- 1Department of Pharmacology, School of Pharmacy, China Medical University, Shenyang, China
- 2Liaoning Key Laboratory of Molecular Targeted Anti-tumor Drug Development and Evaluation China Medical University, Shenyang, China
- 3Department of Family Medicine, Shengjing Hospital, China Medical University, Shenyang, China
Head and neck squamous cell cancer (HNSCC) is one of the most common types of cancer worldwide. There have been many reports suggesting that biomarkers explored via database mining plays a critical role in predicting HNSCC prognosis. However, a single biomarker for prognostic analysis is not adequate. Additionally, there is growing evidence indicating that gene signature could be a better choice for HNSCC prognosis. We performed a comprehensive analysis of mRNA expression profiles using clinical information of HNSCC patients from The Cancer Genome Atlas (TCGA). Gene Set Enrichment Analysis (GSEA) was performed, and we found that a set of genes involved in epithelial mesenchymal transition (EMT) contributed to HNSCC. Cox proportional regression model was used to identify a four-gene (WIPF1, PPIB, BASP1, PLOD2) signature that were significantly associated with overall survival (OS), and all the four genes were significantly upregulated in tumor tissues. We successfully classified the patients with HNSCC into high-risk and low-risk groups, where in high-risk indicated poorer patient prognosis, indicating that this gene signature might be a novel potential biomarker for the prognosis of HNSCC. The prognostic ability of the gene signature was further validated in an independent cohort from the Gene Expression Omnibus (GEO) database. In conclusion, we identified a four-EMT-based gene signature which provides the potentiality to serve as novel independent biomarkers for predicting survival in HNSCC patients, as well as a new possibility for individualized treatment of HNSCC.
Introduction
Head and neck cancer is the sixth most common types of cancer, with about half a million new cases annually diagnosed worldwide [1], among which 350,000 individuals die of it [2]. Although local control rate and quality of life have improved for head and neck squamous cell cancer (HNSCC) owing to advances in surgical techniques and comprehensive treatment techniques, overall survival (OS) has not increased significantly in recent decades. Moreover, the 5-years survival rate of patients with this disease is only 40–50% [3]. In recent years, age, clinical stage, and smoking status which are characteristics emerging as important contributors to the clinical outcome might help improve survival prediction for patients afflicted by HNSCC [4–6]. However, due to the complex molecular mechanisms underlying cancer regulation, the conventional clinical information has limited predictive ability.
Increasing evidence has revealed the important clinical significance of mRNA expression in various pathological and physiological processes of multiple tumor histotypes, including HNSCC. For instance, decreased calpain six expression has been known to be associated with tumorigenesis and poor prognosis of HNSCC [7]. Furthermore, FcGBP expression was upregulated by HPV infection and correlated with longer survival time of HNSCC patients [8]. However, compared with single-gene biomarkers, tumor signatures including several genes have been identified, which might be better choices to facilitate clinical application, provide insights into cancer progression, as well as reveal potentially new therapeutic targets [9, 10]. Thus, it is necessary to establish an expression-based gene signature for predicting survival of HNSCC patients for effective clinical decisions making with respect to optimal treatment regimen.
Metastasis is a complex, highly inefficient, but deadly process that has been under intense investigation in hopes to eliminate distant spread of metastasis and reduce cancer-associated mortality. A key event in promoting stationary tumor cells to migrate and invade is the epithelial mesenchymal transition (EMT) [11]. Specific tumor cell populations with EMT are associated with more aggressive tumor phenotypes and worse outcomes [12, 13]. Therefore, understanding the relationship between EMT and tumor is crucial for elucidating the underlying mechanism of tumorigenesis. Gene Set Enrichment Analysis (GSEA) is not concerned with a limited number of different genes that have significantly changed, but with whether the expression of these detected genes exhibit a common expression pattern in the defined functional groups, and interprets biological information to elaborate its biological significance from another perspective. In the present study, we tried to identify the relationship between the metastatic cascade system and HNSCC by GSEA analysis [14]. As expected, EMT-related risk signature could independently identify patients afflicted by HNSCC with high-risk and poor prognosis.
Materials and Methods
Data Acquisition
We downloaded the mRNA expression profiles and clinical information of head and neck squamous cell cancer patients from the TCGA database (https://cancergenome.nih.gov/). A total of 546 samples involving tumor tissue samples and adjacent noncancerous tissues participated in the study, and their corresponding clinical data including age, sex, TNM classification, OS status, as well as disease-free survival (DFS) status were also examined. The general clinical features are listed in Table 1. As an external validation cohort, the independent data set GSE27020 based upon the GPL96 platform and survival information were extracted from the Gene Expression Omnibus (GEO) database (https://www.ncbi.nlm.nih.gov/geo/).
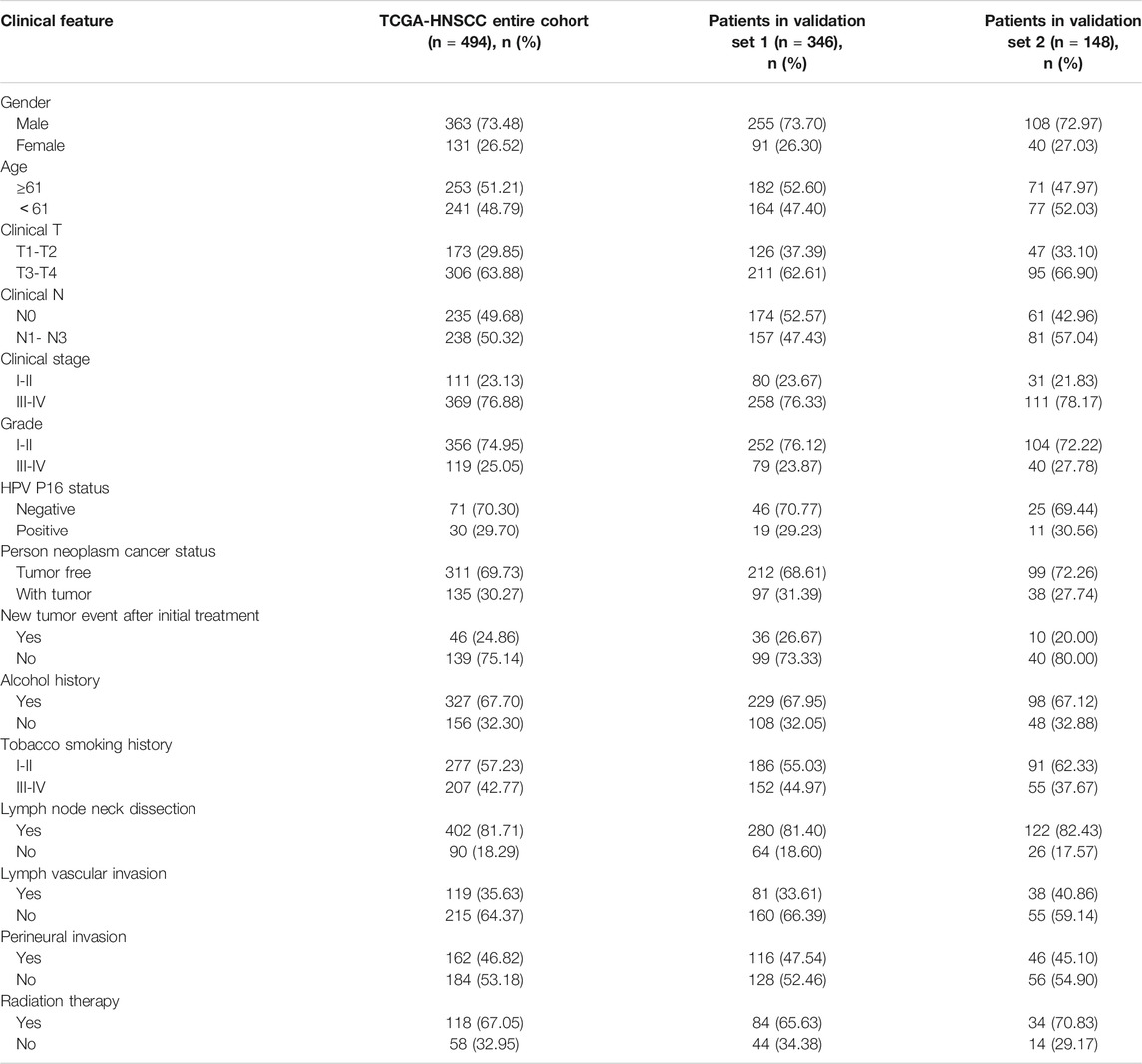
TABLE 1. Summary information of clinical characteristics of HNSCC patients in entire TCGA set (n = 494), TCGA validation set 1 (n = 346), TCGA validation set 2 (n = 148).
Gene Set Enrichment Analysis
GSEA (http://www.broadinstitute.org/gsea/index.jsp) was performed to explore whether the gene sets identified between the two groups showed a significant difference [15, 16]. We conducted GSEA to investigate the differences of biological pathways associated with tumorigenesis and progression between adjacent noncancerous tissue and HNSCC samples by selecting the hallmark gene sets as the reference gene set file. Normalized p values (p < 0.05) were set as threshold to determine which functions for further investigation.
Gene Ontology Analysis
We used DAVID (the Database for Annotation, Visualization and Integration Discovery v6.8, https://david.ncifcrf.gov/) to analyze the TCGA-HNSCC cohort, to obtain the enriched pathways for differentially expressed genes between four-mRNA-low-risk and high-risk groups [17].
Statistical Analysis
Using gene expression profiles as the original data, each gene was normalized by log2 transformed values for further analysis. Univariate Cox regression analysis was used to calculate the association between mRNA expression levels and OS rate of patients. mRNAs were considered significant if their p-values were less than 0.05. Candidate genes were fitted in a stepwise multivariate Cox proportional regression model to identify predictive models with optimal interpretation and valuable information. We used the R package “survival” to build a risk score model. The formula for the risk score is described below: Risk score = expression of gene 1*β1+ expression of gene 2*β2+…+expression of gene n*βn. The filtered mRNAs were classified into risky [hazard ratio (HR) >1] and protective (0 < HR < 1) types. All patients were classified into a high-risk and a low-risk group according to the median risk score. Additionally, the correlations between the risk score and clinical features of HNSCC patients were analyzed by using Chi-square test. Time-dependent receiver-operating characteristic (ROC) curves and area under the ROC curve (AUC) values were calculated to measure prognostic accuracy. Afterward, we applied the R package “caret” to randomly divided TCGA-HNSCC patients into two sets (TCGA validation set 1, n = 346 and TCGA validation set 2, n = 148) at a ratio of 7:3. The prognostic signature was subsequently validated in both internal validation sets and external independent data set GSE27020. All statistical analyses were performed using SPSS 24.0 and GraphPad Prism8 software.
Results
Using GSEA for Preliminary Screening of Genes
Clinical features of TGCA-HNSCC cohort (n = 546), and expression data sets of HNSCC patients were obtained from TCGA. GSEA was applied to explore whether the gene sets identified as EMT, G2/M checkpoint, E2F targets, MYC targets V2 and MYC targets V1 showed statistically significant differences between HNSCC samples and adjacent normal tissues. We found that all the five gene sets were significantly enriched with normalized p-values < 0.05 (Figure 1; Table 2). We then selected the EMT gene set which was of our interest (p = 0.013), containing 94 core genes (Supplementary Table S1) for further analysis.
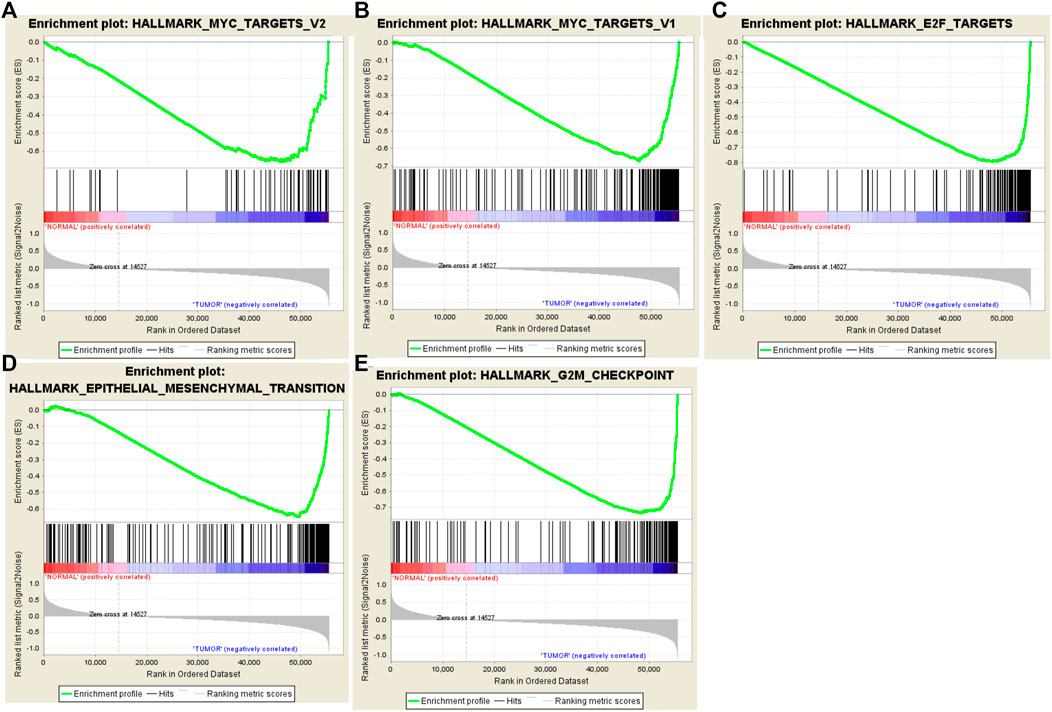
FIGURE 1. Enrichment plots of five gene sets which were significantly different in normal and HNSCC tissues using GSEA. [Including (A) MYC targets V2; (B) MYC targets V1; (C) E2F targets; (D) Epithelial Mesenchymal Transition; (E) G2/M checkpoint].
Identification of EMT mRNAs Related to HNSCC Patient Survival
We first performed a preliminary screening of 94 genes by univariate Cox regression analysis and obtained 12 genes (Supplementary Table S2) with a p-value <0.05. Next, using multivariate Cox regression analysis, we further examined the association between the 12 mRNA expression profiles and patient survival. Subsequently, we confirmed four-mRNA signature (WIPF1, PPIB, BASP1 and PLOD2), with a p-value < 0.001, as an independent prognostic indicator of HNSCC. Filtered mRNAs were classified as risky type (PPIB, BASP1 and PLOD2) with β(cox) was >0 and shorter survival, and a protective type (WIPF1), with β(cox) was <0 and longer survival (Table 3).
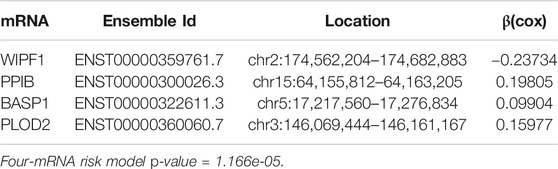
TABLE 3. Detailed information of four prognostic mRNAs significantly associated with OS in patients with HNSCC.
Differential expression of four genes in adjacent normal tissues was also investigated compared to HNSCC tissues. We found that these four genes were up-regulated in tumor tissues with significant differences (p < 0.05, Figure 2).
Four-mRNA Signature Was Constructed to Predict the Prognosis of Patients
Based on the linear combinations of the expression level, the formula for prognostic risk rating was established, and the expression level was weighted by the regression coefficients from the multivariable Cox regression analysis. Risk score = 0.19805 * expression of PPIB + 0.09904 * expression of BASP1 + 0.15977 * expression of PLOD2−0.23734 * expression of WIPF1. Every HNSCC patient had only one risk score. We calculated the scores and then ranked the patients in order of increased risk scores. Based on the median point (0.994727), we then classified them into high-risk and low-risk groups (Figure 3A). The distribution and survival status for each patient is shown in Figure 3B. The median survival time of the high-risk and low-risk group was 998 and 1762 days, respectively. In addition, the mortality rate of the high-risk group was 48.6%, whereas the corresponding rate in the low-risk group was 38.7%. The mortality rate of patients with a high-risk score was higher than that of patients with a low-risk score (p = 0.0268). According to the univariate Cox regression analysis of OS, compared to the low-risk group, a 1.573-fold increased risk of death (95% CI 1.200–2.062, p = 0.001) was determined for the high-risk group. Further, the heat map displayed the expression profiles of four-mRNAs (Figure 3C). HNSCC patients with an increased risk score showed significantly upregulated expression of high-risk type mRNA (PPIB, BASP1 and PLOD2); in contrast, the expression of protective type mRNA (WIPF1) was down-regulated.
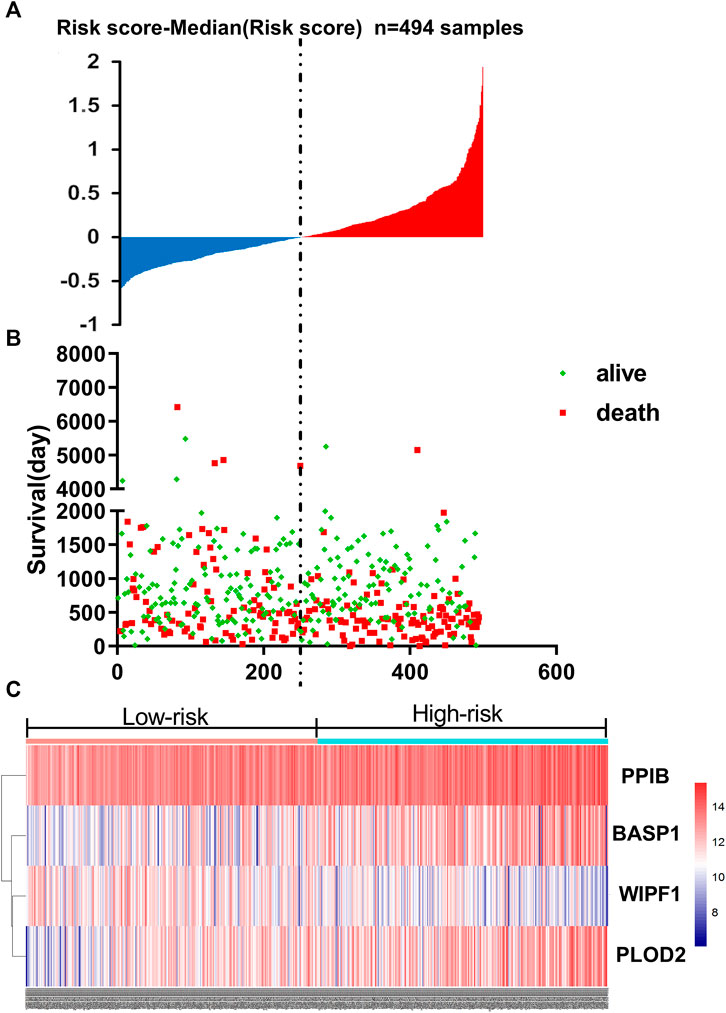
FIGURE 3. The four-mRNA signature related to risk score predicts OS in patients with HNSCC (A) mRNA risk score distribution in each patient. (B) Survival (in days) of patients in order of the value of risk scores. (C) A heatmap showing the expression profile of the four selected genes.
Risk Scores Generated by Four-mRNA Signature as Independent Prognostic Indicators in HNSCC
The results of Chi-square analysis showed that patients in the low-risk group had better clinicopathological parameters, including tumor grade (χ2 = 10.619, p = 0.014), neoplasm cancer status (χ2 = 6.948, p = 0.008), new tumor event after initial treatment (χ2 = 5.535, p = 0.018), compared with those in high-risk cohort (Table 4). Comparison of prognostic values of risk scores with clinical pathology parameters using univariate and multivariate analysis (Table 5). Univariate Cox analysis showed that the high-risk group was closely associated with poor survival of HNSCC patients (HR = 2.177, 95% CI 1.627–2.912, p < 0.001). After adjusting for clinical and pathological parameters, we found that the risk score (HR = 1.844, 95% CI 1.198–2.836, p = 0.005), margin status (HR = 1.410, 95% CI 1.107–1.798, p = 0.005), and presence of perineural invasion (HR = 2.218, 95% CI 1.490–3.302, p < 0.001) were still independent prognostic indicators in the multivariate Cox analysis. To assess the capabilities of the four-EMT-based classifier to predict OS of HNSCC, we plotted the ROC curves and the AUC value for the long-time survival at 3 years of our signature was 0.620 (Figure 4A). In consideration of the role of classical parameters in clinical practice, we combined the EMT-based model and the classical clinical parameters to predict OS of HNSCC, and the AUC value was 0.716, indicating that this model was more accurate than models enrolled in EMT-related signature or clinical parameters solely.
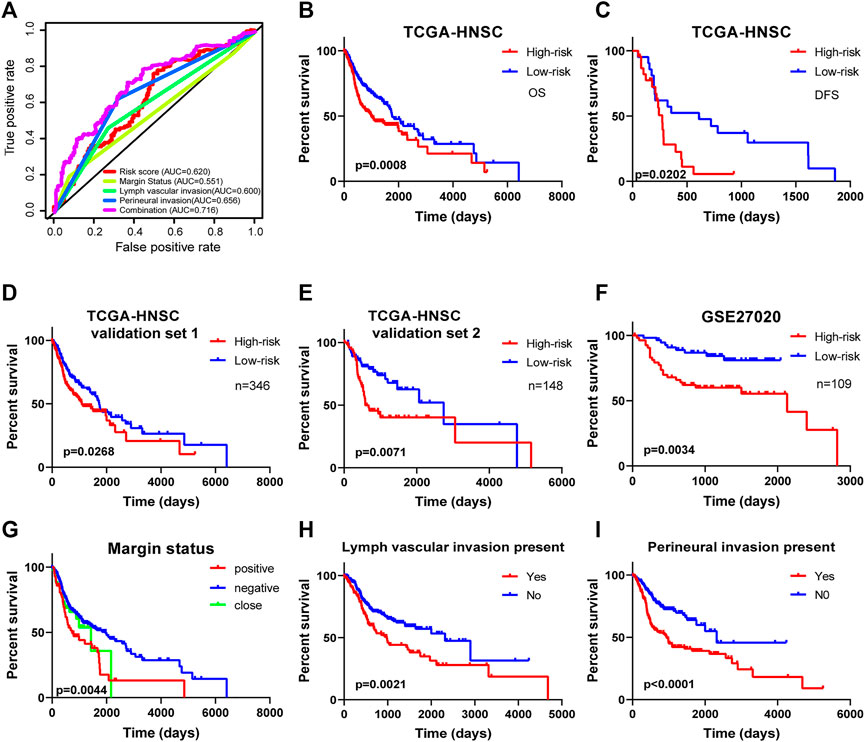
FIGURE 4. Kaplan-Meier survival analysis for patients with HNSCC stratified by the prognostic signature. (A) The receiver operating characteristic (ROC) for EMT-based prognostic signature, margin status, lymph node vascular invasion and perineural invasion only, and the EMT-based risk score combined with the above clinical parameters for OS of HNSCC patients. (B) The Kaplan-Meier curve of OS for patients in TCGA-HNSCC cohort divided into high-risk and low-risk groups. (C) The Kaplan-Meier curve of DFS for patients in TCGA-HNSCC cohort divided into high-risk and low-risk groups. (D) The Kaplan-Meier curve of prognostic signature for HNSCC patients in TCGA validation set 1 (n = 346). (E) The Kaplan-Meier curve of prognostic signature for HNSCC patients in TCGA validation set 2 (n = 148). (F) The Kaplan-Meier curve of OS in the high- and low-risk groups stratified by the four-mRNA signature in the GSE27020. Different clinical features including (G) margin status, (H) lymph vascular invasion, and (I) presence of perineural invasion predicts patient survival.
Kaplan-Meier Curves for Survival Predicted Four-mRNA Signature
Kaplan-Meier curves and the log-rank method showed that patients with high-risk scores had poorer OS time (p = 0.0008; Figure 4B). Meanwhile, the signature showed great utility in predicting DFS with p-value of 0.0202 (Figure 4C). Additionally, in TCGA validation set 1 (n = 346) and validation set 2 (n = 148), the Kaplan-Meier curves displayed significant differences between high-risk and low-risk patients (p < 0.05, Figures 4D,E). We also extracted HNSCC afflicted patients from the GSE27020 dataset (n = 109) and applied the same formula to validate the ability of the four-EMT-based classifier predicting OS of HNSCC. As expected, patients in the low-risk group had a significantly longer survival time compared to the high-risk group (p = 0.0034; Figure 4F). Univariate Cox regression analysis of OS showed that several clinicopathological parameters were effective predictors of HNSCC survival, including clinical N, clinical M, margin status, lymphangitic invasion and perineural infiltration. Then Kaplan-Meier curve was used to verify the above conclusion, and the results were self-consistent (Figures 4G–I). According to the curve, patients with lymphangitic invasions and peripheral nerve invasions had a poor prognosis during follow-up.
Stratified analysis for further data mining. As shown in Kaplan-Meier curve, regardless of age (<61 or ≥61), four-mRNA signature in HNSCC patients are stable prognostic markers, due to the poor prognosis of patients with a high-risk score (Figure 5A). Additionally, when patients were stratified into different subgroups based on gender and HPV P16 status the four-mRNA risk score remained an independent prognostic indicator for male HPV P16-negative (Figures 5B,C), suggesting that HNSCC may require further investigation. Similarly, when patients underwent radiation therapy during follow-up (with positive alcohol status and absence of perineural invasion), we could use risk scores to predict patient results, which showed that patients in the high-risk subgroups had poor survival (Figures 5D,E,G,H).
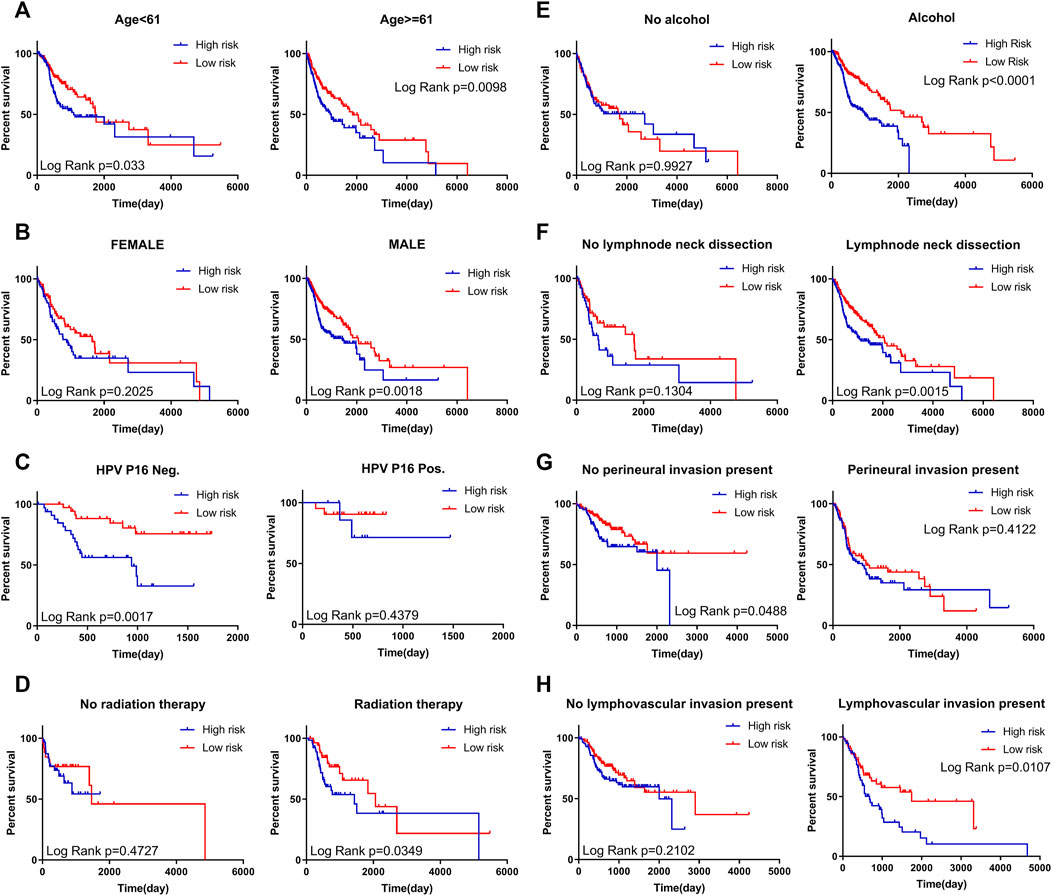
FIGURE 5. Kaplan–Meier curves for prognostic value of risk score signature for patients divided by each clinical feature. (A) Age (B) Gender (C) Expression status of HPV P16 (D) Status of radiation therapy (E) Alcohol status (F) Status of lymph node neck dissection (G) Presence of perineural invasion (H) Presence of lymph vascular invasion.
Functional Enrichment Analysis of the Four-mRNA Signature in HNSCC
We performed enrichment analysis to elucidate the biological function of the four-mRNA signature target genes. The GO categories comprised of three structured networks: biological processes (BP), cellular components (CC) and molecular function (MF). The top ten enriched functional analysis is shown in Figure 6. The top enriched biological process was proteolysis (associated with 10 genes); the top enriched cellular component and molecular function were integral component of membrane part (associated with 53 genes) and calcium ion binding (associated with 14 genes), respectively. Therefore, a total of 12 KEGG pathways were enriched by the four-mRNA signature. The significantly enriched KEGG pathways were the cancer-related pathways (associated with seven genes), cytokine-cytokine receptor interaction pathways (associated with seven genes) and neuroactive ligand-receptor interaction pathways (associated with seven genes).
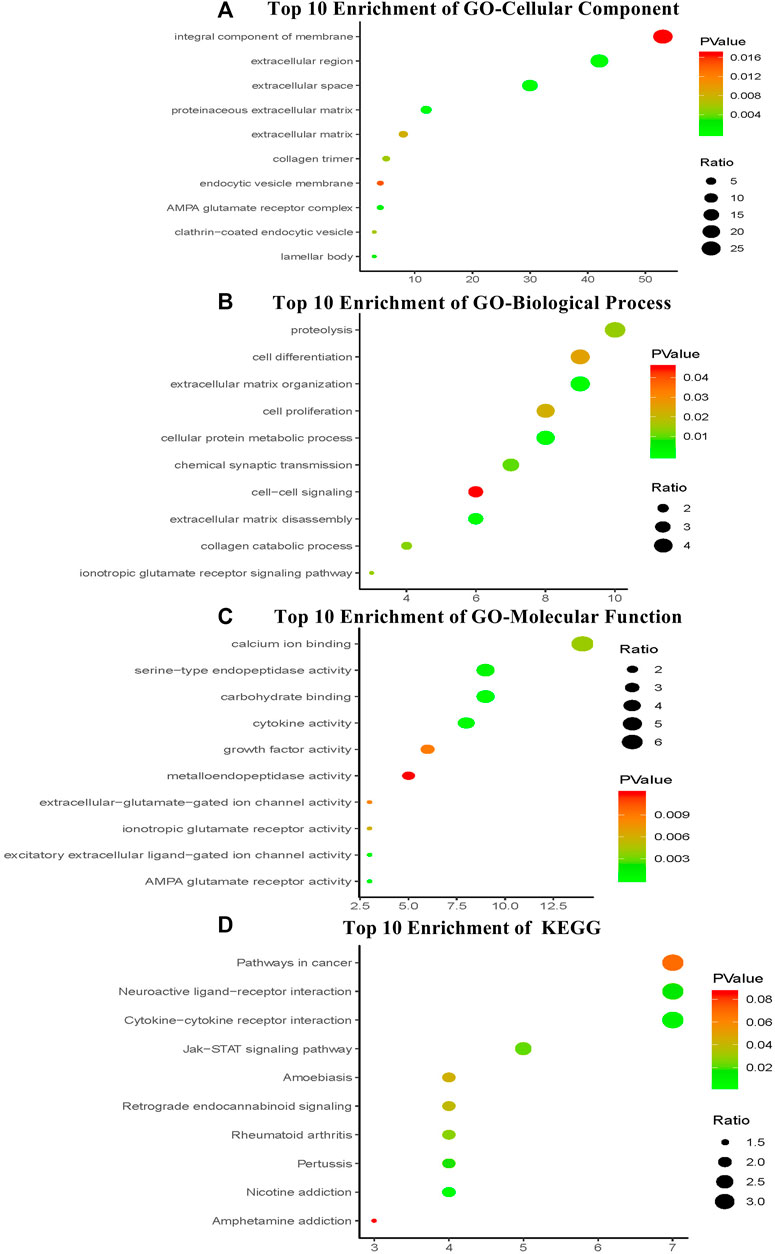
FIGURE 6. The top ten of GO term and pathway by target genes of four-mRNA signature in TCGA HNSCC cohort. The analysis was considered significant when P values were corrected for false discovery rate (FDR) (A) Parts of GO-CC categories of four-mRNA signature (B) Parts of GO-BP categories of four-mRNA signature (C) Parts of GO-MF categories of four-mRNA signature (D) Parts of KEGG pathway of four-mRNA signature.
Discussion
Recent studies have shown that clinicopathological features such as gender, age, tumor margin status, and metastasis are not sufficient for accurately predict the prognosis of patients. As a result, more and more mRNAs might be examined as molecular markers at an increasing rate to predict cancer development and prognosis, indicating that its clinical significance needs to be explored [18]. For example, analysis of the prognostic value of a single lncRNA from qRT-PCR array of 84 gastric cancer patient samples found that higher level of BANCR could predict a poor prognosis for GC patients [19]. The high expression of FAM83H-AS1 is involved in the progression of bladder cancer and serves as a prognostic biomarker and potential therapeutic target for patients with bladder cancer [20]. Compared to single biomarker, integrating multiple biomarkers into the aggregation model could improve the prognostic value [21]. In particular, the expression of a single gene could be controlled by a variety of factors, and hence might not provide a strong predictive effect. Therefore, a statistical model was constructed gene signature contains multiple genes, combined with the effect of each component gene prediction, improve forecasting efficiency. This model has been used widely, and is superior to single biomarkers in predicting disease prognosis [22–24].
With the development of high-throughput genetic testing technology, we are entering a new era of big biological data [25]. Currently, RNA sequencing or micro array data for gene mutations and expression levels often use Cox proportional hazard regression models to construct new prognostic features [26, 27]. In this study, we attempted to apply GSEA using expression data of 57,072 genes of patients from TCGA-HNSCC cohort, and we found significant differences in five biological functions with p-values < 0.05. As mentioned above, we focused on EMT, using GSEA to select genes to predict patient survival rather than exploring a broad range of genes. Univariate and multivariate Cox regression analyses were performed to identify combinations of four genes with predictive values for HNSCC patients, rather than just one gene [21]. Compared to some known predictive biomarkers, the risk profile of this option may have a more targeted and prognostic ability to support positive clinical results and be an effective classification tool for HNSCC patients. In addition, this study used bioinformatics methods to explore the risk characteristics of mRNA and its clinical significance, providing a novel method for mining potential prognostic markers, which not only supports our previous understanding of HNSCC, but also lays the foundation for future studies. It should be noted that, Kaplan-Meier analysis showed that risk score on survival is small and appears even smaller than lymph vascular invasion or perineural invasion. Therefore, more comprehensive and homogeneous datasets are needed to assess the four-mRNA signature before clinical applications. Notably, the stratification analysis found that the four-mRNA signature could generate superior performances for predicting the survival benefit in patients with P16 negative or alcohol or age <61, indicating that the four-mRNA signature could be helpful in predicting HNSCC prognosis, especially in P16 negative or alcohol or age <61 patients. It is highly significant given that P16 positive tumors typically have a good prognosis regardless of stage or high-risk pathologic features, this prognostic mRNA signature classifier for P16 negative-related HNSCC may help clinicians to pinpoint those HNSCC patients at high risk of unfavorable OS. EMT is recognized to be important in cancer cell migration/metastasis. For example, to metastasize, disseminated mesenchyme-like tumor cells of well-differentiated carcinomas must regain their epithelial function (invasion and metastasis) [28, 29]. The disruption of epithelial-cell homeostasis leading to aggressive cancer progression is correlated with the loss of epithelial characteristics and the acquisition of a migratory phenotype, known to EMT, and is considered to be a crucial event in malignancy [30, 31]. EMT and mesenchymal-related gene expression are associated with aggressive breast cancer subtypes and poor clinical outcome in breast cancer patients [32, 33]. Recently, some studies have reported the occurrence and development of gene expression induced EMT and metastasis in HNSCC [34, 35]. Additionally, several studies have been conducted which have predicted that in HNSCC patients, survival is associated with EMT. For instance, TEAD4 might act as a putative oncogenic gene by enhancing the proliferation, migration, and invasion of HNSCC cancer cells [36]. However, the EMT gene signature used to predict HNSCC prognosis have not been established. In the present study, we reported an EMT gene signature of four genes (PPIB, BASP1, WIPF1 and PLOD2), identified using bioinformatics methods which demonstrate the prognostic value of HNSCC. Of the four candidate genes, three have positively correlated coefficients in the prognostic model and correlated with poor survival. PPIB, called cyclophilin B, regulates the protein conformation of its substrate through prolyl cis–trans-isomerization in endoplasmic reticulum (ER) lumen and nucleus, and possesses multiple functions, including chemotaxis and prolactin signaling [37–39]. Additionally, it is a novel wild-type p53 (p53WT)-inducible gene [40]. In cancer biology, PPIB is associated with malignant progression and regulation of genes involved in the pathogenesis of gastric cancer [41],hepatocellular carcinoma [42], pancreatic cancer [43] and is considered as a candidate biomarker for these cancers. However, the exact molecular mechanism by which PPIB leads to cancer cell survival is unclear. BASP1 (brain acid soluble protein 1), was initially isolated from 23,000 brain extracts. BASP1 comprises an effector domain which dynamically couples to the plasma membrane and is also involved in neuronal sprouting process [44]. Recently, some reports have identified BASP1 as a transcriptional co-suppressor for WT1 protein [45]. WT1 can promote tumor cell proliferation, inhibition of apoptosis, and interacts with cytoskeletal proteins to promote migration, invasion and angiogenesis [46]. WT1 gene is expressed highly abnormally in a variety of tumors, including breast [47], thyroid [48, 49], non-small cell lung cancers [50], and HNSCC [51], and is considered to have the characteristics of an oncogene. PLOD2, also called LH2, is the key enzyme mediating the formation of stabilized collagen cross-links [52]. Gain and loss of function studies show that LH2 hydroxylated telopeptide lysine residues on collagen, shifted the tumor stroma toward higher levels of hydroxylysine aldehyde–derived collagen cross-links (HLCCs), lower levels of lysine aldehyde–derived cross-links (LCCs), increased tumor stiffness, and enhanced tumor cell invasion and metastasis [53, 54]. PLOD2 is overexpressed in different cancers such as bladder cancer [55], renal cell carcinoma [56], and oral carcinoma [57], and is closely associated with poor prognosis [58]. All these three genes are associated with EMT in cancer. In contrast, WIPF1 confers an onco-protective effect. WIPF1, also known as WIP, the WASP-interacting protein (WIP) drives the oncogenic activity of mutant p53. WIPF1 knockdown in glioblastoma and breast cancer cells expressing mtp53 greatly reduced the proliferation and growth capacity of cancer stem cell-like cells and reduced the expression of cancer stem cell-like markers (CD44, CD133 or TAZ/YAP). WIPF1 knockdown inhibits the growth of glioblastoma tumor cells and breast cancer cells in vivo [59]. Since EMT is affected in HNSCC, the targeted genes might be useful in controlling cancer. Finally, the prognostic value of the four genes in HNSCC still needs further experimental studies to elucidate biological function.
Conclusion
In conclusion, we identified and verified a four-gene risk signature related to EMT that can predict the survival of HNSCC patients, wherein a high-risk score shows poor patient prognosis, suggesting that this gene signature could be a potential new biomarker for HNSCC prognosis. Further investigation of these genes will provide theoretical guidance for basic research and for the clinical treatment of HNSCC.
Data Availability Statement
The original contributions presented in the study are included in the article/Supplementary Material, further inquiries can be directed to the corresponding authors.
Author Contributions
WX, CZ, LJ, DP, LZ, CZ Writing-original draft preparation: WX; Methodology: CZ and LJ. Formal analysis and investigation: DP; Writing-review and editing: LZ and CZ; All authors critically reviewed the manuscript in its entirety and approved the final content.
Funding
National Natural Science Foundation of China and Liaoning joint fund key program (No. U1608281), Liaoning Revitalization Talents Program (No. XLYC1807201), the Key Laboratory Foundation from Shenyang S&T Projects (F16-094-1-00) and Shenyang S&T Projects (17-123-9-00, Z18-4-020).
Conflict of Interest
The authors declare that the research was conducted in the absence of any commercial or financial relationships that could be construed as a potential conflict of interest.
Supplementary Material
The Supplementary Material for this article can be found online at: https://www.por-journal.com/articles/10.3389/pore.2021.585192/full#supplementary-material.
References
1. Siegel, RL, Miller, KD, and Jemal, A. Cancer statistics, 2016. CA: A Cancer J Clinicians (2016). 66(1):7–30. doi:10.3322/caac.21332
2. García-Carracedo, D, Villaronga, MÁ, Álvarez-Teijeiro, S, Hermida-Prado, F, Santamaría, I, Allonca, E, et al. Impact of PI3K/AKT/mTOR pathway activation on the prognosis of patients with head and neck squamous cell carcinomas. Oncotarget (2016). 7(20):29780–93. doi:10.18632/oncotarget.8957
3. Leemans, CR, Braakhuis, BJM, and Brakenhoff, RH. The molecular biology of head and neck cancer. Nat Rev Cancer (2011). 11(1):9–22. doi:10.1038/nrc2982
4. Patel, SG, and Shah, JP. TNM staging of cancers of the head and neck: striving for uniformity among diversity. CA: A Cancer J Clinicians (2005). 55(4):242–58. doi:10.3322/canjclin.55.4.242
5. Wyss, A, Hashibe, M, Chuang, S-C, Lee, Y-CA, Zhang, Z-F, Yu, G-P, et al. Cigarette, cigar, and pipe smoking and the risk of head and neck cancers: pooled analysis in the International Head and Neck Cancer Epidemiology Consortium. Am J Epidemiol (2013). 178(5):679–90. doi:10.1093/aje/kwt029
6. Ang, KK, Harris, J, Wheeler, R, Weber, R, Rosenthal, DI, Nguyen-Tân, PF, et al. Human papillomavirus and survival of patients with oropharyngeal cancer. N Engl J Med (2010). 363(1):24–35. doi:10.1056/nejmoa0912217
7. Xiang, Y, Li, F, Wang, L, Zheng, A, Zuo, J, Li, M, et al. Decreased calpain 6 expression is associated with tumorigenesis and poor prognosis in HNSCC. Oncol Lett (2017). 13(4):2237–43. doi:10.3892/ol.2017.5687
8. Wang, Y, Liu, Y, Liu, H, Zhang, Q, Song, H, Tang, J, et al. FcGBP was upregulated by HPV infection and correlated to longer survival time of HNSCC patients. Oncotarget (2017). 8(49):86503–14. doi:10.18632/oncotarget.21220
9. Chen, Y-L, Ge, G-J, Qi, C, Wang, H, Wang, H-L, Li, L-Y, et al. A five-gene signature may predict sunitinib sensitivity and serve as prognostic biomarkers for renal cell carcinoma. J Cel Physiol (2018). 233(10):6649–60. doi:10.1002/jcp.26441
10. Cheng, W, Ren, X, Zhang, C, Cai, J, Liu, Y, Han, S, et al. Bioinformatic profiling identifies an immune-related risk signature for glioblastoma. Neurology (2016). 86(24):2226–34. doi:10.1212/wnl.0000000000002770
11. Yeung, KT, and Yang, J. Epithelial-mesenchymal transition in tumor metastasis. Mol Oncol (2017). 11(1):28–39. doi:10.1002/1878-0261.12017
12. Puram, SV, Tirosh, I, Parikh, AS, Patel, AP, Yizhak, K, Gillespie, S, et al. Single-cell transcriptomic analysis of primary and metastatic tumor ecosystems in head and neck cancer. Cell (2017). 171(7):1611–24. e24. doi:10.1016/j.cell.2017.10.044
13. Puram, SV, Parikh, AS, and Tirosh, I. Single cell RNA-seq highlights a role for a partial EMT in head and neck cancer. Mol Cell Oncol (2018). 5(3):e1448244. doi:10.1080/23723556.2018.1448244
14. Thomas, MA, Yang, L, Carter, BJ, and Klaper, RD. Gene set enrichment analysis of microarray data from Pimephales promelas (Rafinesque), a non-mammalian model organism. BMC Genomics (2011). 12:66. doi:10.1186/1471-2164-12-66
15. Subramanian, A, Tamayo, P, Mootha, VK, Mukherjee, S, Ebert, BL, Gillette, MA, et al. Gene set enrichment analysis: a knowledge-based approach for interpreting genome-wide expression profiles. Proc Natl Acad Sci (2005). 102(43):15545–50. doi:10.1073/pnas.0506580102
16. Cheng, W, Li, M, Cai, J, Wang, K, Zhang, C, Bao, Z, et al. HDAC4, a prognostic and chromosomal instability marker, refines the predictive value of MGMT promoter methylation. J Neurooncol (2015). 122(2):303–12. doi:10.1007/s11060-014-1709-6
17. Huang, DW, Sherman, BT, and Lempicki, RA. Systematic and integrative analysis of large gene lists using DAVID bioinformatics resources. Nat Protoc (2009). 4(1):44–57. doi:10.1038/nprot.2008.211
18. Guo, W, Chen, X, Zhu, L, and Wang, Q. A six-mRNA signature model for the prognosis of head and neck squamous cell carcinoma. Oncotarget (2017). 8(55):94528–38. doi:10.18632/oncotarget.21786
19. Li, L, Zhang, L, Zhang, Y, and Zhou, F. Increased expression of LncRNA BANCR is associated with clinical progression and poor prognosis in gastric cancer. Biomed Pharmacother (2015). 72:109–12. doi:10.1016/j.biopha.2015.04.007
20. Shan, H, Yang, Y, Zhu, X, Han, X, Zhang, P, and Zhang, X. FAM83H-AS1 is associated with clinical progression and modulates cell proliferation, migration, and invasion in bladder cancer. J Cel Biochem (2018). 120(3):4687–93. doi:10.1002/jcb.27758
21. Ng, SWK, Mitchell, A, Kennedy, JA, Chen, WC, McLeod, J, Ibrahimova, N, et al. A 17-gene stemness score for rapid determination of risk in acute leukaemia. Nature (2016). 540(7633):433–7. doi:10.1038/nature20598
22. Bao, Z-S, Li, M-Y, Wang, J-Y, Zhang, C-B, Wang, H-J, Yan, W, et al. Prognostic value of a nine-gene signature in glioma patients based on mRNA expression profiling. CNS Neurosci Ther (2014). 20(2):112–8. doi:10.1111/cns.12171
23. Niyazi, M, Pitea, A, Mittelbronn, M, Steinbach, J, Sticht, C, Zehentmayr, F, et al. A 4-miRNA signature predicts the therapeutic outcome of glioblastoma. Oncotarget (2016). 7(29):45764–75. doi:10.18632/oncotarget.9945
24. Cheng, W, Ren, X, Cai, J, Zhang, C, Li, M, Wang, K, et al. A five-miRNA signature with prognostic and predictive value for MGMT promoter-methylated glioblastoma patients. Oncotarget (2015). 6(30):29285–95. doi:10.18632/oncotarget.4978
25. Chen, P-f., Wang, F, Zhang, Z-x., Nie, J-y., Liu, L, Feng, J-r., et al. A novel gene-pair signature for relapse-free survival prediction in colon cancer. Cmar (2018). 10:4145–53. doi:10.2147/cmar.s176260
26. Peng, P-L, Zhou, X-Y, Yi, G-D, Chen, P-F, Wang, F, and Dong, W-G. Identification of a novel gene pairs signature in the prognosis of gastric cancer. Cancer Med (2018). 7(2):344–50. doi:10.1002/cam4.1303
27. Yan, H, Xin, S, Ma, J, Wang, H, Zhang, H, and Liu, J. A three microRNA-based prognostic signature for small cell lung cancer overall survival. J Cel Biochem (2018). 120(5):8723–30. doi:10.1002/jcb.28159
28. Brabletz, T, Jung, A, Reu, S, Porzner, M, Hlubek, F, Kunz-Schughart, LA, et al. Variable -catenin expression in colorectal cancers indicates tumor progression driven by the tumor environment. Proc Natl Acad Sci (2001). 98(18):10356–61. doi:10.1073/pnas.171610498
29. Baek, T-H, Kang, D-W, Kim, J-H, and Son, H-J. Gland attenuation, a novel morphological feature of colorectal cancer: evidence for an epithelial-mesenchymal transition. Ann Coloproctol (2018). 34(4):187–96. doi:10.3393/ac.2017.12.02
30. Thiery, JP. Epithelial-mesenchymal transitions in development and pathologies. Curr Opin Cel Biol (2003). 15(6):740–6. doi:10.1016/j.ceb.2003.10.006
31. Brabletz, T, Jung, A, Spaderna, S, Hlubek, F, and Kirchner, T. Migrating cancer stem cells - an integrated concept of malignant tumour progression. Nat Rev Cancer (2005). 5(9):744–9. doi:10.1038/nrc1694
32. Hollier, BG, Evans, K, and Mani, SA. The epithelial-to-mesenchymal transition and cancer stem cells: a coalition against cancer therapies. J Mammary Gland Biol Neoplasia (2009). 14(1):29–43. doi:10.1007/s10911-009-9110-3
33. Blick, T, Widodo, E, Hugo, H, Waltham, M, Lenburg, ME, Neve, RM, et al. Epithelial mesenchymal transition traits in human breast cancer cell lines. Clin Exp Metastasis (2008). 25(6):629–42. doi:10.1007/s10585-008-9170-6
34. Wu, D, Cheng, J, Sun, G, Wu, S, Li, M, Gao, Z, et al. p70S6K promotes IL-6-induced epithelial-mesenchymal transition and metastasis of head and neck squamous cell carcinoma. Oncotarget (2016). 7(24):36539–50. doi:10.18632/oncotarget.9282
35. Ock, C-Y, Kim, S, Keam, B, Kim, M, Kim, TM, Kim, J-H, et al. PD-L1 expression is associated with epithelial-mesenchymal transition in head and neck squamous cell carcinoma. Oncotarget (2016). 7(13):15901–14. doi:10.18632/oncotarget.7431
36. Zhang, W, Li, J, Wu, Y, Ge, H, Song, Y, Wang, D, et al. TEAD4 overexpression promotes epithelial-mesenchymal transition and associates with aggressiveness and adverse prognosis in head neck squamous cell carcinoma. Cancer Cel Int (2018). 18:178. doi:10.1186/s12935-018-0675-z
37. Obata, Y, Yamamoto, K, Miyazaki, M, Shimotohno, K, Kohno, S, and Matsuyama, T. Role of cyclophilin B in activation of interferon regulatory factor-3. J Biol Chem (2005). 280(18):18355–60. doi:10.1074/jbc.m501684200
38. Rycyzyn, MA, and Clevenger, CV. The intranuclear prolactin/cyclophilin B complex as a transcriptional inducer. Proc Natl Acad Sci (2002). 99(10):6790–5. doi:10.1073/pnas.092160699
39. Bukrinsky, MI. Cyclophilins: unexpected messengers in intercellular communications. Trends Immunol (2002). 23(7):323–5. doi:10.1016/s1471-4906(02)02237-8
40. Choi, TG, Nguyen, MN, Kim, J, Jo, YH, Jang, M, Nguyen, NNY, et al. Cyclophilin B induces chemoresistance by degrading wild-type p53 via interaction with MDM2 in colorectal cancer. J Pathol (2018). 246(1):115–26. doi:10.1002/path.5107
41. Meng, DQ, Li, PL, and Xie, M. Expression and role of cyclophilin B in stomach cancer. Genet Mol Res (2015). 14(2):5346–54. doi:10.4238/2015.may.22.5
42. Kim, Y, Jang, M, Lim, S, Won, H, Yoon, K-S, Park, J-H, et al. Role of cyclophilin B in tumorigenesis and cisplatin resistance in hepatocellular carcinoma in humans. Hepatology (2011). 54(5):1661–78. doi:10.1002/hep.24539
43. Ray, P, Rialon-Guevara, KL, Veras, E, Sullenger, BA, and White, RR. Comparing human pancreatic cell secretomes by in vitro aptamer selection identifies cyclophilin B as a candidate pancreatic cancer biomarker. J Clin Invest (2012). 122(5):1734–41. doi:10.1172/jci62385
44. Toska, E, Shandilya, J, Goodfellow, SJ, Medler, KF, and Roberts, SGE. Prohibitin is required for transcriptional repression by the WT1-BASP1 complex. Oncogene (2014). 33(43):5100–8. doi:10.1038/onc.2013.447
45. Carpenter, B, Hill, KJ, Charalambous, M, Wagner, KJ, Lahiri, D, James, DI, et al. BASP1 is a transcriptional cosuppressor for the Wilms' tumor suppressor protein WT1. Mcb (2004). 24(2):537–49. doi:10.1128/mcb.24.2.537-549.2004
46. Sugiyama, H. WT1 (Wilms' tumor gene 1): biology and cancer immunotherapy. Jpn J Clin Oncol (2010). 40(5):377–87. doi:10.1093/jjco/hyp194
47. Qi, X-W, Zhang, F, Yang, X-H, Fan, L-J, Zhang, Y, Liang, Y, et al. High Wilms' tumor 1 mRNA expression correlates with basal-like and ERBB2 molecular subtypes and poor prognosis of breast cancer. Oncol Rep (2012). 28(4):1231–6. doi:10.3892/or.2012.1906
48. Oji, Y, Miyoshi, Y, Koga, S, Nakano, Y, Ando, A, Nakatsuka, S-i., et al. Overexpression of the Wilms' tumor gene WT1 in primary thyroid cancer. Cancer Sci (2003). 94(7):606–11. doi:10.1111/j.1349-7006.2003.tb01490.x
49. Guo, R-S, Yu, Y, Chen, J, Chen, Y-Y, Shen, N, and Qiu, M. Restoration of brain acid soluble protein 1 inhibits proliferation and migration of thyroid cancer cells. Chin Med J (Engl) (2016). 129(12):1439–46. doi:10.4103/0366-6999.183434
50. Wu, C, Wang, Y, Xia, Y, He, S, Wang, Z, Chen, Y, et al. Wilms' tumor 1 enhances Cisplatin-resistance of advanced NSCLC. FEBS Lett (2014). 588(24):4566–72. doi:10.1016/j.febslet.2014.10.026
51. Li, X, Ottosson, S, Wang, S, Jernberg, E, Boldrup, L, Gu, X, et al. Wilms' tumor gene 1 regulates p63 and promotes cell proliferation in squamous cell carcinoma of the head and neck. BMC Cancer (2015). 15:342. doi:10.1186/s12885-015-1356-0
52. Yamauchi, M, and Sricholpech, M. Lysine post-translational modifications of collagen. Essays Biochem (2012). 52:113–33. doi:10.1042/bse0520113
53. Provenzano, PP, Eliceiri, KW, Campbell, JM, Inman, DR, White, JG, and Keely, PJ. Collagen reorganization at the tumor-stromal interface facilitates local invasion. BMC Med (2006). 4(1):38. doi:10.1186/1741-7015-4-38
54. Chen, Y, Guo, H, Terajima, M, Banerjee, P, Liu, X, Yu, J, et al. Lysyl hydroxylase 2 is secreted by tumor cells and can modify collagen in the extracellular space. J Biol Chem (2016). 291(50):25799–808. doi:10.1074/jbc.m116.759803
55. Miyamoto, K, Seki, N, Matsushita, R, Yonemori, M, Yoshino, H, Nakagawa, M, et al. Tumour-suppressive miRNA-26a-5p and miR-26b-5p inhibit cell aggressiveness by regulating PLOD2 in bladder cancer. Br J Cancer (2016). 115(3):354–63. doi:10.1038/bjc.2016.179
56. Kurozumi, A, Kato, M, Goto, Y, Matsushita, R, Nishikawa, R, Okato, A, et al. Regulation of the collagen cross-linking enzymes LOXL2 and PLOD2 by tumor-suppressive microRNA-26a/b in renal cell carcinoma. Int J Oncol (2016). 48(5):1837–46. doi:10.3892/ijo.2016.3440
57. Reis, PP, Waldron, L, Goswami, RS, Xu, W, Xuan, Y, Perez-Ordonez, B, et al. mRNA transcript quantification in archival samples using multiplexed, color-coded probes. BMC Biotechnol (2011). 11:46. doi:10.1186/1472-6750-11-46
58. Du, H, Pang, M, Hou, X, Yuan, S, and Sun, L. PLOD2 in cancer research. Biomed Pharmacother (2017). 90:670–6. doi:10.1016/j.biopha.2017.04.023
Keywords: epithelial-mesenchymal transition, HNSCC, mRNAs, Prognostic, survival
Citation: Xin W, Zhao C, Jiang L, Pei D, Zhao L and Zhang C (2021) Identification of a Novel Epithelial–Mesenchymal Transition Gene Signature Predicting Survival in Patients With HNSCC. Pathol. Oncol. Res. 27:585192. doi: 10.3389/pore.2021.585192
Received: 20 July 2020; Accepted: 15 February 2021;
Published: 29 March 2021.
Edited by:
József Tímár, Semmelweis University, HungaryCopyright © 2021 Xin, Zhao, Jiang, Pei, Zhao and Zhang. This is an open-access article distributed under the terms of the Creative Commons Attribution License (CC BY). The use, distribution or reproduction in other forums is permitted, provided the original author(s) and the copyright owner(s) are credited and that the original publication in this journal is cited, in accordance with accepted academic practice. No use, distribution or reproduction is permitted which does not comply with these terms.
*Correspondence: Chengpu Zhang, emhhbmdjcEBzai1ob3NwaXRhbC5vcmc=; Lin Zhao, emxfY211QDE2My5jb20=