- 1Knight Cancer Institute, Oregon Health & Science University, Portland, OR, United States
- 2Cancer Early Detection Advanced Research Center, Knight Cancer Institute, Oregon Health & Science University, Portland, OR, United States
Collection and analysis of circulating tumor DNA (ctDNA) is one of the few methods of liquid biopsy that measures generalizable and tumor specific molecules, and is one of the most promising approaches in assessing the effectiveness of cancer care. Clinical assays that utilize ctDNA are commercially available for the identification of actionable mutations prior to treatment and to assess minimal residual disease after treatment. There is currently no clinical ctDNA assay specifically intended to monitor disease response during treatment, partially due to the complex challenge of understanding the biological sources of ctDNA and the underlying principles that govern its release. Although studies have shown pre- and post-treatment ctDNA levels can be prognostic, there is evidence that early, on-treatment changes in ctDNA levels are more accurate in predicting response. Yet, these results also vary widely among cohorts, cancer type, and treatment, likely due to the driving biology of tumor cell proliferation, cell death, and ctDNA clearance kinetics. To realize the full potential of ctDNA monitoring in cancer care, we may need to reorient our thinking toward the fundamental biological underpinnings of ctDNA release and dissemination from merely seeking convenient clinical correlates.
Background
Circulating tumor DNA (ctDNA) is extracellular DNA in plasma that originates from tumor cells and has emerged as a useful biomarker in non-invasive liquid biopsy (1-3). ctDNA abundance shows broad correlation with tumor burden and generally reflects the tumor DNA content such that clinical assays are commercially available for detection of molecular/minimal residual disease (MRD) and tumor mutational profiling (4–6). However, there are currently no ctDNA-based assays approved for serial monitoring during treatment to assess immediate tumor response and treatment efficacy.
Serial ctDNA monitoring during treatment can provide insight into underlying biological factors that can potentially be used to predict response, treatment efficacy, and long-term outcomes (7–11). In practice however, ctDNA levels can appear erratic across time points and are often inconsistent between patients with similar disease and treatment. This variability may be partially the result of disparate sampling frequency (often within the same study), extraction methods, and analytical approaches between studies. More likely, this variation is driven by factors that have yet to be elucidated and may vary between patients, such as individual host physiology, tumor location, tumor biology, and treatment modality.
Evidence that ctDNA concentration is more dependent on tumor cell replication rates than simply on tumor volume also suggests that understanding tumor biology and patient physiology are necessary to guide proper interpretation of ctDNA dynamics (12, 13). In some studies, early spikes in ctDNA shortly after treatment may predict a favorable clinical response, in keeping with the hypothesis that shedding is directly associated with treatment-induced tumor cell death (14–16). It is unclear however, how soon after treatment initiation this spike must occur in such cases, emphasizing the importance of collection timing. Nevertheless, early and rapid ctDNA clearance during treatment has consistently been shown to correlate with objective response and outcome (17–20). Evidence supports the idea that ctDNA release is clearly a byproduct of tumor cell proliferation, though whether this is through increased cell turnover and higher death rates or active release during cellular expansion is still an open question.
This review seeks to discuss the biological sources of variability in ctDNA abundance, with the hope that thoughtful analysis and a mechanistic understanding of ctDNA release will allow improved approaches to ctDNA interpretation in clinical response and progression.
A Note on Circulating Tumor DNA Detection and Its Implications for This Review
Typically, ctDNA is detected and characterized using methods such as droplet digital polymerase chain reaction (ddPCR) or next-generate sequencing (NGS) (reviewed by Heitzer et al. (2)) either targeting genomic positions based on a priori knowledge of tumor mutations or by calling mutations de novo at novel sites and recurrent hotspots. Although both a priori and de novo approaches to ctDNA detection assume that molecules harboring alternate alleles are tumor-derived, the later approach allows for ctDNA detection without a priori knowledge of tumor-specific mutations but is subject to much more uncertainty. The detection of tumor-derived copy-number aberrations in cell-free DNA is also possible and is dependent solely on read counts to detect gains or losses found in tumor cells (21). De novo mutations can be called from whole-genome and whole-exome sequencing, or smaller panels targeting just a few sites or genes known to harbor recurrent mutations. Panels intended to detect mutations at these canonical sites are often less informative about passenger mutations, secondary drivers, and subclonal populations. The same may be true for tumor-informed and patient-specific panels depending on the breadth of the panel used and the sampling bias of the original tumor tissue used to design the panel, particularly in the case of high intratumoral heterogeneity. These various approaches further confound our ability to compare results across studies, patient populations, and cancers. This problem is particularly true for studies where ctDNA was characterized by the prevalence of a single mutation in a single gene, where subclonal populations driven by other genetic aberrations may be under selective pressure during treatment. Of course, with a broader analytical space comes greater cost and complexity and additional challenges for implementation of accurate ctDNA assays in a clinical setting. For example, a simple ddPCR or amplicon-sequencing test to detect the presence of low-abundance EGFR mutations in the cell-free DNA of lung cancer patients is much cheaper and simpler to validate and execute in a diagnostic laboratory than a whole-exome or a multi-gene sequencing panel with similar accuracy and sensitivity. However, such an assay may not be representative of the entire tumor cell population, particularly if there are treatment-resistant subclones that harbor distinct genotypes. Consequently, careful evaluation of single-target vs. multi-target approaches is necessary.
Although the data available to assess ctDNA abundance as it relates to clinical observations, treatment response, and outcome consist primarily of mutant-allele detection and prevalence estimates, evidence suggests approaches like methylation profiling by whole-genome or targeted bisulfite sequencing may be more sensitive and are not dependent on the presence of genetic aberrations (5). A serial comparison of single-nucleotide variants (SNVs) and methylation profiles in EGFR T790M-positive advanced cancer patients found that methylation levels closely followed SNV mutant allele frequency and both were predictive of long-term treatment response (22). Silva et al. (23) reported changes in cell-free DNA methylation over time that were associated with therapy response and progression in prostate cancer patients. Few studies, however, have assessed methylation dynamics in cell-free DNA with high-frequency sample collection during early phases of treatment, and therefore the data presented here are biased toward somatic mutations as a means of ctDNA detection and characterization. It remains to be seen how broad the search space needs to be in order to effectively monitor tumor cell populations by ctDNA, and which reporter (i.e., mutations, methylation, etc.) will be the most informative, but may vary by patient, tumor, and treatment.
Biological Factors That Most Affect Circulating Tumor DNA Abundance
In order to utilize ctDNA monitoring during treatment we must understand the various factors that impact ctDNA concentration over time. Multiple sources of ctDNA have been suggested including apoptosis, necrosis, and so called “active/passive release” (reviewed by Aucamp et al. (24)). Apoptosis, necrosis, and other forms of tumor cell death result in ctDNA release into interstitial space where it moves to the lymphatic system and blood circulation (8, 25). It is also hypothesized that extracellular DNA can be released from living cells in various contexts that are both energy-dependent and independent, and range in mechanism from shedding of mis-segregated DNA to intercellular signaling (26). Once ctDNA enters circulation, it is subject to further degradation by DNases in the blood and is putatively removed by the liver, spleen, and/or kidneys within 30–120 min (27). Changes in the balance of these processes due to treatment are assumed to be reflected in ctDNA dynamics, which, if correctly interpreted, may inform us about a patient’s disease state and response to therapy (Figure 1). Furthermore, disruption of biological homeostasis resulting from disease and treatment can increase overall levels of cell-free DNA, decreasing the relative abundance of ctDNA and thus impacting assay sensitivity. This can also make interpreting data from studies that simply report mutant allele frequency challenging without accounting for such changes in total cell-free DNA. The following sections address how the unique biology of a cancer and host physiology influences ctDNA abundance in the blood stream.
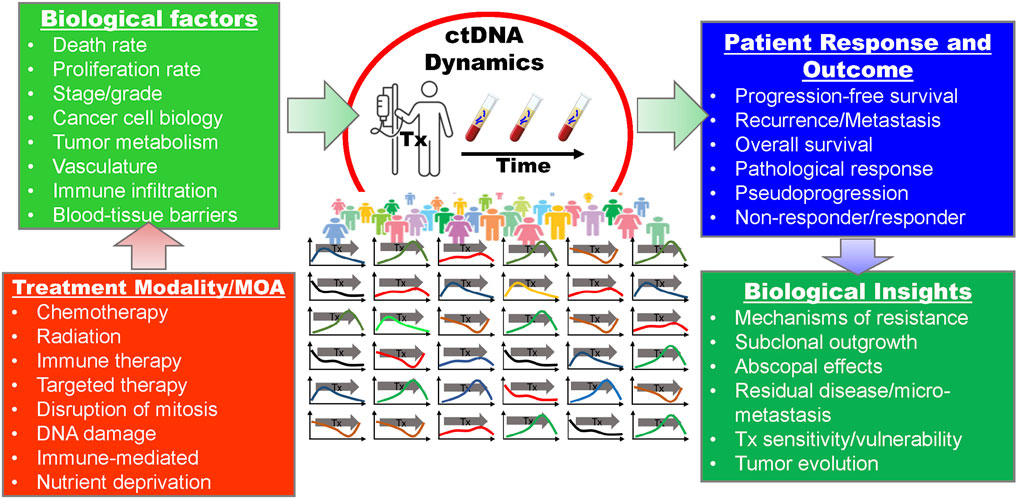
FIGURE 1. The relationships between treatment, biological factors, clinical indicators and outcomes, and potential insights with regard to serial ctDNA monitoring during treatment. MOA, mechanism of action; Tx, treatment.
Cancer Type and Biology
Even before our ability to distinguish ctDNA molecules amongst cell-free DNA, there appeared to be a clear relationship between cell-free DNA abundance and disease state. The more severe the patient’s cancer, the more cell-free DNA present in their blood (reviewed by de Miranda et al. (28), Grabuschnig et al. (26), Aucamp et al. (24)), suggesting that disease burden impacts cell-free DNA homeostasis. Later work has shown that ctDNA levels, independent of non-tumor, cell-free DNA, vary dramatically across disease type, tumor location, and stage (29–33). In vitro assays have helped isolate the mechanistic variables involved in cell-free DNA release, particularly with regard to known apoptotic and necrotic processes. These experiments found that cell-free DNA release can vary significantly between cell-lines with different phenotypes and histologies (34–39).
Observations in human cohorts also correlate ctDNA with tumor histology, grade, and stage. Various studies in neoadjuvantly-treated breast cancer patients found that ctDNA levels and mutations were significantly different between breast cancer subtypes (9, 11, 40, 41). Expression levels of the proliferation-associated nuclear protein, Ki-67, have also been directly associated with ctDNA characteristics in breast and lung cancers (42–44). Studies assessing ctDNA in non-small cell lung cancer (NSCLC) patients found that ctDNA concentration was correlated with tumor stage, histology, and degree of cytological atypia (45–47).
Tumor Volume, Growth Rate, and Metabolism
Cell death has historically been considered the largest contributor to ctDNA and tumor volume the most reliable predictor of ctDNA abundance. More recently, various mechanisms and conditions have been proposed in which living tumor cells, particularly during mitosis, could shed DNA in both an energy-dependent and independent manner (26, 34). Therefore, it is likely the complex interplay between tumor cell proliferation and death that determines ctDNA abundance. This relationship has significant implications for how we should think about ctDNA measurements in the context of tumor volume, growth rate, and metabolism. For example, one can easily imagine a scenario where proliferation and death rates both increase but are in balance resulting in increased ctDNA shedding but no net change in tumor volume (48). Furthermore, although ctDNA may be hypothetically representative of the entire cancer cell population, it is likely subject to significant composition bias from differential cell turnover rates across subclones (3). Examining these variables in vitro and in vivo can shed light on which processes contribute more to ctDNA abundance.
Tumor growth rate and metabolism are often inferred by measuring tumor glucose uptake. Studies in metastatic melanoma patients found a strong correlation between the tumor PET avidity (a measure of cellular glucose uptake) and ctDNA abundance, independent of tumor volume (49). These results are supported by studies in resected NSCLC, where a correlation was found between increased mitotic rates and higher ctDNA levels measured 24 h prior to surgery, as well as increased levels of the proliferation marker Ki-67 (42–44, 47). Indeed, in vitro studies have consistently found that large amounts of cell-free DNA can accumulate in the media of actively proliferating cell populations independent of apoptosis or necrosis (34, 50–53). DNA fragments resulting from mis-segregation events during mitosis were found to be released by actively proliferating cancer cells via the creation of micronuclei (54, 55), but their relative contribution to overall ctDNA abundance in vivo remains unclear. Similar to the DNA products of necrosis, it has been assumed that these fragments would appear distinct from apoptotic ctDNA given their larger size and that their contribution would therefore be obvious. However, evidence is emerging that cleavage of larger DNA fragments by extracellular DNases may also occur (51). These DNA fragments might have an apoptotic fragmentation pattern, yet be generated by non-apoptotic mechanisms, such as release during proliferative states.
The relationship between tumor cell proliferation and death is not independent. In healthy tissues, cell density homeostasis is achieved by compensating for cell death with an appropriate rate of cell proliferation. This process is known as “apoptosis-induced proliferation” (reviewed by Heitzer et al. (2) and Ryoo et al. (56)), but it is unclear how significant a role it plays in tumors. The consequences for ctDNA shedding could be straightforward, where increased cell death leads to increase cell birth and so on, and both processes result in increased ctDNA levels. However, positive feedback mechanisms like this may be tissue-dependent and could be dysregulated in cancer, complicating interpretation of ctDNA dynamics.
Tumor Vasculature, Blood Vessel Proximity, and Hypoxia
Tumor vascularization and proximity to major blood vessels are also features of tumor physiology that might be expected to significantly impact ctDNA levels. Blood flow to a tumor is the direct means by which ctDNA enters circulation and it affects the metabolic activity of a tumor by providing oxygen and nutrients (57). As a tumor grows its vasculature becomes more irregular and dysfunctional leading to reduced oxygen levels, hypoxia and necrosis. Necrosis, reduced nutrient levels, and limited accesses to wider blood circulation all potentially effect ctDNA abundance in unique ways. Vasculature can also impact drug delivery and efficacy, which may also affect ctDNA shedding. It is unclear how much ctDNA abundance is dependent on direct access to blood vessels. Interstitial ctDNA is assumed to passively enter circulation through nearby blood vessels, but other processes like macrophage clearance of dead and dying cells (see “Immune Response” and “Immunotherapy” sections below) may also play a role in transporting ctDNA from areas with poor vasculature to the bloodstream (8, 25, 27).
Results from studies directly comparing tumor vascularization, angiogenesis, and ctDNA abundance, are inconsistent between studies. Post-excision pathology by Abbosh et al. (4) found lymphovascular invasion to be predictive of ctDNA detection in early-stage lung cancer. Two other studies in lung cancer found vascular invasion to be marginally (47), or not at all (46), correlated with ctDNA detection. Interestingly, when only looking at patients with EGFR mutations, ctDNA was significantly correlated with vascular invasion in the former study by Cho et al. (47). In liver cancer, microvascular invasion was correlated with preoperative ctDNA levels (58). In recent preliminary data collected from a large cohort of colorectal cancer (CRC) patients, ctDNA was found to be strongly associated with lymphovascular invasion (59). In neurological malignancies, which typically have less detectable ctDNA, Nabavizadeh et al. (60) found that tumor vessel size was correlated with detectable ctDNA. Notably, previous work by the same group and others found that the amount of microvascular proliferation was not significantly correlated to ctDNA in glioblastoma (GBM) specifically (61, 62). The nature of such studies makes it challenging to discern if these correlations are independent of tumor stage and volume. Proving a causal link may only be possible with further evaluation of preclinical models, tumor pathology, and imaging.
Hypoxia is in many ways a measure of tumor cell access to functional vasculature (63). As a tumor grows, cells become more isolated from functional vasculature, despite upregulated angiogenesis that is characteristic of many cancers. This process selects for cells that are more tolerant of low-oxygen conditions while the remaining population become necrotic (64). There is a clear link between hypoxia and necrosis and some studies have suggested that ctDNA is primarily derived from necrotic processes (1, 35, 65, 66). This suggests that as a tumor grows and vasculature becomes more distant and dysfunctional, wider ctDNA abundance could either increase due to further necrosis, or decrease due to reduced access to that vasculature. Since both forces are not equal in all tumors, the overall effect on ctDNA levels from this process may not be neutral. In vitro experiments with CRC cells have found that hypoxic conditions induced cell-free DNA production during the first 24 h but decreased dramatically over the following 48–72 h (37). These results are also consistent with previous findings in both tumor-injected and tumor-free mice where hypoxia induced cell-free DNA release (67). Deprivation of the metabolite, folate, has been found to induced double-strand DNA breaks and mis-segregation events, which may also lead to ctDNA shedding in nutrient-starved tumors as well (68).
Organ Encapsulation
The free movement of cell-free DNA between tissue and blood may be restricted in some organs. Blood-tissue barriers have been identified throughout the body, such as the thymus, testes, retina, and intestines, but it is unclear what role they might play in cell-free DNA exchange (69). The blood-brain barrier (BBB) is often cited as the primary reason that neurological malignancies, particularly gliomas, produce less detectable ctDNA than other cancer types (29). In a 2018 review on ctDNA kinetics, Khier and Lohan speculate that physiological barriers, like the BBB, restrict the movement of cell-free DNA throughout the body while also acknowledging the exception of placental cell-free DNA, which has been shown to move quite freely throughout the mother (8, 70). Notably, disruption of the BBB that results in increased permeability and risk of metastasis also resulted in increased levels of ctDNA in patients with GBM (60). Several studies have shown that disrupting the BBB in animal models using focused ultrasound techniques leads to increase blood levels of ctDNA and other biomarkers (71, 72). Therefore, it is possible that changes in tissue-blood barrier permeability, particularly during treatment, might significantly affect ctDNA dynamics.
Immune Response
Although there may be a significant role for inflammation and infection (e.g., sepsis) in cell-free DNA release, this review is primarily interested in the extent to which they directly impact ctDNA release from cancer cells. Early studies exploring the origins of cell-free DNA found that macrophages may play a significant role in cell-free DNA release though phagocytosis of dead and dying cells (73). Phagocytes have been shown to digest apoptotic cells and release the resulting cell debris and fragmented DNA (24, 73, 74). In the GBM study mentioned earlier, ctDNA levels were strongly associated with the density of macrophages around the tumor (60). In healthy individuals cell turnover is a tightly regulated process where apoptotic cells are quickly removed by phagocytes, however, this process appears to be dysfunctional in tumors resulting in excess cell debris (including DNA) that accumulates locally and in circulation (27, 66). The extent to which ctDNA levels might be directly affected by tumor cell targeting and/or clearance by immune cells is still an open question. Evidence for this phenomenon however, might be found in studies where ctDNA levels spike within 2 weeks of immune-therapy initiation in metastatic melanoma patients, if and only if, the tumors were responsive (75).
Cell-Free DNA Clearance
Cell-free DNA digestion and clearance, whether achieved locally via phagocytosis or in circulation via the liver, spleen, and kidneys, is influenced by a number of factors (8, 27, 76, 77). As described above, cell-free DNA clearance in situ is potentially dependent on interstitial diffusion and the presence of phagocytic cells, however, once it is in circulation its half-life is determined by extracellular DNase activity and organ function (8). ctDNA half-life in the blood ranges from 30 to 120 min (27) making blood collection timing critical. The decreased levels of DNase activity observed in the blood of cancer patients potentially explains the accompanying increase in cell-free DNA levels from disruption of homeostasis (78, 79). Studies have also suggested that cell-free DNA clearance and half-life is dependent on proper liver and kidney function suggesting that treatment toxicity in cancer patients could affect ctDNA clearance rates and abundance (80, 81). The role of renal function in ctDNA clearance is not well understood, however, based on experiments assessing cell-free DNA levels in urine (27). The presence of cell-free DNA in urine implies involvement of the kidneys in clearance from circulation, however, patients with chronic renal failure were found not to have increased levels of cell-free DNA in their plasma (82). Methylation profiling has suggested that cell-free DNA present in urine is derived from white blood cells, kidney cells and urinary tract cells, but data from stem cell transplant patients found that the majority of this DNA was from the renal system itself and not plasma (83, 84).
The Effect of Treatment on Circulating Tumor DNA Abundance
The effect of treatment on tumor cell proliferation and death, and thus ctDNA dynamics, is dependent on its mechanism of action, efficacy, and tumor biology. Considering the factors described above that influence ctDNA abundance, it is not surprising that there are many discernable differences between the ctDNA dynamics of responders and non-responders during treatment. Predicting their timing and trajectories is not so simple, particularly when considering the short half-life of ctDNA. We might at least expect that ctDNA dynamics should reflect treatment response depending on the mechanism of action of a given treatment, but the timing of those effects is still unclear (Figure 2). The correlation between in vitro and in vivo models of treatment-induced cell death remains largely unclear, and is likely dependent on a variety of factors including the treatment and tissue of interest. Despite the paucity of data, it is possible that tumor cell death can occur within hours of treatment and therefore ctDNA levels may rapidly increase as well (36, 85–87). Serial ctDNA monitoring has been done with collection times ranging from minutes, hours or days after administration of treatment, to weeks and months. Some guidance may be gleaned from these studies, but the optimal time for sampling may be unique to cancer type and therapy and may need to be determined empirically. The following sections outline the expected effects of cancer treatment modalities on ctDNA dynamics, and what existing evidence, if any, tells about these hypotheses.
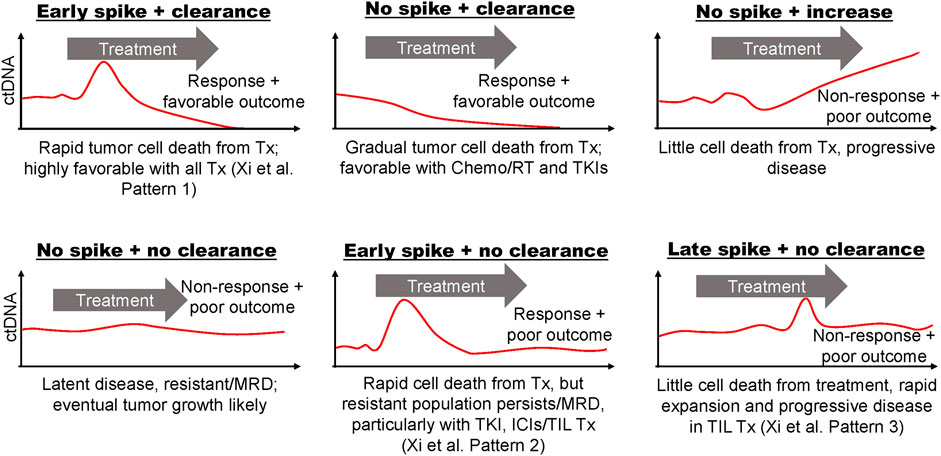
FIGURE 2. Hypothetical ctDNA dynamics from blood sampled frequently before, during, and after treatment. Characteristic ctDNA dynamics are depicted as observed in various studies throughout the text or otherwise hypothesized. The speculated driving tumor biology is described for each plot and example outcomes are stated. Response patterns defined by Xi et al. (14) for metastatic melanoma patients undergoing tumor infiltrating lymphocyte (TIL) immunotherapy are indicated when relevant. Tx, treatment; TKI, tyrosine kinase inhibitors; ICI, immune checkpoint inhibitors; MRD, molecular/minimal residual disease; ChemoRT, chemoradiation therapy.
Chemotherapy and Radiation
Cytotoxic chemotherapies and radiation therapy (RT) are often used independently or in combination as first-line treatment in many cancers. Chemotherapy agents function by disrupting mitosis or causing DNA damage leading to cell-cycle arrest, mitotic catastrophe, and apoptosis. Radiation therapy kills cells by DNA damage as well, but it also elicits an immune response and vascular damage, which can result in subsequent rounds of tumor cell death. Mitotic arrests and DNA damage are thought to cause tumor cell death within 6–72 h of administration in vivo, so detecting ctDNA shedding in response to effective treatment may require immediate sampling (87). Unfortunately, very few studies sample ctDNA within the first 72 h of chemotherapy. It has also been suggested that treatment-induced mitotic catastrophe can cause delays in cell death from chemotherapy and RT for up to a week (36, 62, 88). These various mechanisms of action may result in multiple shedding events, where one may be more informative about treatment response over another.
Limited studies assessing ctDNA levels immediately after treatment are conflicting. In castration-resistant prostate cancer patients receiving docetaxel-based therapy, early ctDNA levels were found to increase rapidly within 1 h of administration with a corresponding decrease in total cell-free DNA (89). This observation is consistent with increased tumor cell sensitivity to cytotoxic agents compared to healthy tissue. Contrary to these findings, however, CRC patients receiving FOLFOX did not exhibit a spike in ctDNA at any point within the first 48 h of treatment, despite high-resolution sampling at 3, 9, 18, 23, 26, 42, and 47 h (90). Another study involving metastatic CRC patients receiving FOLFIRI looked at ctDNA levels before and 7 days after each of the first two treatment cycles and again at progression (91). Interestingly, this study found that temporary increases in ctDNA while on treatment were predictive of progressive disease and worse survival rates, and suggested ctDNA monitoring within the first week of treatment to evaluate treatment efficacy. While ctDNA levels were decreased at the time of radiological assessment compared to baseline for all patient, patients with temporary spikes in ctDNA appeared to have more sustained ctDNA burden than those with favorable response (see Figure 2: “Early spike + no clearance”). Clonal composition was also found to vary during treatment suggesting a differential response to treatment among tumor cell subpopulations. Increases in cell-free DNA methylation levels of tumor suppressor genes (APC and RASSF1A) 24 h after receiving cisplatin-based chemotherapy were correlated with improved tumor response and overall outcome in advanced lung cancer patients (92). The same study showed that methylation levels of those genes in lung cancer cells also peaked 24 h after cisplatin exposure, however, it is unclear if the hypermethylated DNA was tumor-derived in patients. Notably, this study also found that elevated methylation of APC and/or RASSF1A in tumor-bearing mice were associated with tumor cell death as determined by biopsy shortly after treatment and blood collection. This finding might suggest that the methylated cell-free DNA originated from these dying tumor cells. In pancreatic cancer patients sampled for 4 weeks following treatment with gemcitabine, decreases in ctDNA were correlated with tumor response (93). In our work and others’, decreases in or complete clearance of ctDNA levels during low-resolution sampling (i.e., weeks to months) of neoadjuvantly-treated breast cancer patients were associated with pathological complete response at the time of surgery (9–11).
In patient cohorts receiving combination chemoradiation therapy (CRT), decrease and clearance of ctDNA after 3–4 weeks was associated with tumor response in oropharyngeal and lung cancer (94, 95). Studies employing high-frequency sampling at time points within hours of treatment are sparse, however, recent data from Breadner et al. (96) found that ctDNA abundance increased in 77% of stage III/IV non-small cell lung cancer patients shortly after receiving CRT with peak abundance observed 7 h after chemotherapy initiation and 2 days after the first fraction of radiation. Early spikes in ctDNA were seen in some patients receiving CRT for treatment of locally advanced head and neck cancer, but were not correlated with response (16). Rather, overall decreases in ctDNA at later time points, which were not unique to patients who had early peaks, were more predictive of outcome.
Some of the earliest observations of cell-free DNA by Leon et al. (97) occurred in patients receiving RT alone, finding that general cell-free DNA levels decreased after treatment. Aucamp et al. (24) speculate that the reason for this may have been the coincident destruction of phagocytes needed to generate cell-free DNA. Another consideration that may confound ctDNA measurement from irradiated tumors is that, although mitotic arrest and catastrophe are the primary means by which RT is thought to kill cancer cells, they have also been found to result in mis-segregation events (98, 99). As discussed above, this process can result in releasing of DNA from living cells, providing another potential source of ctDNA that does not coincide with cell death. A recent study found that irradiation of head and neck cancer and NSCLC cell lines induced cell-free DNA shedding after 6–24 h in culture (36). The same study found that ctDNA levels increased within 24 h and peaked 96–144 h after 20 Gy of irradiation in xenograft mouse models. Interestingly, the authors found that treatment-induced senescence that was overcome with the senolytic drug, Navitoclax, lead to apoptosis and increased ctDNA release. In human subjects, investigation of RT alone in NSCLC has shown mixed results. Walls et al. (100) found that 3 of 5 patients had decreased ctDNA levels 3-day after their first RT fraction, while the remaining 2 had increased levels of some tumor-derived variants, but not others. Preliminary data from our lab (101) and another study from Chen et al. (102) found that ctDNA levels were elevated 24–48 h after the first dose of stereotactic ablative radiotherapy. These three studies in NSCLC patients used varying doses of radiation per fraction (2.75, 12, and ∼12.5 Gy, respectively), which along with sampling time differences, may account for the discrepancy. Chaudhuri et al. (103) also reported that mid-RT ctDNA levels in 13 NSCLC patients were correlated with outcomes at 2 years. A recently published study by our lab found that a metastatic breast cancer patient had increased ctDNA levels while receiving palliative radiation therapy. Deep sequencing using a 53-mutation panel representative of both clonal and subclonal mutations, which were previously identified from WES of multiple tumors, revealed differential response in ctDNA levels for various subclones with sample collection every 48-hour during RT (104). Differential response in subclonal ctDNA abundance is suggestive of varying sensitivity in irradiated tumor cell subpopulations and/or an abscopal response. First observed by Dr. R.H. Mole in 1953, the abscopal effect is the shrinkage of a distant, untreated tumor in response to RT of another tumor. It is thought that the destruction of cells in the irradiated tumor elicits an immune response that affects the non-radiated tumors elsewhere in the body (105). In our case, radiation of a single lesion may have induced immune-mediated responses and ctDNA shedding from distant metastatic sites that harbored subclonal tumor cell populations. It is also worth noting that CTCs may also be sources of ctDNA and CTC release timing may also be similar to tumor ctDNA shedding (106). In preliminary data in head and neck cancer patients treated with RT, 3 of 11 patients had increased circulating tumor cell (CTC) counts after the first fraction of RT, and 5 of 6 patients had increases CTCs after 2 weeks into therapy.(107).
Immunotherapy
ctDNA monitoring during treatment with immune checkpoint inhibitors (ICIs) has shown promise in multiple cancer types. A pan-cancer analysis done by Zhang et al. (17) found that changes in ctDNA levels during ICI treatment may be predictive of benefit. Patients in this study with increases in ctDNA levels during treatment had worse outcomes as compared to those that did not. Furthermore, patients with ctDNA clearance after detectable pre-treatment levels had the best progression-free and overall survival. A recent study by Herbretreau et al. (75) in patients with metastatic melanoma found that significant increases in ctDNA levels during the first 2–4 weeks of anti-PD1 (with or without anti-CTLA4) allowed early and highly-specific identification of treatment-resistant patients. Furthermore, ctDNA levels that rapidly decreased after starting PD-1 inhibitors were highly predictive of responses consistent with pseudoprogression (108, 109). When compared to changes in ctDNA levels later in treatment, regardless of early changes, increases beyond 12 weeks were not necessarily predictive of non-response, further suggesting that early sampling is more informative (110). In NSCLC patients treated with ICI, decreases in ctDNA 2 weeks after treatment initiation were strongly correlated with radiographic response and progression-free survival (111). A study investigating early response to tumor infiltrating lymphocyte (TIL) immunotherapy in metastatic melanoma patients identified three patterns of ctDNA dynamics that could be used to stratify patients by overall survival (14). Patients with an early spike in ctDNA within 5–10 days of treatment followed by clearance showed a statistically significant survival outcome over patients who had early peaks but latent ctDNA burden, or no peaks with or without clearing (see Figure 2). The study’s authors speculate that early spike it ctDNA was in part due to the newly-transferred lymphocytes “identifying their targets and are effective in killing [them].”
Targeted Therapy
Given the clinical implications of tumor heterogeneity, one of the most significant unanswered questions in ctDNA analysis is whether ctDNA observed during therapy is more representative of resistant or responsive tumor cell populations. In a study of lung cancer patients undergoing EGFR tyrosine kinase inhibitor (TKI) therapy, ctDNA sampled 2 weeks after treatment initiation revealed activating mutations not previously detected in the tumor biopsies (112). Another study of lung cancer patients on TKIs found that clearing of ctDNA within days of treatment was associated with response, whereas sudden increases in ctDNA load later in treatment correlated with rapid tumor progression and poor outcome (113). In lung cancer patients receiving either anti-EGFR or HER2 therapies, increases in ctDNA abundance were observed within 4–12 h after initiation of treatment while total cell-free DNA was relatively constant (86). Phallen and coauthors point out that this timeframe is consistent with other studies in which apoptosis is observed in vitro within 6–48 h of treatment with EGFR TKIs. Patients in this study with an initial radiographic response all had ctDNA abundance eventually decrease by more than 95% within the first 19 days of treatment. Interestingly, baseline levels in a study of ALK-fusion positive lung cancer patients, pre-treatment ctDNA levels were not correlated with treatment response, yet changes in ctDNA during treatment with ALK TKIs were associated with progression (114).
Increases in ctDNA abundance of therapy-sensitive clones corresponds with response, however, increases in ctDNA abundance of therapy-resistant clones can also portend clinical progression (2). Outgrowth of subclonal tumor cell populations that are resistant to targeted therapy can be directly observed in allele-specific ctDNA dynamics. For example, genomic changes conferring resistance to targeted therapy in prostate cancer patients were detected by increasing fractions of the resistance-associated allele in several studies (115, 116).
Discussion
Host physiology and tumor biology affect ctDNA abundance while changes in ctDNA levels during treatment may indicate disease response. Cancer type and stage appear to have the most dramatic impact on ctDNA abundance, and significant decreases in, or clearance of ctDNA early in treatment seems to be predictive of response and improved outcomes. The association of treatment response and overall decreases in ctDNA levels during treatment is consistent with the hypothesis that tumor burden and tumor growth rate are reflected in ctDNA dynamics. Very early changes (1–3 h) in ctDNA levels have been hypothesized to reflect treatment response as well, but this appears to be less generalizable. For example, we might expect effective chemotherapy to induce ctDNA shedding immediately, and early, high-frequency sampling to detect it, yet observations between NSCLC, CRC and prostate cancer patients sampled within 1–3 h of chemotherapy were inconsistent (89, 90, 117). Unfortunately, there are a lack of studies sampling within this timeframe. Immunotherapy may be less fast-acting than chemotherapy given the time required for the body to prepare a successful immune response. PET/CT imaging has shown tumor responses with 4–6 weeks of treatment with ICIs in melanoma patients (118). Sample collection at 2 weeks following treatment found changes in ctDNA that correlated with outcome in ICI therapy of melanoma patients, but earlier time points were not collected (75). It possible that changes in ctDNA in response to treatment existed sooner, again, earlier, high-frequency sampling is needed to test such hypotheses. TKI-induced cell death appears to occur within 6–48 h of exposure in vitro (85); a similar timeframe as cell death from cytotoxic agents. Evidence presented in this review suggests that ctDNA dynamics might reflect TKI-induced cell death in this timeframe in NSCLC patients more consistently than during chemotherapy.
One potential use of ctDNA monitoring during treatment that has been explored by our lab and others (96, 101), is to induce ctDNA shedding from either inaccessible tumors or suspicious lesions for evaluation. Radiation treatment seems particularly suited for this task, however any method of perturbation that elicits ctDNA shedding could be used. For example, such approaches could improve the detection rates of ctDNA assays like CAPP-Seq and Lung-CLiP (45) in lung cancer patients or where low-dose CT is already in use for screening high-risk populations. Compression of breast tissue during mammography has been shown to temporarily increase ctDNA abundance, which could be leveraged for non-invasive biopsy or early detection (44). Other work has explored the use of ultrasound to elicit better movement of blood biomarkers across the BBB in preclinical brain tumor models (71, 72). Again, a clear understanding of early ctDNA dynamics in response to tumor perturbations is crucial before such approaches can be implemented in the clinic.
Variability in ctDNA measurements between patients and studies has been a challenge for serial monitoring. Efforts have been made to assess the biological variability of both cell-free DNA and ctDNA between measurements taken over short intervals (31, 119). Several groups have attempted to standardize criteria for evaluating differences between pre-treatment and on-treatment ctDNA levels. O’Leary et al. (120) created a “circulating tumor DNA ratio” or CDR, defined simply as the ratio of on-treatment to pre-treatment levels, to evaluate treatment response in metastatic breast cancer. Herbreteau, Kruger et al. (2018 and 2021) (75, 93) defined a “quantitative biological response and progression criteria” where patients were stratified by increases or decreases in ctDNA during treatment as compared to baseline. This approach also recognized the variability in accuracy at each time point when evaluating significance between measurements at different time points. Out of similar concern, our lab has also developed a Bayesian approach for testing statistical significance between ctDNA mutations in NGS data from serial collections (10). Given the variability in coverage of NGS data, which determines the limit of detection, the allele-specific background error rate, and the stochastic nature of mutant read detection by NGS, sophisticated methods may be required to account for these uncertainties in ctDNA evaluation.
Finally, attempts to model ctDNA shedding have shown promise in predicting ctDNA abundance based on tumor size and growth rate. Avanzini et al. (48) presented a stochastic mathematical framework based on observations in lung cancer patients which could extrapolate ctDNA copy counts using tumor cell proliferation rate, death rate, shedding probability, clearance rate, and starting tumor volume. Such modeling can reveal unexpected behavior that may be informative of real-world scenarios. For example, in simulations the authors unexpectedly found that a slow growing cancer generated more ctDNA molecules than a faster-growing cancer of the same size when the faster growth was achieved by proportional increases and decreases in birth and death rates, respectively. However, if a faster growth rate is achieved by increased birth rate and a stable death rate, the difference in ctDNA release was negligible. By integrating these variables over time, similar models might be useful in predicting changes in ctDNA abundance from changes in birth and death rates resulting from treatment.
Conclusion
The utilization of ctDNA in assessing treatment response will require a better understanding of the biological factors involved. We believe that ctDNA monitoring has the potential to truly revolutionize personal medicine in cancer care but there remain significant challenges that must first be overcome.
Author Contributions
CB prepared and edited the text and figures; PS edited the text and figures.
Conflict of Interest
PS has consulted for Natera.
The remaining author declares that the research was conducted in the absence of any commercial or financial relationships that could be construed as a potential conflict of interest.
References
1. Wan, JCM, Massie, C, Garcia-Corbacho, J, Mouliere, F, Brenton, JD, Caldas, C, et al. Liquid Biopsies Come of Age: towards Implementation of Circulating Tumour DNA. Nat Rev Cancer (2017) 17(4):223–38. doi:10.1038/nrc.2017.7
2. Heitzer, E, Auinger, L, and Speicher, MR. Cell-Free DNA and Apoptosis: How Dead Cells Inform about the Living. Trends Mol Med (2020) 26(5):519–28. doi:10.1016/j.molmed.2020.01.012
3. Merker, JD, Oxnard, GR, Compton, C, Diehn, M, Hurley, P, Lazar, AJ, et al. Circulating Tumor DNA Analysis in Patients with Cancer: American Society of Clinical Oncology and College of American Pathologists Joint Review. J Clin Oncol (2018) 36(16):1631–41. doi:10.1200/jco.2017.76.8671
4. Abbosh, C, Birkbak, NJ, Wilson, GA, Jamal-Hanjani, M, Constantin, T, Salari, R, et al. Phylogenetic ctDNA Analysis Depicts Early-Stage Lung Cancer Evolution. Nature (2017) 545(7655):446–51. doi:10.1038/nature22364
5. Keller, L, Belloum, Y, Wikman, H, and Pantel, K. Clinical Relevance of Blood-Based ctDNA Analysis: Mutation Detection and beyond. Br J Cancer (2021) 124(2):345–58. doi:10.1038/s41416-020-01047-5
6. Adashek, JJ, Janku, F, and Kurzrock, R. Signed in Blood: Circulating Tumor DNA in Cancer Diagnosis, Treatment and Screening. Cancers (Basel) (2021) 13(14), 3600. doi:10.3390/cancers13143600
7. De Michino, S, Aparnathi, M, Rostami, A, Lok, BH, and Bratman, SV. The Utility of Liquid Biopsies in Radiation Oncology. Int J Radiat Oncol Biol Phys (2020) 107(5):873–86. doi:10.1016/j.ijrobp.2020.05.008
8. Khier, S, and Lohan, L. Kinetics of Circulating Cell–free DNA for Biomedical Applications: Critical Appraisal of the Literature. Future Sci OA (2018) 4(4):FSO295. doi:10.4155/fsoa-2017-0140
9. Butler, TM, Boniface, CT, Johnson-Camacho, K, Tabatabaei, S, Melendez, D, Kelley, T, et al. Circulating Tumor DNA Dynamics Using Patient-Customized Assays are Associated with Outcome in Neoadjuvantly Treated Breast Cancer. Cold Spring Harb Mol Case Stud (2019) 5(2):a003772. doi:10.1101/mcs.a003772
10. Boniface, C, Deig, C, Halsey, C, Kelley, T, Heskett, MB, Thomas, CR, et al. The Feasibility of Patient-specific Circulating Tumor DNA Monitoring throughout Multi-Modality Therapy for Locally Advanced Esophageal and Rectal Cancer: A Potential Biomarker for Early Detection of Subclinical Disease. Diagnostics (Basel) (2021) 11(1):73. doi:10.3390/diagnostics11010073
11. Magbanua, MJM, Swigart, LB, Wu, H-T, Hirst, GL, Yau, C, Wolf, DM, et al. Circulating Tumor DNA in Neoadjuvant-Treated Breast Cancer Reflects Response and Survival. Ann Oncol (2021) 32(2):229–39. doi:10.1016/j.annonc.2020.11.007
12. Morbelli, S, Alama, A, Ferrarazzo, G, Coco, S, Genova, C, Rijavec, E, et al. Circulating Tumor DNA Reflects Tumor Metabolism rather Than Tumor Burden in Chemotherapy-Naive Patients with Advanced Non-small Cell Lung Cancer: 18F-FDG PET/CT Study. J Nucl Med (2017) 58(11):1764–9. doi:10.2967/jnumed.117.193201
13. García-Olmo, DC, Samos, J, Picazo, MG, Asensio, AI, Toboso, I, and García-Olmo, D. Release of Cell-free DNA into the Bloodstream Leads to High Levels of Non-tumor Plasma DNA during Tumor Progression in Rats. Cancer Lett (2008) 272(1):133–40. doi:10.1016/j.canlet.2008.07.003
14. Xi, L, Pham, TH-T, Payabyab, EC, Sherry, RM, Rosenberg, SA, and Raffeld, M. Circulating Tumor DNA as an Early Indicator of Response to T-Cell Transfer Immunotherapy in Metastatic Melanoma. Clin Cancer Res (2016) 22(22):5480–6. doi:10.1158/1078-0432.ccr-16-0613
15. Lo, YM, Leung, SF, Chan, LY, Chan, AT, Lo, KW, Johnson, PJ, et al. Kinetics of Plasma Epstein-Barr Virus DNA during Radiation Therapy for Nasopharyngeal Carcinoma. Cancer Res (2000) 60(9):2351–5.
16. Hilke, FJ, Muyas, F, Admard, J, Kootz, B, Nann, D, Welz, S, et al. Dynamics of Cell-free Tumour DNA Correlate with Treatment Response of Head and Neck Cancer Patients Receiving Radiochemotherapy. Radiother Oncol (2020) 151:182–9. doi:10.1016/j.radonc.2020.07.027
17. Zhang, Q, Luo, J, Wu, S, Si, H, Gao, C, Xu, W, et al. Prognostic and Predictive Impact of Circulating Tumor DNA in Patients with Advanced Cancers Treated with Immune Checkpoint Blockade. Cancer Discov (2020) 10(12):1842–53. doi:10.1158/2159-8290.cd-20-0047
18. Song, Y, Hu, C, Hu, C, Xie, Z, Wu, L, Zhu, Z, et al. Circulating Tumor DNA Clearance Predicts Prognosis across Treatment Regimen in a Large Real-World Longitudinally Monitored Advanced Non-small Cell Lung Cancer Cohort. Transl Lung Cancer Res (2020) 9(2):269–79. doi:10.21037/tlcr.2020.03.17
19. Wang, Y, Yang, L, Bao, H, Fan, X, Xia, F, Wan, J, et al. Utility of ctDNA in Predicting Response to Neoadjuvant Chemoradiotherapy and Prognosis Assessment in Locally Advanced Rectal Cancer: A Prospective Cohort Study. Plos Med (2021) 18(8):e1003741. doi:10.1371/journal.pmed.1003741
20. Anagnostou, V, Forde, PM, White, JR, Niknafs, N, Hruban, C, Naidoo, J, et al. Dynamics of Tumor and Immune Responses during Immune Checkpoint Blockade in Non-small Cell Lung Cancer. Cancer Res (2019) 79(6):1214–25. doi:10.1158/0008-5472.can-18-1127
21. Adalsteinsson, VA, Ha, G, Freeman, SS, Choudhury, AD, Stover, DG, Parsons, HA, et al. Scalable Whole-Exome Sequencing of Cell-free DNA Reveals High Concordance with Metastatic Tumors. Nat Commun (2017) 8(1):1324. doi:10.1038/s41467-017-00965-y
22. Xia, S, Ye, J, Chen, Y, Lizaso, A, Huang, L, Shi, L, et al. Parallel Serial Assessment of Somatic Mutation and Methylation Profile from Circulating Tumor DNA Predicts Treatment Response and Impending Disease Progression in Osimertinib-Treated Lung Adenocarcinoma Patients. Transl Lung Cancer Res (2019) 8(6):1016–28. doi:10.21037/tlcr.2019.12.09
23. Silva, R, Moran, B, Baird, A-M, O’Rourke, CJ, Finn, SP, McDermott, R, et al. Longitudinal Analysis of Individual cfDNA Methylome Patterns in Metastatic Prostate Cancer. Clin Epigenet (2021) 13(1):168. doi:10.1186/s13148-021-01155-w
24. Aucamp, J, Bronkhorst, AJ, Badenhorst, CPS, and Pretorius, PJ. The Diverse Origins of Circulating Cell-free DNA in the Human Body: A Critical Re-evaluation of the Literature. Biol Rev (2018) 93(3):1649–83. doi:10.1111/brv.12413
25. Gold, B, Cankovic, M, Furtado, LV, Meier, F, and Gocke, CD. Do circulating Tumor Cells, Exosomes, and Circulating Tumor Nucleic Acids Have Clinical Utility? A Report of the Association for Molecular Pathology. J Mol Diagn (2015) 17(3):209–24. doi:10.1016/j.jmoldx.2015.02.001
26. Grabuschnig, S, Bronkhorst, AJ, Holdenrieder, S, Rosales Rodriguez, I, Schliep, KP, Schwendenwein, D, et al. Putative Origins of Cell-free DNA in Humans: A Review of Active and Passive Nucleic Acid Release Mechanisms. Int J Mol Sci (2020) 21(21):8062. doi:10.3390/ijms21218062
27. Kustanovich, A, Schwartz, R, Peretz, T, and Grinshpun, A. Life and Death of Circulating Cell-free DNA. Cancer Biol Ther (2019) 20(8):1057–67. doi:10.1080/15384047.2019.1598759
28. de Miranda, FS, Barauna, VG, Dos Santos, L, Costa, G, Vassallo, PF, and Campos, LCG. Properties and Application of Cell-free DNA as a Clinical Biomarker. Int J Mol Sci (2021) 22(17):9110. doi:10.3390/ijms22179110
29. Bettegowda, C, Sausen, M, Leary, RJ, Kinde, I, Wang, Y, Agrawal, N, et al. Detection of Circulating Tumor DNA in Early- and Late-Stage Human Malignancies. Sci Transl Med (2014) 6(224):224ra24. doi:10.1126/scitranslmed.3007094
30. Phallen, J, Sausen, M, Adleff, V, Leal, A, Hruban, C, White, J, et al. Direct Detection of Early-Stage Cancers Using Circulating Tumor DNA. Sci Transl Med (2017) 9(403):eaan2415. doi:10.1126/scitranslmed.aan2415
31. Hojbjerg, JA, Madsen, AT, Schmidt, HH, Sorensen, SF, Stougaard, M, Meldgaard, P, et al. Intra-individual Variation of Circulating Tumour DNA in Lung Cancer Patients. Mol Oncol (2019) 13(10):2098–106. doi:10.1002/1878-0261.12546
32. Diehl, F, Schmidt, K, Choti, MA, Romans, K, Goodman, S, Li, M, et al. Circulating Mutant DNA to Assess Tumor Dynamics. Nat Med (2008) 14(9):985–90. doi:10.1038/nm.1789
33. Cheng, ML, Pectasides, E, Hanna, GJ, Parsons, HA, Choudhury, AD, and Oxnard, GR. Circulating Tumor DNA in Advanced Solid Tumors: Clinical Relevance and Future Directions. CA Cancer J Clin (2021) 71(2):176–90. doi:10.3322/caac.21650
34. Aucamp, J, Bronkhorst, AJ, Peters, DL, Van Dyk, HC, Van der Westhuizen, FH, and Pretorius, PJ. Kinetic Analysis, Size Profiling, and Bioenergetic Association of DNA Released by Selected Cell Lines In Vitro. Cell. Mol. Life Sci. (2017) 74(14):2689–707. doi:10.1007/s00018-017-2495-z
35. Wang, W, Kong, P, Ma, G, Li, L, Zhu, J, Xia, T, et al. Characterization of the Release and Biological Significance of Cell-free DNA from Breast Cancer Cell Lines. Oncotarget (2017) 8(26):43180–91. doi:10.18632/oncotarget.17858
36. Rostami, A, Lambie, M, Yu, CW, Stambolic, V, Waldron, JN, and Bratman, SV. Senescence, Necrosis, and Apoptosis Govern Circulating Cell-free DNA Release Kinetics. Cel Rep (2020) 31(13):107830. doi:10.1016/j.celrep.2020.107830
37. Otandault, A, Abraham, J-D, Al Amir Dache, Z, Khalyfa, A, Jariel-Encontre, I, Forné, T, et al. Hypoxia Differently Modulates the Release of Mitochondrial and Nuclear DNA. Br J Cancer (2020) 122(5):715–25. doi:10.1038/s41416-019-0716-y
38. Bronkhorst, AJ, Wentzel, JF, Aucamp, J, van Dyk, E, du Plessis, L, and Pretorius, PJ. Characterization of the Cell-free DNA Released by Cultured Cancer Cells. Biochim Biophys Acta Mol Cel Res (2016) 1863(1):157–65. doi:10.1016/j.bbamcr.2015.10.022
39. Bronkhorst, AJ, Wentzel, JF, Ungerer, V, Peters, DL, Aucamp, J, de Villiers, EP, et al. Sequence Analysis of Cell-free DNA Derived from Cultured Human Bone Osteosarcoma (143B) Cells. Tumour Biol (2018) 40(9):1010428318801190. doi:10.1177/1010428318801190
40. Hu, Z-Y, Xie, N, Tian, C, Yang, X, Liu, L, Li, J, et al. Identifying Circulating Tumor DNA Mutation Profiles in Metastatic Breast Cancer Patients with Multiline Resistance. EBioMedicine (2018) 32:111–8. doi:10.1016/j.ebiom.2018.05.015
41. Zhou, Y, Xu, Y, Gong, Y, Zhang, Y, Lu, Y, Wang, C, et al. Clinical Factors Associated with Circulating Tumor DNA (Ct DNA ) in Primary Breast Cancer. Mol Oncol (2019) 13(5):1033–46. doi:10.1002/1878-0261.12456
42. Ortolan, E, Appierto, V, Silvestri, M, Miceli, R, Veneroni, S, Folli, S, et al. Blood-based Genomics of Triple-Negative Breast Cancer Progression in Patients Treated with Neoadjuvant Chemotherapy. ESMO Open (2021) 6(2):100086. doi:10.1016/j.esmoop.2021.100086
43. Abbosh, C, Birkbak, NJ, Wilson, GA, Jamal-Hanjani, M, Constantin, T, Salari, R, et al. Corrigendum: Phylogenetic ctDNA Analysis Depicts Early-Stage Lung Cancer Evolution. Nature (2018) 554(7691):264. doi:10.1038/nature25161
44. Förnvik, D, Aaltonen, KE, Chen, Y, George, AM, Brueffer, C, Rigo, R, et al. Detection of Circulating Tumor Cells and Circulating Tumor DNA before and after Mammographic Breast Compression in a Cohort of Breast Cancer Patients Scheduled for Neoadjuvant Treatment. Breast Cancer Res Treat (2019) 177(2):447–55. doi:10.1007/s10549-019-05326-5
45. Chabon, JJ, Hamilton, EG, Kurtz, DM, Esfahani, MS, Moding, EJ, Stehr, H, et al. Integrating Genomic Features for Non-invasive Early Lung Cancer Detection. Nature (2020) 580(7802):245–51. doi:10.1038/s41586-020-2140-0
46. Chen, K-Z, Lou, F, Yang, F, Zhang, J-B, Ye, H, Chen, W, et al. Circulating Tumor DNA Detection in Early-Stage Non-small Cell Lung Cancer Patients by Targeted Sequencing. Sci Rep (2016) 6:31985. doi:10.1038/srep31985
47. Cho, M-S, Park, CH, Lee, S, and Park, HS. Clinicopathological Parameters for Circulating Tumor DNA Shedding in Surgically Resected Non-small Cell Lung Cancer with EGFR or KRAS Mutation. PLoS One (2020) 15(3):e0230622. doi:10.1371/journal.pone.0230622
48. Avanzini, S, Kurtz, DM, Chabon, JJ, Moding, EJ, Hori, SS, Gambhir, SS, et al. A Mathematical Model of ctDNA Shedding Predicts Tumor Detection Size. Sci Adv (2020) 6(50):eabc4308. doi:10.1126/sciadv.abc4308
49. McEvoy, AC, Warburton, L, Al-Ogaili, Z, Celliers, L, Calapre, L, Pereira, MR, et al. Correlation between Circulating Tumour DNA and Metabolic Tumour burden in Metastatic Melanoma Patients. BMC Cancer (2018) 18(1):726. doi:10.1186/s12885-018-4637-6
50. Chen, Z, Fadiel, A, Naftolin, F, Eichenbaum, KD, and Xia, Y. Circulation DNA: Biological Implications for Cancer Metastasis and Immunology. Med Hypotheses (2005) 65(5):956–61. doi:10.1016/j.mehy.2005.04.042
51. Ungerer, V, Bronkhorst, AJ, Van den Ackerveken, P, Herzog, M, and Holdenrieder, S. Serial Profiling of Cell-free DNA and Nucleosome Histone Modifications in Cell Cultures. Sci Rep (2021) 11(1):9460. doi:10.1038/s41598-021-88866-5
52. Fleischhacker, M, and Schmidt, B. Circulating Nucleic Acids (CNAs) and Cancer-A Survey. Biochim Biophys Acta Rev Cancer (2007) 1775(1):181–232. doi:10.1016/j.bbcan.2006.10.001
53. Choi, JJ, Reich, CF, and Pisetsky, DS. Release of DNA from Dead and Dying Lymphocyte and Monocyte Cell Lines In Vitro. Scand J Immunol (2004) 60(1-2):159–66. doi:10.1111/j.0300-9475.2004.01470.x
54. Utani, K-i., Okamoto, A, and Shimizu, N. Generation of Micronuclei during Interphase by Coupling between Cytoplasmic Membrane Blebbing and Nuclear Budding. PLoS One (2011) 6(11):e27233. doi:10.1371/journal.pone.0027233
55. Kisurina-Evgenieva, OP, Sutiagina, OI, and Onishchenko, GE. Biogenesis of Micronuclei. Biochem Mosc (2016) 81(5):453–64. doi:10.1134/s0006297916050035
56. Ryoo, HD, and Bergmann, A. The Role of Apoptosis-Induced Proliferation for Regeneration and Cancer. Cold Spring Harbor Perspect Biol (2012) 4(8):a008797. doi:10.1101/cshperspect.a008797
57. Lugano, R, Ramachandran, M, and Dimberg, A. Tumor Angiogenesis: Causes, Consequences, Challenges and Opportunities. Cel Mol. Life Sci. (2020) 77(9):1745–70. doi:10.1007/s00018-019-03351-7
58. Wang, J, Huang, A, Wang, Y-P, Yin, Y, Fu, P-Y, Zhang, X, et al. Circulating Tumor DNA Correlates with Microvascular Invasion and Predicts Tumor Recurrence of Hepatocellular Carcinoma. Ann Transl Med (2020) 8(5):237. doi:10.21037/atm.2019.12.154
59. Shirasu, H, Taniguchi, H, Watanabe, J, Kotaka, M, Yamazaki, K, Hirata, K, et al. O-11 Monitoring Molecular Residual Disease by Circulating Tumor DNA in Resectable Colorectal Cancer: Molecular Subgroup Analyses of a Prospective Observational Study GALAXY in CIRCULATE-Japan. Ann Oncol (2021) 32:S222–S223. doi:10.1016/j.annonc.2021.05.015
60. Nabavizadeh, SA, Ware, JB, Guiry, S, Nasrallah, MP, Mays, JJ, Till, JE, et al. Imaging and Histopathologic Correlates of Plasma Cell-free DNA Concentration and Circulating Tumor DNA in Adult Patients with Newly Diagnosed Glioblastoma. Neurooncol Adv (2020) 2(1):vdaa016. doi:10.1093/noajnl/vdaa016
61. Bagley, SJ, Nabavizadeh, SA, Mays, JJ, Till, JE, Ware, JB, Levy, S, et al. Clinical Utility of Plasma Cell-free DNA in Adult Patients with Newly Diagnosed Glioblastoma: A Pilot Prospective Study. Clin Cancer Res (2020) 26(2):397–407. doi:10.1158/1078-0432.ccr-19-2533
62. Mair, R, Mouliere, F, Smith, CG, Chandrananda, D, Gale, D, Marass, F, et al. Measurement of Plasma Cell-free Mitochondrial Tumor DNA Improves Detection of Glioblastoma in Patient-Derived Orthotopic Xenograft Models. Cancer Res (2019) 79(1):220–30. doi:10.1158/0008-5472.can-18-0074
63. McKeown, SR. Defining Normoxia, Physoxia and Hypoxia in Tumours-Implications for Treatment Response. Br J Radiol (2014) 87(1035):20130676. doi:10.1259/bjr.20130676
64. Harris, AL. Hypoxia - A Key Regulatory Factor in Tumour Growth. Nat Rev Cancer (2002) 2(1):38–47. doi:10.1038/nrc704
65. Giacona, MB, Ruben, GC, Iczkowski, KA, Roos, TB, Porter, DM, and Sorenson, GD. Cell-free DNA in Human Blood Plasma: Length Measurements in Patients with Pancreatic Cancer and Healthy Controls. Pancreas (1998) 17(1):89–97. doi:10.1097/00006676-199807000-00012
66. Jahr, S, Hentze, H, Englisch, S, Hardt, D, Fackelmayer, FO, Hesch, RD, et al. DNA Fragments in the Blood Plasma of Cancer Patients: Quantitations and Evidence for Their Origin from Apoptotic and Necrotic Cells. Cancer Res (2001) 61(4):1659–65.
67. Cortese, R, Almendros, I, Wang, Y, and Gozal, D. Tumor Circulating DNA Profiling in Xenografted Mice Exposed to Intermittent Hypoxia. Oncotarget (2015) 6(1):556–69. doi:10.18632/oncotarget.2785
68. Lindberg, HK, Wang, X, Järventaus, H, Falck, GC, Norppa, H, and Fenech, M. Origin of Nuclear Buds and Micronuclei in normal and Folate-Deprived Human Lymphocytes. Mutat Res (2007) 617(1-2):33–45. doi:10.1016/j.mrfmmm.2006.12.002
69. Al-Asmakh, M, and Hedin, L. Microbiota and the Control of Blood-Tissue Barriers. Tissue Barriers (2015) 3(3):e1039691. doi:10.1080/21688370.2015.1039691
70. Lo, YMD, Tein, MSC, Lau, TK, Haines, CJ, Leung, TN, Poon, PMK, et al. Quantitative Analysis of Fetal DNA in Maternal Plasma and Serum: Implications for Noninvasive Prenatal Diagnosis. Am J Hum Genet (1998) 62(4):768–75. doi:10.1086/301800
71. Zhu, L, Nazeri, A, Pacia, CP, Yue, Y, and Chen, H. Focused Ultrasound for Safe and Effective Release of Brain Tumor Biomarkers into the Peripheral Circulation. PLoS One (2020) 15(6):e0234182. doi:10.1371/journal.pone.0234182
72. Pacia, CP, Zhu, L, Yang, Y, Yue, Y, Nazeri, A, Michael Gach, H, et al. Feasibility and Safety of Focused Ultrasound-Enabled Liquid Biopsy in the Brain of a Porcine Model. Sci Rep (2020) 10(1):7449. doi:10.1038/s41598-020-64440-3
73. Choi, J-J, Reich, CF, and Pisetsky, DS. The Role of Macrophages in the In Vitro Generation of Extracellular DNA from Apoptotic and Necrotic Cells. Immunology (2005) 115(1):55–62. doi:10.1111/j.1365-2567.2005.02130.x
74. Jiang, N, Reich, CF, and Pisetsky, DS. Role of Macrophages in the Generation of Circulating Blood Nucleosomes from Dead and Dying Cells. Blood (2003) 102(6):2243–50. doi:10.1182/blood-2002-10-3312
75. Herbreteau, G, Vallee, A, Knol, AC, Theoleyre, S, Quereux, G, Varey, E, et al. Circulating Tumor DNA Early Kinetics Predict Response of Metastatic Melanoma to Anti-PD1 Immunotherapy: Validation Study. Cancers (Basel) (2021) 13(8):1826. doi:10.3390/cancers13081826
76. Westman, J, Grinstein, S, and Marques, PE. Phagocytosis of Necrotic Debris at Sites of Injury and Inflammation. Front Immunol (2019) 10:3030. doi:10.3389/fimmu.2019.03030
77. Lo, YMD, Zhang, J, Leung, TN, Lau, TK, Chang, AMZ, and Hjelm, NM. Rapid Clearance of Fetal DNA from Maternal Plasma. Am J Hum Genet (1999) 64(1):218–24. doi:10.1086/302205
78. Patel, PS, Patel, BP, Rawal, RM, Raval, GN, Patel, MM, Patel, JB, et al. Evaluation of Serum Alkaline DNase Activity in Treatment Monitoring of Head and Neck Cancer Patients. Tumor Biol (2000) 21(2):82–9. doi:10.1159/000030113
79. Martin, M, Leffler, J, Smoląg, KI, Mytych, J, Björk, A, Chaves, LD, et al. Factor H Uptake Regulates Intracellular C3 Activation during Apoptosis and Decreases the Inflammatory Potential of Nucleosomes. Cell Death Differ (2016) 23(5):903–11. doi:10.1038/cdd.2015.164
80. Kirsch, C, Weickmann, S, Schmidt, B, and Fleischhacker, M. An Improved Method for the Isolation of Free-Circulating Plasma DNA and Cell-free DNA from Other Body Fluids. Ann N Y Acad Sci (2008) 1137:135–9. doi:10.1196/annals.1448.035
81. Gauthier, VJ, Tyler, LN, and Mannik, M. Blood Clearance Kinetics and Liver Uptake of Mononucleosomes in Mice. J Immunol (1996) 156(3):1151–6.
82. Korabecna, M, Opatrna, S, Wirth, J, Rulcova, K, Eiselt, J, Sefrna, F, et al. Cell-free Plasma DNA during Peritoneal Dialysis and Hemodialysis and in Patients with Chronic Kidney Disease. Ann N Y Acad Sci (2008) 1137:296–301. doi:10.1196/annals.1448.014
83. Cheng, THT, Jiang, P, Tam, JCW, Sun, X, Lee, W-S, Yu, SCY, et al. Genomewide Bisulfite Sequencing Reveals the Origin and Time-dependent Fragmentation of Urinary cfDNA. Clin Biochem (2017) 50(9):496–501. doi:10.1016/j.clinbiochem.2017.02.017
84. Hung, ECW, Shing, TKF, Chim, SSC, Yeung, PC, Chan, RWY, Chik, KW, et al. Presence of Donor-Derived DNA and Cells in the Urine of Sex-Mismatched Hematopoietic Stem Cell Transplant Recipients: Implication for the Transrenal Hypothesis. Clin Chem (2009) 55(4):715–22. doi:10.1373/clinchem.2008.113530
85. Costa, DB, Halmos, B, Kumar, A, Schumer, ST, Huberman, MS, Boggon, TJ, et al. BIM Mediates EGFR Tyrosine Kinase Inhibitor-Induced Apoptosis in Lung Cancers with Oncogenic EGFR Mutations. Plos Med (2007) 4(10):1669–80. doi:10.1371/journal.pmed.0040315
86. Phallen, J, Leal, A, Woodward, BD, Forde, PM, Naidoo, J, Marrone, KA, et al. Early Noninvasive Detection of Response to Targeted Therapy in Non-small Cell Lung Cancer. Cancer Res (2019) 79(6):1204–13. doi:10.1158/0008-5472.can-18-1082
87. Blankenberg, FG. In Vivo imaging of Apoptosis. Cancer Biol Ther (2008) 7(10):1525–32. doi:10.4161/cbt.7.10.6934
88. Maier, P, Hartmann, L, Wenz, F, and Herskind, C. Cellular Pathways in Response to Ionizing Radiation and Their Targetability for Tumor Radiosensitization. Int J Mol Sci (2016) 17(1):102. doi:10.3390/ijms17010102
89. Patsch, K, Matasci, N, Soundararajan, A, Diaz, P, Agus, DB, Ruderman, D, et al. Monitoring Dynamic Cytotoxic Chemotherapy Response in Castration-Resistant Prostate Cancer Using Plasma Cell-free DNA (cfDNA). BMC Res Notes (2019) 12(1):275. doi:10.1186/s13104-019-4312-2
90. Moser, T, Waldispuehl-Geigl, J, Belic, J, Weber, S, Zhou, Q, Hasenleithner, SO, et al. On-treatment Measurements of Circulating Tumor DNA during FOLFOX Therapy in Patients with Colorectal Cancer. NPJ Precis Oncol (2020) 4(1):30. doi:10.1038/s41698-020-00134-3
91. Lyskjær, I, Kronborg, CS, Rasmussen, MH, Sørensen, BS, Demuth, C, Rosenkilde, M, et al. Correlation between Early Dynamics in Circulating Tumour DNA and Outcome from FOLFIRI Treatment in Metastatic Colorectal Cancer. Sci Rep (2019) 9(1):11542. doi:10.1038/s41598-019-47708-1
92. Wang, H, Zhang, B, Chen, D, Xia, W, Zhang, J, Wang, F, et al. Real-time Monitoring Efficiency and Toxicity of Chemotherapy in Patients with Advanced Lung Cancer. Clin Epigenet (2015) 7:119. doi:10.1186/s13148-015-0150-9
93. Kruger, S, Heinemann, V, Ross, C, Diehl, F, Nagel, D, Ormanns, S, et al. Repeated mutKRAS ctDNA Measurements Represent a Novel and Promising Tool for Early Response Prediction and Therapy Monitoring in Advanced Pancreatic Cancer. Ann Oncol (2018) 29(12):2348–55. doi:10.1093/annonc/mdy417
94. Chera, BS, Kumar, S, Shen, C, Amdur, R, Dagan, R, Green, R, et al. Plasma Circulating Tumor HPV DNA for the Surveillance of Cancer Recurrence in HPV-Associated Oropharyngeal Cancer. J Clin Oncol (2020) 38(10):1050–8. doi:10.1200/jco.19.02444
95. Moding, EJ, Liu, Y, Hui, AB, He, J, Qiao, Y, Xu, T, et al. Abstract PO-069: Circulating Tumor DNA Kinetics to Identify Genomic Predictors of Rapid Response to Chemoradiation in Non-small Cell Lung Cancer. Clin Cancer Res (2021) 27(8 Suppl.):PO-069. doi:10.1158/1557-3265.radsci21-po-069
96. Breadner, DA, Vincent, MD, Correa, R, Black, M, Warner, A, Sanatani, M, et al. Exploitation of Treatment Induced Tumor Lysis to Enhance the Sensitivity of ctDNA Analysis: A First-In-Human Pilot Study. Lung Cancer (2022) 165:145–51. doi:10.1016/j.lungcan.2022.01.013
97. Leon, SA, Shapiro, B, Sklaroff, DM, and Yaros, MJ. Free DNA in the Serum of Cancer Patients and the Effect of Therapy. Cancer Res (1977) 37(3):646–50.
98. Medvedeva, NG, Panyutin, IV, Panyutin, IG, and Neumann, RD. Phosphorylation of Histone H2AX in Radiation-Induced Micronuclei. Radiat Res (2007) 168(4):493–8. doi:10.1667/rr0788.1
99. Durante, M, and Formenti, SC. Radiation-Induced Chromosomal Aberrations and Immunotherapy: Micronuclei, Cytosolic DNA, and Interferon-Production Pathway. Front Oncol (2018) 8:192. doi:10.3389/fonc.2018.00192
100. Walls, GM, McConnell, L, McAleese, J, Murray, P, Lynch, TB, Savage, K, et al. Early Circulating Tumour DNA Kinetics Measured by Ultra-deep Next-Generation Sequencing during Radical Radiotherapy for Non-small Cell Lung Cancer: A Feasibility Study. Radiat Oncol (2020) 15(1):132. doi:10.1186/s13014-020-01583-7
101. Boniface, C, Baker, K, Dieg, C, Halsey, C, Rahmani, R, Deffenbach, M, et al. Abstract PR05: Radiation-Assisted Amplification Sequencing (RAMP-Seq): Evaluating the Use of Stereotactic Body Radiation Therapy (SBRT) for Enriching Circulating Tumor DNA in Liquid Biopsies. Clin Cancer Res (2020) 26(11 Suppl.):PR05. doi:10.1158/1557-3265.liqbiop20-pr05
102. Chen, EL, Chaudhuri, AA, Nabet, BY, Chabon, JJ, Merriott, DJ, Loo, BW, et al. Analysis of Circulating Tumor DNA Kinetics during Stereotactic Ablative Radiation Therapy for Non-small Cell Lung Cancer. Int J Radiat Oncol Biol Phys (2018) 102(3 Suppl.):e676. doi:10.1016/j.ijrobp.2018.07.1826
103. Chaudhuri, AA, Lovejoy, AF, Chabon, JJ, Newman, A, Stehr, H, Merriott, DJ, et al. Circulating Tumor DNA Analysis during Radiation Therapy for Localized Lung Cancer Predicts Treatment Outcome. Int J Radiat Oncol Biol Phys (2017) 99(2 Suppl.):S1–S2. doi:10.1016/j.ijrobp.2017.06.021
104. Johnson, BE, Creason, AL, Stommel, JM, Keck, JM, Parmar, S, Betts, CB, et al. An Omic and Multidimensional Spatial Atlas from Serial Biopsies of an Evolving Metastatic Breast Cancer. Cel Rep Med (2022) 3(2):100525. doi:10.1016/j.xcrm.2022.100525
105. Mole, RH. Whole Body Irradiation-Radiobiology or Medicine? Br J Radiol (1953) 26(305):234–41. doi:10.1259/0007-1285-26-305-234
106. Neumann, MHD, Bender, S, Krahn, T, and Schlange, T. ctDNA and CTCs in Liquid Biopsy - Current Status and where We Need to Progress. Comput Struct Biotechnol J (2018) 16:190–5. doi:10.1016/j.csbj.2018.05.002
107. Ng, SP, Meas, S, Bahig, H, Skinner, HD, Garden, AS, Phan, J, et al. Detection of Circulating Tumor Cells during Radiation Therapy in Patients with Head and Neck Cancer. Int J Radiat Oncol Biol Phys (2018) 102(3 Suppl.):e177. doi:10.1016/j.ijrobp.2018.07.657
108. Lee, JH, Long, GV, Menzies, AM, Lo, S, Guminski, A, Whitbourne, K, et al. Association between Circulating Tumor DNA and Pseudoprogression in Patients with Metastatic Melanoma Treated with Anti-programmed Cell Death 1 Antibodies. JAMA Oncol (2018) 4(5):717–21. doi:10.1001/jamaoncol.2017.5332
109. Ma, Y, Wang, Q, Dong, Q, Zhan, L, and Zhang, J. How to Differentiate Pseudoprogression from True Progression in Cancer Patients Treated with Immunotherapy. Am J Cancer Res (2019) 9(8):1546–53.
110. Váraljai, R, Elouali, S, Lueong, SS, Wistuba-Hamprecht, K, Seremet, T, Siveke, JT, et al. The Predictive and Prognostic Significance of Cell-free DNA Concentration in Melanoma. J Eur Acad Dermatol Venereol (2021) 35(2):387–95. doi:10.1111/jdv.16766
111. Goldberg, SB, Narayan, A, Kole, AJ, Decker, RH, Teysir, J, Carriero, NJ, et al. Early Assessment of Lung Cancer Immunotherapy Response via Circulating Tumor DNA. Clin Cancer Res (2018) 24(8):1872–80. doi:10.1158/1078-0432.ccr-17-1341
112. Kato, K, Uchida, J, Kukita, Y, Kumagai, T, Nishino, K, Inoue, T, et al. Transient Appearance of Circulating Tumor DNA Associated with De Novo Treatment. Sci Rep (2016) 6:38639. doi:10.1038/srep38639
113. Riediger, AL, Dietz, S, Schirmer, U, Meister, M, Heinzmann-Groth, I, Schneider, M, et al. Mutation Analysis of Circulating Plasma DNA to Determine Response to EGFR Tyrosine Kinase Inhibitor Therapy of Lung Adenocarcinoma Patients. Sci Rep (2016) 6:33505. doi:10.1038/srep33505
114. Horn, L, Whisenant, JG, Wakelee, H, Reckamp, KL, Qiao, H, Leal, TA, et al. Monitoring Therapeutic Response and Resistance: Analysis of Circulating Tumor DNA in Patients with ALK+ Lung Cancer. J Thorac Oncol (2019) 14(11):1901–11. doi:10.1016/j.jtho.2019.08.003
115. Wyatt, AW, Azad, AA, Volik, SV, Annala, M, Beja, K, McConeghy, B, et al. Genomic Alterations in Cell-free DNA and Enzalutamide Resistance in Castration-Resistant Prostate Cancer. JAMA Oncol (2016) 2(12):1598–606. doi:10.1001/jamaoncol.2016.0494
116. Romanel, A, Gasi Tandefelt, D, Conteduca, V, Jayaram, A, Casiraghi, N, Wetterskog, D, et al. Plasma AR and Abiraterone-Resistant Prostate Cancer. Sci Transl Med (2015) 7(312):312re10. doi:10.1126/scitranslmed.aac9511
117. Breadner, DA, Vincent, MD, Correa, RJM, Black, M, Warner, A, Qu, M, et al. Exploitation of Treatment Induced Tumor Lysis to Enhance Sensitivity of ctDNA Analysis: A First-In-Human Pilot Study. J Clin Oncol (2020) 38(15_Suppl. l):3530. doi:10.1200/jco.2020.38.15_suppl.3530
118. van de Donk, PP, Kist de Ruijter, L, Lub-de Hooge, MN, Brouwers, AH, van der Wekken, AJ, Oosting, SF, et al. Molecular Imaging Biomarkers for Immune Checkpoint Inhibitor Therapy. Theranostics (2020) 10(4):1708–18. doi:10.7150/thno.38339
119. Wagner, JT, Kim, HJ, Johnson-Camacho, KC, Kelley, T, Newell, LF, Spellman, PT, et al. Diurnal Stability of Cell-free DNA and Cell-free RNA in Human Plasma Samples. Sci Rep (2020) 10(1):16456. doi:10.1038/s41598-020-73350-3
Keywords: biomarkers, liquid biopsy, circulating tumor DNA, ctDNA, tumor growth, treatment response
Citation: Boniface CT and Spellman PT (2022) Blood, Toil, and Taxoteres: Biological Determinants of Treatment-Induced ctDNA Dynamics for Interpreting Tumor Response. Pathol. Oncol. Res. 28:1610103. doi: 10.3389/pore.2022.1610103
Received: 02 October 2021; Accepted: 29 April 2022;
Published: 19 May 2022.
Edited by:
József Tímár, Semmelweis University, HungaryCopyright © 2022 Boniface and Spellman. This is an open-access article distributed under the terms of the Creative Commons Attribution License (CC BY). The use, distribution or reproduction in other forums is permitted, provided the original author(s) and the copyright owner(s) are credited and that the original publication in this journal is cited, in accordance with accepted academic practice. No use, distribution or reproduction is permitted which does not comply with these terms.
*Correspondence: Christopher T. Boniface, Ym9uaWZhY2VAb2hzdS5lZHU=; Paul T. Spellman, c3BlbGxtYXBAb2hzdS5lZHU=