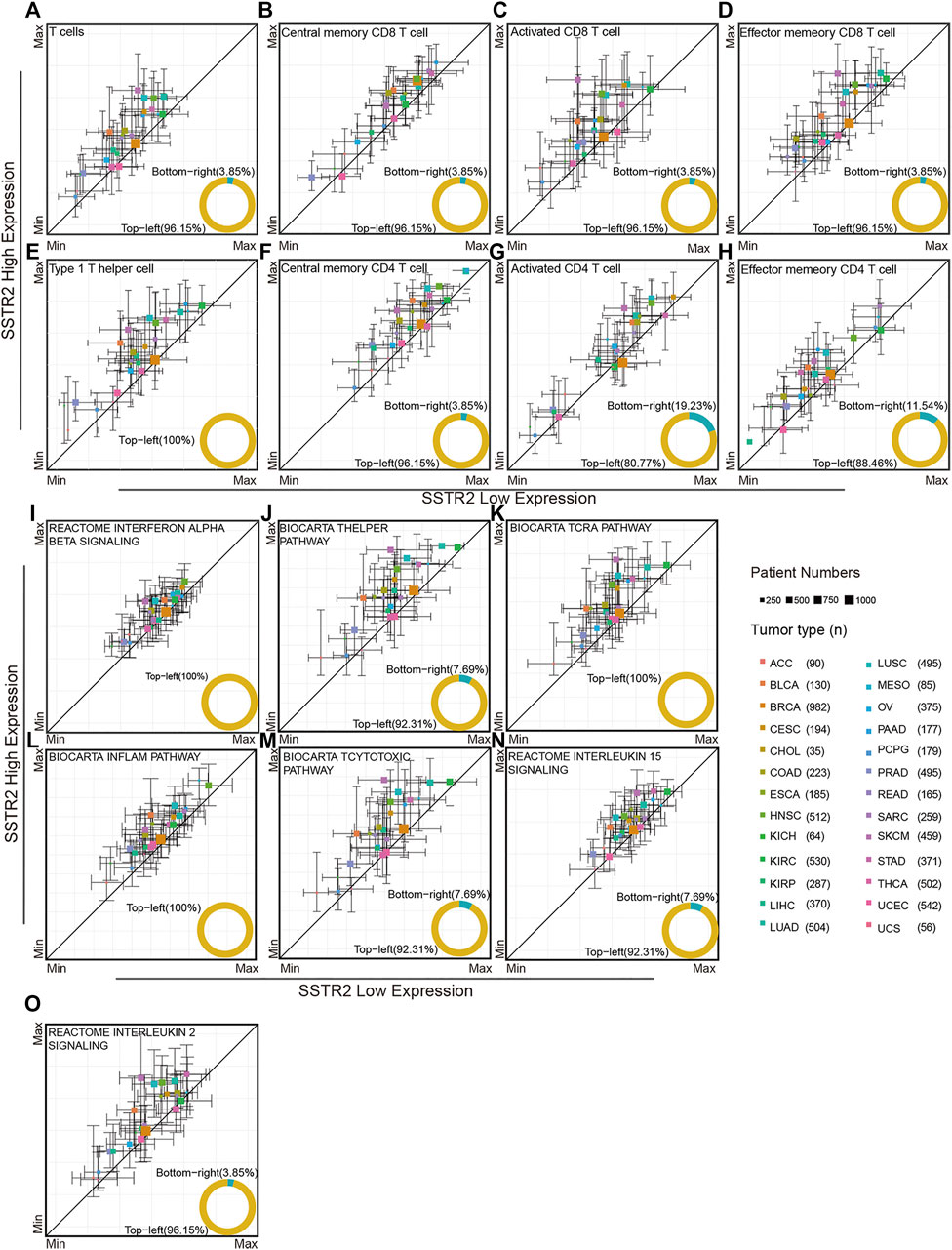
FIGURE 2. Immune signatures compared between the SSTR2-high group and SSTR2-low group. (A–H) Immune cell signatures in SSTR2-high and SSTR2-low groups. (I–O) Immune functional pathway signatures in SSTR2-high and SSTR2-low groups. The square point Y-axis positions were the scores median of SSTR2-high group, X-axis positions were the scores median of SSTR2-low group. Bars show 25%–75% scores range of different groups in each cancer.
We further investigated the immune functional pathway signature distribution across multiple cancers. The interferon alpha beta signature, T helper pathway, and TCRA pathway of SSTR2-high groups were higher than those of SSTR2-low groups in most cancers (interferon alpha beta signature: 100%; T helper signature: 92.31%; and TCRA pathway: 100%, Figures 2I–K), suggesting that the SSTR2-high group has better T cell activation than the SSTR2-low group in most cancers. The same results were observed in the inflammatory pathway and T cytotoxic pathway, suggesting that SSTR2-high groups showed a stronger inflammatory and cytotoxic immune environment than SSTR2-low groups in most cancers (inflammatory pathway: 100%; T cytotoxic pathway: 92.31%, Figures 2L,M). T cells survival in tumor tissue is vital to sustain an anti-tumor response, which relies on the interleukin 15 pathway and interleukin 2 pathway. Our results show that the interleukin 15 pathway appeared to increase in activity in SSTR2-high groups in 92.31% of cancers, and the interleukin 2 pathway appeared to increase in activity in SSTR2-high groups in 96.15% of cancers (Figures 2N,O). Our findings suggest that better activation and maintenance of cell-mediated immunity may exist in patients with a high expression of SSTR2.
We then performed GSEA analysis to compare the immune microenvironment between SSTR2-high patients and SSTR2-low patients. We found that immune cell signatures were higher in SSTR2-high patients in TCGA pooled cohort (activated CD8 T cell signature: normalized enrichment score (NES) = 2.25, p < 0.001; type 1 helper cell signature: NES = 2.36, p < 0.001, Figures 3A,B). Immune functional signature results were consistent with those of the immune cell signatures (hallmark interferon-γ signature: NES = 2.29, p < 0.001; hallmark inflammatory response signature: NES = 2.28, p < 0.001, Figures 3C,D). We then analyzed the correlation between SSTR2 expression and immune signature ssGSEA scores. We found that SSTR2 tends to be positively correlated with immune signature scores in most cancers. In BLCA, KICH, LUSC, PRAD and SKCM, SSTR2 expression is positively correlated with four signatures (Figures 3E–H). Our findings suggest that high SSTR2 expression is accompanied by an actived immune microenvironment in various cancers.
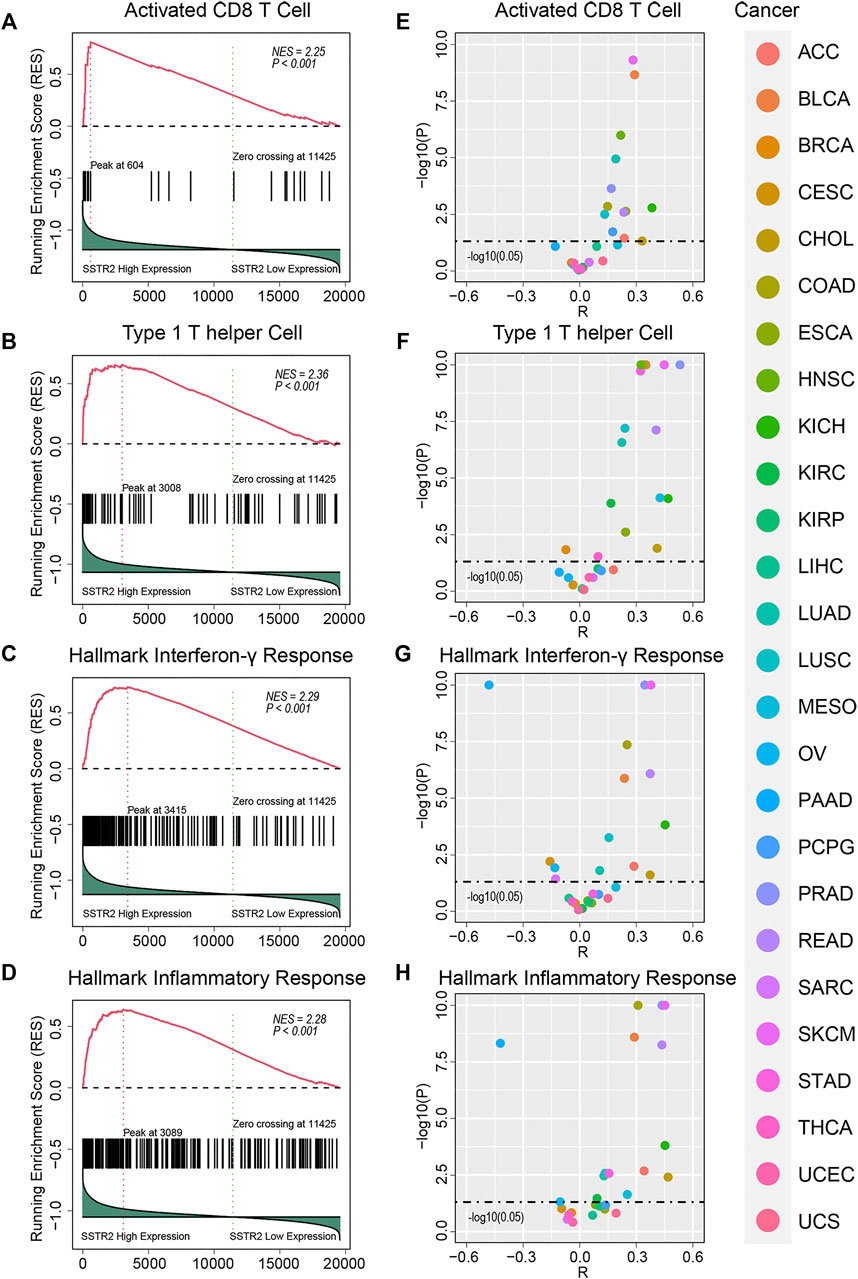
FIGURE 3. Immune signature enrichment results and correlation between SSTR2 expression and immune signatures scores. (A–D) immune signatures enrichment plots in TCGA pooled Q18 cohort. (E–H) Correlation summary plots of SSTR2 expression and immune signature scores.
SSTR2 is Not a Prognostic Factor in TCGA Pooled Cohort
We then investigated the effects of SSTR2 expression and alteration on prognosis in TCGA. We found the average alteration rate of SSTR2 in TCGA was 1.18% (Supplementary Figure S1). The top five cancer with highest alteration rate were UCEC, COAD, SKCM, READ, STAD (UCEC: 5.85%; COAD: 3.51%; SKCM: 3.43%; READ: 2.19%; STAD: 1.37%; Supplementary Figure S1). Additionally, there was no significant difference between OS in SSTR2-high patients and SSTR2-low patients (HR (95% CI): 0.94 [0.86–1.03], p = 0.20, Supplementary Figure S2). SSTR2 mutation likely did not affect the prognosis in TCGA pooled cohort (HR (95% CI): 0.77 [0.49–1.19], p = 0.22, Supplementary Figure S2). This finding suggests that SSTR2 is not a prognostic factor in TCGA pan-cancers cohort.
SSTR2 is Associated With Prognosis of ICIs Treatment
We then aimed to investigate the effect of SSTR2 expression on the prognosis of ICIs treatment. With the exception of the Nathanson cohort, the objective response rates of SSTR2-high groups were numerically higher than those of SSTR2-low groups (Braun: 14.8% vs 13.4%; Gide: 69.4% vs 40.5%; Mariathasan: 22.4% vs 16.7%; Miao: 37.5% vs 11.8%; Riaz: 32.0% vs 7.7%; Pooled: 24.6% vs 16.7%; Figure 4A). As Chi-Squared Test showed, in Gide cohort and Pooled cohort, the differences were significant (Gide: p = 0.025; Pooled: p = 0.0077; Figure 4A). Consistently, the SSTR2-high groups in the Braun, Gide, Mariathasan, Miao and pooled cohorts, had numerically higher response rate than the SSTR2-low groups (Braun: 54.8% vs 53.2%; Gide: 80.6% vs 59.5%; Mariathasan: 39.7% vs 30.5%; Miao: 56.3% vs 35.3%; Pooled: 51.0% vs 42.7%; Figure 4B), but only in pooled cohort, the result was significant (p = 0.02; Figure 4B). These results might suggest that patients with high SSTR2 expression are more likely to respond to ICIs than patients with low SSTR2 expression. Thus, We compared the patients OS between SSTR2 high expression group and SSTR2 low expression group. We found that patients with high SSTR2 expression experienced significantly longer OS than patients with low SSTR2 expression in the Miao, Riaz, and pooled cohorts (Miao: HR (95% CI): 0.24 [0.086–0.65], p = 0.0028, Riaz: HR (95% CI): 0.24 [0.086–0.65], p = 0.028, Pool: HR (95% CI): 0.77 [0.65–0.91], p = 0.0018, Figures 4F,H,I). The same results were observed in the Braun, Gide, Mariathasan, and Nathanson cohorts; however, these results were not found to be significant (Braun: HR (95% CI): 0.80 [0.62–1.04], p = 0.80; Gide: HR (95% CI): 0.61 [0.29–1.30], p = 0.200; Mariathasan: HR (95% CI): 0.83 [0.64–1.08], p = 0.16; Nathanson cohort: HR (95% CI): 0 [0-inf], p = 0.18, Figures 4C–E,G). Our findings suggest that patients with high SSTR2 expression might obtain more benefits from ICIs, such as a higher response rate and longer OS, than patients with low SSTR2 expression.
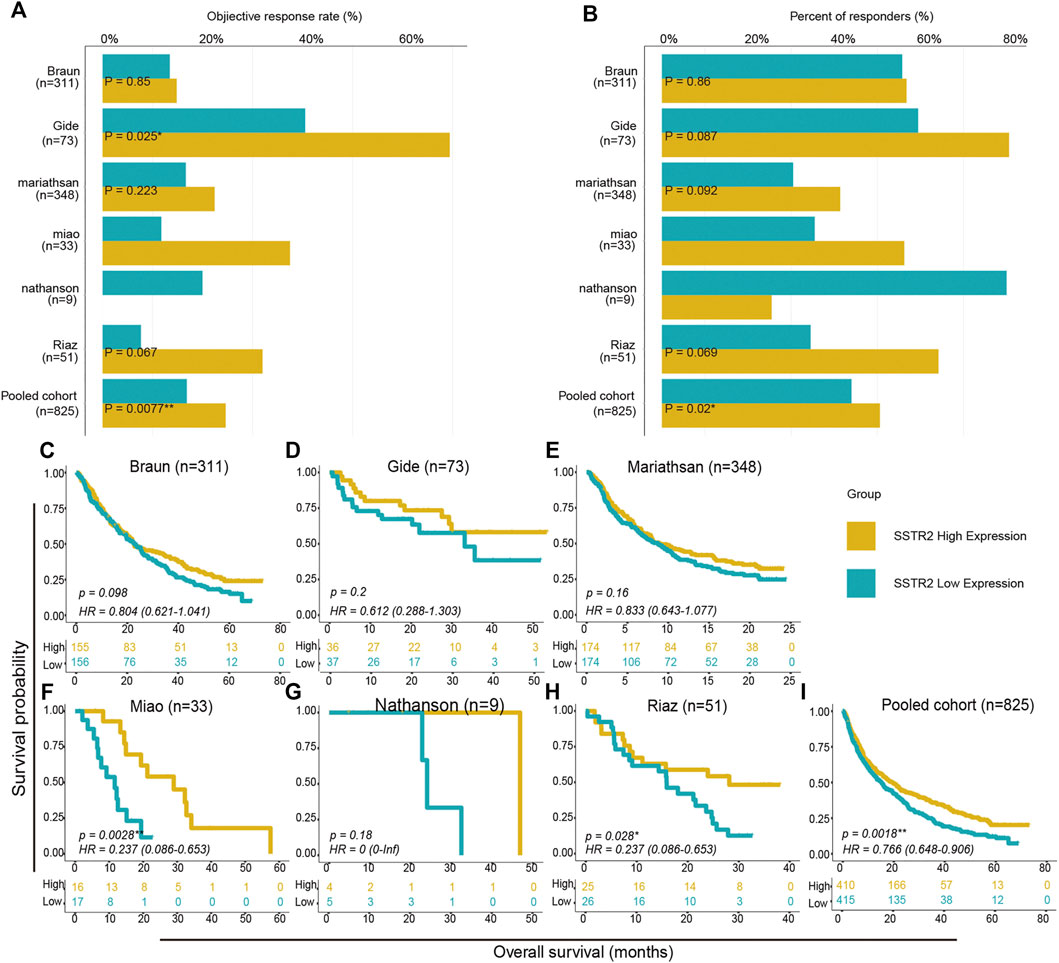
FIGURE 4. Survival analysis between SSTR2-high groups and SSTR2-low groups in ICIs treated cohorts. (A,B) Histogram describing objective response rate and responder percentage. (C–I) Kaplan-Meier curves of overall survival (OS) in ICIs treated cohorts comparing patients with high and low SSTR2 expression.
Discussion
The clinical use of ICIs has been proven to result in a better prognosis than conventional treatments in multiple types of cancer. However, the majority of patients do not respond to ICIs. Thus, biomarkers for predicting patients who can benefit from ICIs need to be investigated. In this study, we found that high expression of SSTR2 is associated with an activated immune microenvironment across multiple cancers. We then analyzed whether SSTR2 expression was associated with the efficacy of ICIs across multiple types of cancer. Our results suggest that SSTR2 expression varies among the types of cancer and patients with high SSTR2 expression could obtain longer OS after ICIs treatment. The SSTR2-high groups were also found to have a numerically higher objective response rate and more patients responded to ICIs treatment than in the SSTR2-low groups.
SST, which is mainly produced by the nervous system and peripheral digestive system, is a strong inhibitory peptide of secretory response of target cells, including inhibition of release of growth hormone, gastro-intestinal hormones and pancreatic enzymes (36). The function of SST has been profoundly investigated in previous studies using its receptors (SSTR1-SSTR5) as mediators (25). Among all SST receptors, SSTR2 is the most abundant (19). Additionally, SSTR2 is expressed in human pancreatic tissue, but could be loss in pancreatic cancers and derived cell lines(37–39). Previous studies have demonstrated that the combination of SST and SSTR2 could inhibit cytokine release from immune cells (26). In colorectal cancer, SSTR2 was shown to be significantly methylated, which results in SSTR2 function loss (22). Some researchers suggest that the combination of SST and SSTR2 may affect the TME, but clinical evidence is lacking (25). Our study investigated the association between SSTR2 expression and immune signatures. We found that high SSTR2 expression groups have higher median immune cell infiltration scores and immune function pathway scores compared with low SSTR2 expression groups, suggesting that high SSTR2 expression is associated with better immune infiltration, activation, and maintenance. This may account for the improvement of OS in patients with high SSTR2 expression in ICIs treated cohorts. Currently, the SSTR2 effects on TME are not well-investigated, and our study could bring new insights to the role that SSTR2 plays in TME.
Previous studies have developed various biomarkers that can screen patients who will respond to ICIs. Currently, PD-L1 IHC and TMB are the major biomarkers, however, they have limitations. For example, there are some patients without PD-L1 expression that can still respond to ICIs, while others with high PD-L1 expression do not benefit from ICIs treatment (40, 41). TMB serves as a surrogate indicator of tumor neoantigen and has no standard calculation formula or cut-off value (17). Furthermore, there are controversies regarding the use of TMB in ICIs prognosis in recent studies (42). MSI is another biomarker approved for ICIs clinical practice. However, intertumoral heterogeneity and intratumoral heterogeneity, which exist widely in tumors, interfere with the action of MSI. Moreover, multiple gene signatures, including immune cell infiltration scores and IFN-γ signatures, are currently not available for clinical use due to their high cost (43, 44). Our study demonstrates that high expression of SSTR2 is associated with high objective response rate and longer OS in ICIs treated cohorts. This indicates that SSTR2 could be a potential biomarker for response to ICIs.
This study has several limitations. First, limited information about SSTR2 mutation in ICIs treated cohorts prevented us from investigating the effect of functional SSTR2 mutation on ICIs treated patient prognosis. More molecular studies including cell line and animal models are needed to clarify the underlying mechanism of the effect of SSTR2 on TME. Second, limited IHC results in a single cancer type may cause statistical bias; pooled analysis and consistent results from multiple cancers could minimize this bias. Third, as patient numbers in Nathanson cohort and Miao Cohort were limited, we were not able to perform statistical tests for their responders.
Our study explored the association between SSTR2 expression and immune signatures with ICIs treatment efficacy across multiple cancers. We found that high SSTR2 expression in patients had enduring clinical benefits and was associated with longer OS and activated immunity. Therefore, SSTR2 could be a novel potential predictive biomarker for identifying patients who may benefit from ICIs treatment.
Data Availability Statement
The original contributions presented in the study are included in the article/Supplementary Material, further inquiries can be directed to the corresponding authors.
Author Contributions
Concept: QJ and BZ; Design: QJ and BZ; Literature search: AW and YY; Manuscript preparation: AW and YY; Data acquisition: ZJ and YG; Statistical analysis: AW, YY, HC, YG, and ZJ; Data analysis: AW, YY, HC, and YG; Manuscript editing: QJ and BZ; Manuscript review: QJ and BZ.
Funding
This research was supported by grants from the National Natural Science Foundation of China (Grant number: 81802797).
Conflict of Interest
Author ZJ was employed by the company GloriousMed Clinical Laboratory (Shanghai) Co., Ltd.
The remaining authors declare that the research was conducted in the absence of any commercial or financial relationships that could be construed as a potential conflict of interest.
Acknowledgments
We thank all the R programming package developers.
Supplementary Material
The Supplementary Material for this article can be found online at: https://www.por-journal.com/articles/10.3389/pore.2022.1610196/full#supplementary-material
Supplementary Figure S1 | The alteration rate of SSTR2 in TCGA database.
Supplementary Figure S2 | The survival analysis of SSTR2 in TCGA pooled cohort.
References
1. Gandhi, L, Rodríguez-Abreu, D, Gadgeel, S, Esteban, E, Felip, E, De Angelis, F, et al. Pembrolizumab Plus Chemotherapy in Metastatic Non-small-cell Lung Cancer. N Engl J Med (2018) 378:2078–92. doi:10.1056/NEJMoa1801005
2. Garon, EB, Hellmann, MD, Rizvi, NA, Carcereny, E, Leighl, NB, Ahn, M-J, et al. Five-Year Overall Survival for Patients with Advanced Non‒Small-Cell Lung Cancer Treated with Pembrolizumab: Results from the Phase I KEYNOTE-001 Study. Jco (2019) 37:2518–27. doi:10.1200/JCO.19.00934
3. Larkin, J, Chiarion-Sileni, V, Gonzalez, R, Grob, J-J, Rutkowski, P, Lao, CD, et al. Five-Year Survival with Combined Nivolumab and Ipilimumab in Advanced Melanoma. N Engl J Med (2019) 381:1535–46. doi:10.1056/NEJMoa1910836
4. Rosenberg, JE, Hoffman-Censits, J, Powles, T, van der Heijden, MS, Balar, AV, Necchi, A, et al. Atezolizumab in Patients with Locally Advanced and Metastatic Urothelial Carcinoma Who Have Progressed Following Treatment with Platinum-Based Chemotherapy: a Single-Arm, Multicentre, Phase 2 Trial. The Lancet (2016) 387:1909–20. doi:10.1016/S0140-6736(16)00561-4
5. Mok, TSK, Wu, YL, Kudaba, I, Kowalski, DM, Cho, BC, Turna, HZ, et al. Pembrolizumab versus Chemotherapy for Previously Untreated, PD-L1-Expressing, Locally Advanced or Metastatic Non-small-cell Lung Cancer (KEYNOTE-042): a Randomised, Open-Label, Controlled, Phase 3 Trial. Lancet (2019) 393:1819–30. doi:10.1016/S0140-6736(18)32409-7
6. Ouwerkerk, W, van den Berg, M, van der Niet, S, Limpens, J, and Luiten, RM. Biomarkers, Measured during Therapy, for Response of Melanoma Patients to Immune Checkpoint Inhibitors: a Systematic Review. Melanoma Res (2019) 29:453–64. doi:10.1097/CMR.0000000000000589
7. Havel, JJ, Chowell, D, and Chan, TA. The Evolving Landscape of Biomarkers for Checkpoint Inhibitor Immunotherapy. Nat Rev Cancer (2019) 19:133–50. doi:10.1038/s41568-019-0116-x
8. Zou, W, Wolchok, JD, and Chen, L. PD-L1 (B7-H1) and PD-1 Pathway Blockade for Cancer Therapy: Mechanisms, Response Biomarkers, and Combinations. Sci Transl Med (2016) 8:328rv324. doi:10.1126/scitranslmed.aad7118
9. Marcus, L, Lemery, SJ, Keegan, P, and Pazdur, R. FDA Approval Summary: Pembrolizumab for the Treatment of Microsatellite Instability-High Solid Tumors. Clin Cancer Res (2019) 25:3753–8. doi:10.1158/1078-0432.CCR-18-4070
10. Prat, A, Navarro, A, Paré, L, Reguart, N, Galván, P, Pascual, T, et al. Immune-Related Gene Expression Profiling after PD-1 Blockade in Non-small Cell Lung Carcinoma, Head and Neck Squamous Cell Carcinoma, and Melanoma. Cancer Res (2017) 77:3540–50. doi:10.1158/0008-5472.CAN-16-3556
11. Riaz, N, Havel, JJ, Kendall, SM, Makarov, V, Walsh, LA, Desrichard, A, et al. Recurrent SERPINB3 and SERPINB4 Mutations in Patients Who Respond to Anti-CTLA4 Immunotherapy. Nat Genet (2016) 48:1327–9. doi:10.1038/ng.3677
12. Rizvi, NA, Hellmann, MD, Snyder, A, Kvistborg, P, Makarov, V, Havel, JJ, et al. Mutational Landscape Determines Sensitivity to PD-1 Blockade in Non-small Cell Lung Cancer. Science (2015) 348:124–8. doi:10.1126/science.aaa1348
13. Taube, JM, Klein, A, Brahmer, JR, Xu, H, Pan, X, Kim, JH, et al. Association of PD-1, PD-1 Ligands, and Other Features of the Tumor Immune Microenvironment with Response to Anti-PD-1 Therapy. Clin Cancer Res (2014) 20:5064–74. doi:10.1158/1078-0432.CCR-13-3271
14. Tumeh, PC, Harview, CL, Yearley, JH, Shintaku, IP, Taylor, EJM, Robert, L, et al. PD-1 Blockade Induces Responses by Inhibiting Adaptive Immune Resistance. Nature (2014) 515:568–71. doi:10.1038/nature13954
15. Bellmunt, J, de Wit, R, Vaughn, DJ, Fradet, Y, Lee, J-L, Fong, L, et al. Pembrolizumab as Second-Line Therapy for Advanced Urothelial Carcinoma. N Engl J Med (2017) 376:1015–26. doi:10.1056/NEJMoa1613683
16. Brahmer, J, Reckamp, KL, Baas, P, Crinò, L, Eberhardt, WEE, Poddubskaya, E, et al. Nivolumab versus Docetaxel in Advanced Squamous-Cell Non-small-cell Lung Cancer. N Engl J Med (2015) 373:123–35. doi:10.1056/NEJMoa1504627
17. Mehnert, JM, Monjazeb, AM, Beerthuijzen, JMT, Collyar, D, Rubinstein, L, and Harris, LN. The Challenge for Development of Valuable Immuno-Oncology Biomarkers. Clin Cancer Res (2017) 23:4970–9. doi:10.1158/1078-0432.CCR-16-3063
18. Cristescu, R, Mogg, R, Ayers, M, Albright, A, Murphy, E, Yearley, J, et al. Pan-tumor Genomic Biomarkers for PD-1 Checkpoint Blockade-Based Immunotherapy. Science (2018) 362:eaar3593. doi:10.1126/science.aar3593
19. Wu, W, Zhou, Y, Wang, Y, Liu, L, Lou, J, Deng, Y, et al. Clinical Significance of Somatostatin Receptor (SSTR) 2 in Meningioma. Front Oncol (2020) 10:1633. doi:10.3389/fonc.2020.01633
20. Dutour, A, Kumar, U, Panetta, R, Ouafik, LH, Fina, F, Sasi, R, et al. Expression of Somatostatin Receptor Subtypes in Human Brain Tumors. Int J Cancer (1998) 76:620–7. doi:10.1002/(sici)1097-0215(19980529)76:5<620:aid-ijc2>3.0.co;2-s
21. Schulz, S, Pauli, SU, Schulz, S, Händel, M, Dietzmann, K, Firsching, R, et al. Immunohistochemical Determination of Five Somatostatin Receptors in Meningioma Reveals Frequent Overexpression of Somatostatin Receptor Subtype sst2A. Clin Cancer Res (2000) 6:1865–74.
22. Li, J, Chen, C, Bi, X, Zhou, C, Huang, T, Ni, C, et al. DNA Methylation of CMTM3 , SSTR2 , and MDFI Genes in Colorectal Cancer. Gene (2017) 630:1–7. doi:10.1016/j.gene.2017.07.082
23. Tang, LH, and Modlin, IM. Somatostatin Receptor Regulation of Gastric Carcinoid Tumours. Digestion (1996) 57(Suppl. 1):11–4. doi:10.1159/000201385
24. Yin, X, Wang, P, Yang, T, Li, G, Teng, X, Huang, W, et al. Identification of Key Modules and Genes Associated with Breast Cancer Prognosis Using WGCNA and ceRNA Network Analysis. Aging (2020) 13:2519–38. doi:10.18632/aging.202285
25. Patel, YC. Somatostatin and its Receptor Family. Front Neuroendocrinology (1999) 20:157–98. doi:10.1006/frne.1999.0183
26. Hagen, PMV, Krenning, EP, Kwekkeboom, DJ, Reubi, JC, Anker-Lugtenburg, v. PJ, Löwenberg, B, et al. Somatostatin and the Immune and Haematopoetic System; a Review. Eur J Clin Invest (1994) 24:91–9. doi:10.1111/j.1365-2362.1994.tb00972.x
27. Braun, DA, Hou, Y, Bakouny, Z, Ficial, M, Sant’ Angelo, M, Forman, J, et al. Interplay of Somatic Alterations and Immune Infiltration Modulates Response to PD-1 Blockade in Advanced clear Cell Renal Cell Carcinoma. Nat Med (2020) 26:909–18. doi:10.1038/s41591-020-0839-y
28. Gide, TN, Quek, C, Menzies, AM, Tasker, AT, Shang, P, Holst, J, et al. Distinct Immune Cell Populations Define Response to Anti-PD-1 Monotherapy and Anti-PD-1/Anti-CTLA-4 Combined Therapy. Cancer Cell (2019) 35:238–55. doi:10.1016/j.ccell.2019.01.003
29. Mariathasan, S, Turley, SJ, Nickles, D, Castiglioni, A, Yuen, K, Wang, Y, et al. TGFβ Attenuates Tumour Response to PD-L1 Blockade by Contributing to Exclusion of T Cells. Nature (2018) 554:544–8. doi:10.1038/nature25501
30. Miao, D, Margolis, CA, Gao, W, Voss, MH, Li, W, Martini, DJ, et al. Genomic Correlates of Response to Immune Checkpoint Therapies in clear Cell Renal Cell Carcinoma. Science (2018) 359:801–6. doi:10.1126/science.aan5951
31. Nathanson, T, Ahuja, A, Rubinsteyn, A, Aksoy, BA, Hellmann, MD, Miao, D, et al. Somatic Mutations and Neoepitope Homology in Melanomas Treated with CTLA-4 Blockade. Cancer Immunol Res (2017) 5:84–91. doi:10.1158/2326-6066.CIR-16-0019
32. Riaz, N, Havel, JJ, Makarov, V, Desrichard, A, Urba, WJ, Sims, JS, et al. Tumor and Microenvironment Evolution during Immunotherapy with Nivolumab. Cell (2017) 171:934–49. doi:10.1016/j.cell.2017.09.028
33. Angelova, M, Charoentong, P, Hackl, H, Fischer, ML, Snajder, R, Krogsdam, AM, et al. Characterization of the Immunophenotypes and Antigenomes of Colorectal Cancers Reveals Distinct Tumor Escape Mechanisms and Novel Targets for Immunotherapy. Genome Biol (2015) 16:64. doi:10.1186/s13059-015-0620-6
34. Charoentong, P, Finotello, F, Angelova, M, Mayer, C, Efremova, M, Rieder, D, et al. Pan-cancer Immunogenomic Analyses Reveal Genotype-Immunophenotype Relationships and Predictors of Response to Checkpoint Blockade. Cell Rep (2017) 18:248–62. doi:10.1016/j.celrep.2016.12.019
35. Subramanian, A, Tamayo, P, Mootha, VK, Mukherjee, S, Ebert, BL, Gillette, MA, et al. Gene Set Enrichment Analysis: a Knowledge-Based Approach for Interpreting Genome-wide Expression Profiles. Proc Natl Acad Sci (2005) 102:15545–50. doi:10.1073/pnas.0506580102
36. Theodoropoulou, M, and Stalla, GK. Somatostatin Receptors: from Signaling to Clinical Practice. Front Neuroendocrinology (2013) 34:228–52. doi:10.1016/j.yfrne.2013.07.005
37. Benali, N, Cordelier, P, Calise, D, Pages, P, Rochaix, P, Nagy, A, et al. Inhibition of Growth and Metastatic Progression of Pancreatic Carcinoma in Hamster after Somatostatin Receptor Subtype 2 (Sst2) Gene Expression and Administration of Cytotoxic Somatostatin Analog AN-238. Proc Natl Acad Sci (2000) 97:9180–5. doi:10.1073/pnas.130196697
38. Li, M, Fisher, WE, Kim, HJ, Wang, X, Brunicardi, CF, Chen, C, et al. Somatostatin, Somatostatin Receptors, and Pancreatic Cancer. World J Surg (2005) 29:293–6. doi:10.1007/s00268-004-7814-5
39. Buscail, L, Saint-Laurent, N, Chastre, E, Vaillant, JC, Gespach, C, Capella, G, et al. Loss of Sst2 Somatostatin Receptor Gene Expression in Human Pancreatic and Colorectal Cancer. Cancer Res (1996) 56:1823–7.
40. Carbone, DP, Reck, M, Paz-Ares, L, Creelan, B, Horn, L, Steins, M, et al. First-Line Nivolumab in Stage IV or Recurrent Non-small-cell Lung Cancer. N Engl J Med (2017) 376:2415–26. doi:10.1056/NEJMoa1613493
41. Topalian, SL, Hodi, FS, Brahmer, JR, Gettinger, SN, Smith, DC, McDermott, DF, et al. Safety, Activity, and Immune Correlates of Anti-PD-1 Antibody in Cancer. N Engl J Med (2012) 366:2443–54. doi:10.1056/NEJMoa1200690
42. McGrail, DJ, Pilié, PG, Rashid, NU, Voorwerk, L, Slagter, M, Kok, M, et al. High Tumor Mutation burden Fails to Predict Immune Checkpoint Blockade Response across All Cancer Types. Ann Oncol (2021) 32:661–72. doi:10.1016/j.annonc.2021.02.006
43. Ayers, M, Lunceford, J, Nebozhyn, M, Murphy, E, Loboda, A, Kaufman, DR, et al. IFN-γ-related mRNA Profile Predicts Clinical Response to PD-1 Blockade. J Clin Invest (2017) 127:2930–40. doi:10.1172/JCI91190
44. Ott, PA, Bang, Y-J, Piha-Paul, SA, Razak, ARA, Bennouna, J, Soria, J-C, et al. T-Cell-Inflamed Gene-Expression Profile, Programmed Death Ligand 1 Expression, and Tumor Mutational Burden Predict Efficacy in Patients Treated with Pembrolizumab across 20 Cancers: KEYNOTE-028. Jco (2019) 37:318–27. doi:10.1200/JCO.2018.78.2276
Glossary
ACC adrenocortical carcinoma
BLCA bladder urothelial carcinoma
BRCA breast invasive carcinoma
CESC cervical squamous cell carcinoma and endocervical adenocarcinoma
CHOL cholangiocarcinoma
COAD colon adenocarcinoma
DLBC lymphoid neoplasm diffuse large B-cell lymphoma
ESCA esophageal carcinoma
GBM glioblastoma multiforme
HNSC head and neck squamous cell carcinoma
KICH kidney chromophobe
KIRC kidney renal clear cell carcinoma
KIRP kidney renal papillary cell carcinoma
LAML acute myeloid leukemia
LGG brain lower grade glioma
LIHC liver hepatocellular carcinoma
LUAD lung adenocarcinoma
LUSC lung squamous cell carcinoma
MESO mesothelioma
OV ovarian serous cystadenocarcinoma
PAAD pancreatic adenocarcinoma
PCPG pheochromocytoma and paraganglioma
PRAD prostate adenocarcinoma
READ rectum adenocarcinoma
SARC sarcoma
SKCM skin cutaneous melanoma
STAD stomach adenocarcinoma
TGCT testicular germ cell tumors
THCA thyroid carcinoma
THYM thymoma
UCEC uterine Corpus Endometrial Carcinoma
UCS uterine carcinosarcoma
UVM uveal melanoma.
Keywords: bioinformatics, tumor microenvironment, immune checkpoint inhibitors, somatostatin receptor 2, predictive biomarker
Citation: Wang A, Yuan Y, Chu H, Gao Y, Jin Z, Jia Q and Zhu B (2022) Somatostatin Receptor 2: A Potential Predictive Biomarker for Immune Checkpoint Inhibitor Treatment. Pathol. Oncol. Res. 28:1610196. doi: 10.3389/pore.2022.1610196
Received: 16 November 2021; Accepted: 26 January 2022;
Published: 21 February 2022.
Edited by:
Anna Sebestyén, Semmelweis University, HungaryCopyright © 2022 Wang, Yuan, Chu, Gao, Jin, Jia and Zhu. This is an open-access article distributed under the terms of the Creative Commons Attribution License (CC BY). The use, distribution or reproduction in other forums is permitted, provided the original author(s) and the copyright owner(s) are credited and that the original publication in this journal is cited, in accordance with accepted academic practice. No use, distribution or reproduction is permitted which does not comply with these terms.
*Correspondence: Qingzhu Jia, amlhcWluZ3podTA4MDFAb3V0bG9vay5jb20=; Bo Zhu, Ym8uemh1QHRtbXUuZWR1LmNu
†These authors have contributed equally to this work