- 1Department of Chemotherapy, Guangxi Medical University Cancer Hospital, Nanning, China
- 2Department of Medical Oncology, Fujian Medical University Union Hospital, Fuzhou, China
- 3Department of Gastroenterology, Jiangbin Hospital, Nanning, China
- 4Department of Medical Oncology, Second Affiliated Hospital of Guangxi Medical University, Nanning, China
Background: This study aimed to explore the relationship between MALAT1 and the prognosis of patients with hepatocellular carcinoma (HCC).
Methods: We constructed a MALAT1 protein-protein interaction network using the STRING database and a network of competing endogenous RNAs (ceRNAs) using the StarBase database. Using data from the GEPIA2 database, we studied the association between genes in these networks and survival of patients with HCC. The potential mechanisms underlying the relationship between MALAT1 and HCC prognosis were studied using combined data from RNA sequencing, DNA methylation, and somatic mutation data from The Cancer Genome Atlas (TCGA) liver cancer cohort. Tumor tissues and 19 paired adjacent non-tumor tissues (PANTs) from HCC patients who underwent radical resection were analyzed for MALAT1 mRNA levels using real-time PCR, and associations of MALAT1 expression with clinicopathological features or prognosis of patients were analyzed using log-rank test and Gehan-Breslow-Wilcoxon test.
Results: Five interacting proteins and five target genes of MALAT1 in the ceRNA network significantly correlated with poor survival of patients with HCC (p < 0.05). High MALAT1 expression was associated with mutations in two genes leading to poor prognosis and may upregulate some prognostic risk genes through methylation. MALAT1 was significantly co-expressed with various signatures of genes involved in HCC progression, including the cell cycle, DNA damage repair, mismatch repair, homologous recombination, molecular cancer m6A, exosome, ferroptosis, infiltration of lymphocyte (p < 0.05). The expression of MALAT1 was markedly upregulated in HCC tissues compared with PANTs. In Kaplan-Meier analysis, patients with high MALAT1 expression had significantly shorter progression-free survival (PFS) (p = 0.033) and overall survival (OS) (p = 0.023) than those with low MALAT1 expression. Median PFS was 19.2 months for patients with high MALAT1 expression and 52.8 months for patients with low expression, while the corresponding median OS was 40.5 and 78.3 months. In subgroup analysis of patients with vascular invasion, cirrhosis, and HBsAg positive or AFP positive, MALAT1 overexpression was significantly associated with shorter PFS and OS. Models for predicting PFS and OS constructed based on MALAT1 expression and clinicopathological features had moderate predictive power, with areas under the receiver operating characteristic curves of 0.661–0.731. Additionally, MALAT1 expression level was significantly associated with liver cirrhosis, vascular invasion, and tumor capsular infiltration (p < 0.05 for all).
Conclusion: MALAT1 is overexpressed in HCC, and higher expression is associated with worse prognosis. MALAT1 mRNA level may serve as a prognostic marker for patients with HCC after hepatectomy.
Introduction
Hepatocellular carcinoma (HCC) is the most frequent subtype of primary liver cancer and a common malignancy. HCC has been rising in incidence in the last decades and is a major cancer-related cause of death among men in China (1, 2). Non-curative therapies such as transcatheter arterial chemoembolization (TACE) and stereotactic radiotherapy (SBRT) can prolong the survival of patients with advanced HCC by slowing tumor progression (3, 4), while the combination of angiogenesis and immune checkpoint inhibitors can prolong progression-free survival (PFS) (5, 6).
Patients with early disease can benefit from curative treatments such as radical resection, liver transplantation (LT), and ablative techniques (7–9), but 5-year recurrence rates after such procedures are 40%–70% (8). A growing body of studies has focused on screening and clinical application of biomarkers for prognostic monitoring, but the sensitivity and specificity of traditional prognostic indicators such as alpha-fetoprotein (AFP) are inadequate on their own to guide clinical decisions (9). Emerging markers based on histology and genomics may more comprehensively reflect the biological characteristics of cancer cells and therefore support individualized diagnosis and treatment of HCC (10).
Genomic and transcriptomic sequencing studies suggest that long non-coding RNAs (lncRNAs) regulate many of the characteristics of HCC, including proliferation, invasion, metastasis, and immunosuppression (11, 12). These RNAs more than 200 nucleotides long are not translated into proteins but can regulate gene expression at the levels of transcription, post-transcriptional processing, and translation (13). In addition, lncRNAs may compete with microRNAs (miRNAs) to bind to the same target mRNA in order to regulate its translation (14). In this case, the lncRNA is referred to as a competing endogenous RNA (ceRNA). LncRNAs can also regulate DNA methylation to influence cancer (15, 16).
Recently, studies have shown that the 8-kb lncRNA MALAT1 could be linked to other cancers, including breast cancer, prostate cancer, pancreatic cancer, glioma, and leukemia. (17). Therefore, MALAT1 may be a biomarker for early diagnosis, severity assessment, or prognostic evaluation in HCC (18). In this study, we analyzed MALAT1 expression at the multi-omics level and identified associations of its expression with gene transcription, DNA methylation, and gene mutations, and we explored the potential influence of these associations on prognosis. Second, we investigated the relationships of MALAT1 expression with clinicopathological features and prognosis of HCC patients after hepatectomy.
Methods
Public data preparation and preprocessing
RNA-sequencing (RNA-seq) counts and tumor data for 368 patients in The Cancer Genome Atlas liver hepatocellular carcinoma (TCGA-LIHC) cohort were downloaded using UCSCXenaTools (19). Counts were normalized using the transcript per million (TPM) method, and genes with expression levels below the lower end of the interquartile range (IQR) of expression across all genes in the sample were excluded. Samples with low-frequency counts (the number of occasions of “count-per-million values > 0.5” in the sample less than 2) were excluded.
We also downloaded somatic mutation and DNA methylation profiling data for 364 and 430 patients, respectively, in the TCGA-LIHC cohort using UCSCXenaTools.
Interacting protein analysis
We constructed the network of MALAT1 with interacting proteins using text mining in the STRING database. A first shell was created with no more than 10 interactors, and a second shell with no more than 20 interactors. Enrichment of terms from the Kyoto Encyclopedia of Genes and Genomes (KEGG) in the network were visualized using the GOplot package in R. Genes encoding first-shell proteins were analyzed for potential assocations with HCC patient survival using the GEPIA2 database (http://gepia2.cancer-pku.cn/).
Construction of ceRNA network
StarBase is an online database that can build ceRNA networks based on thousands of interactions between miRNAs and their target genes in a CLIP-seq (Crosslinking or RNA immunoprecipitation followed by sequencing) database (https://starbase.sysu.edu.cn/). We obtained potential MALAT1 target miRNAs and genes that may compete with MALAT1 for binding miRNAs through StarBase (screening by miRNA number ≥ 30 and p-value ≤ 1.0e-5). In this way, a network of ceRNAs was constructed and visualized by Cytoscape. Genes regulated by MALAT1 and miRNAs were analyzed for their potential associations with survival of HCC patients using the GEPIA2 database.
Somatic mutation data analysis
Somatic mutations were analyzed using the maftools package in R (4.1.0). We selected samples tested for both expression and mutation, then selected 84 samples whose MALAT1 expression was below the lower end of the IQR and 85 samples whose MALAT1 expression exceeded the upper end of the IQR. We summarized the overall mutation profiles of samples expressing low or high MALAT1, then we compared the prevalences of differentially mutated genes between the two groups using Fisher’s exact test and enrichment analysis. Differences associated with p < 0.05 were considered significant. Samples were categorized as wild-type or mutant depending on the presence or absence of somatic mutations. Survival of patients in the wild-type or mutant groups was compared using the Kaplan-Meier method and log-rank test.
DNA methylation data analysis
After being loaded, methylation data were filtered, quality-controlled, and normalized using the ChAMP package in R. Beta values were the quantification values of methylation levels. The singular value decomposition method (SVD) calculated the correlation between biological factors (MALAT1 and other clinical features) and the variation of beta values. Differentially methylated positions (DMPs) were identified by comparing samples expressing high or low MALAT1. We considered that the following DMPs could upregulate target genes of MALAT1: hypermethylated DMPs in the coding sequence of the gene, or hypomethylated DMPs in the promoter or transcriptional start site (TSS). Conversely, we considered that the following DMPs could downregulate target genes of MALAT1: hypomethylated DMPs in the coding sequence of the gene, or hypermethylated DMPs in the promoter or TSS. We then calculated correlation coefficients between MALAT1 and target genes to identify genes regulated by MALAT1-associated methylation. Results associated with p < 0.05 were considered significant. Cox regression was performed on the beta values of each DMP. DMPs that emerged as significant (p < 0.05) were shown as forest plots. Enrichment of Molecular Signatures Database’s terms or pathways in DMPs was assessed using gene set enrichment analysis (GSEA) using the “champ.GSEA()” function.
Analysis of tumor-associated signatures and patient survival
We used the Immuno-Oncology-Biological-Research (IOBR) package in R integrating six commonly used algorithms (MCPcounter, TIMER, xCell, CIBERSORT, EPIC, and quanTiseq), to calculate scores for tumor-infiltrating lymphocytes (TILs) and tumor-associated signatures. Scores that reached statistical significance were determined for 92 samples whose MALAT1 expression was below the lower end of the IQR and 92 samples whose MALAT1 expression exceeded the upper end of the IQR. Kaplan-Meier analysis was used to compare survival of patients whose MALAT1 expression was above or below the median, based on data for the TCGA-LIHC cohort. Differences between the two groups were compared using the log-rank test.
Collection of clinical data and survival information
We analyzed samples from 179 HCC patients who underwent radical resection in the Affiliated Tumor Hospital of Guangxi Medical University between April 2004 and December 2012. None of the patients received TACE, radiotherapy, chemotherapy, or other anti-tumor treatments before surgery. Tumor tissues from all patients, as well as paired adjacent non-tumor tissues (PANTs) from 19 patients, were frozen in liquid nitrogen immediately after surgery and stored at −80°C until RNA extraction. Two pathologists participated in the diagnosis of histopathology of resected tumor samples. One of these two pathologists is responsible for routine pathological diagnosis in the clinical practice, while the other is responsible for routine quality control in the clinical study. This work was approved by the Institutional Ethics Committee of the Affiliated Tumor Hospital of Guangxi Medical University (Nanning, China, Approval Number: LW2022145), which waived the requirement for informed consent because patients, at the time of treatment, consented for their anonymized data to be analyzed and published for research purposes.
Clinicopathological data on all patients were collected, including age, sex, Child-Pugh classification, tumor size, tumor numbers, clinical TNM stage, the presence or absence of vascular invasion, capsular infiltration, and cirrhosis. Laboratory results were also collected, such as serum levels of alpha-fetoprotein (AFP) and hepatitis B surface antigen (HBsAg).
Follow-up information of patients was collected for survival analysis. Patients were followed up every 3 months during the first 2 years, and thereafter every 6 months, for 2–10 years. Tumor recurrence was detected based on radiology and serum AFP examination. Relationships of MALAT1 expression with PFS and overall survival (OS) were explored.
Detection of MALAT1 expression
Total RNA was extracted from HCC tumors and PANTs using TRIzol (Invitrogen, Carlsbad, CA, United States) and stored at −80°C. RNA (1 μg) was reverse-transcribed into complementary DNA (cDNA) using the PrimeScriptTM RT Reagent Kit (Takara Bio, Kusatsu, Japan) in reactions with a total volume of 20 μl. Real-time PCR (RT-PCR) was performed in 10-μl reactions on a LightCycler® 480 II Real-time PCR system (Roche, Basel, Switzerland) using GAPDH as an internal reference. Reactions were performed in a microassay plate (Roche) at 95°C for 10 min, followed by 40 cycles at 95°C for 10 s and 60°C for 30 s. Primer sequences were: MALAT1 forward, 5′-CAGGCGTTGTGCGTAGAGGA-3′; MALAT1 reverse, 5′-TGCCGACCTCACGGATTTT-3′; GAPDH forward, 5′-GTCAGCCGCATCTTCTTT-3′; and GAPDH reverse, 5′-CGCCCAATACGACCAAAT-3′. All samples were tested in triplicate. Expression levels of MALAT1 were normalized to those of GAPDH and were calculated using the 2−ΔΔCT method (20).
Statistical analysis
All statistical analyses were performed using SPSS 22.0 (IBM, Chicago, IL, United States) and R version 4.1.0. Differences in continuous variables were compared using Student’s t-test for normally distributed data or Mann-Whitney U-test for skewed data. Patients’ survival was analyzed by the Kaplan-Meier method, and differences were assessed for significance using the log-rank test. Univariate analysis was performed to identify clinicopathological features associated with survival, and results were assessed for significance using the Gehan-Breslow-Wilcoxon test.
Covariates that were associated with p < 0.05 in univariate analysis were included in multivariate Cox analysis, which was used to select features for constructing Cox proportional hazard models to predict survival. Models for predicting PFS and OS were assessed using the area under time-dependent receiver operating characteristic curves (AUC), as calculated with the timeROC package in R. Net benefits of models was assessed using decision curves, plotted with the ggDCA package in R. The ability of MALAT1 expression to diagnose clinicopathological features of HCC patients was assessed using AUCs. All hypothesis testing was two-sided, with p < 0.05 considered statistically significant.
Results
MALAT1-associated interacting proteins are associated with HCC and prognosis
The protein interaction network related to MALAT1 was obtained using the text mining algorithm in STRING (Figure 1A). The text mining identified 10 proteins interacting with MALAT1 as EZH2, SRSF1, SMARCA4, HNRNPC, SUZ12, ELAVL1, SFPQ, SP1, AGO2, HDAC9. The proteins in the network were most enriched in KEGG terms associated with cancer (Figure 1B). Among these terms, HCC had the highest z-score, indicating that the proteins in this network were most closely related to HCC (Figure 1C). Five protein-coding genes in the first shell of the network were significantly associated with worse survival when highly expressed: AGO2, HNRNPC, EZH2, SFPQ, and SRSF1 (Figure 2; log-rank p < 0.05).
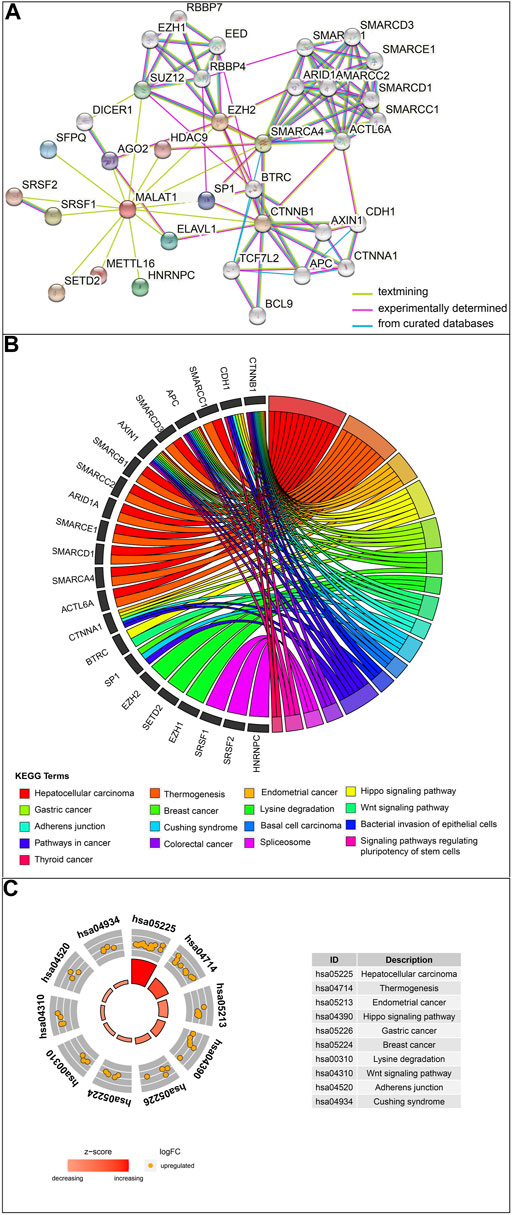
FIGURE 1. Analysis of MALAT1-interacting proteins. (A) The protein–protein interaction network of MALAT1 was analyzed using the STRING tool. (B) Chord plot displaying the relationship between MALAT1-interacting proteins and Kyoto Encyclopedia of Genes and Genomes (KEGG) terms. (C) The outer circle shows a scatter plot for each term of the log (fold change) of the assigned genes. Orange circles indicate upregulation. The z-score is a crude measure of significance of enrichment.
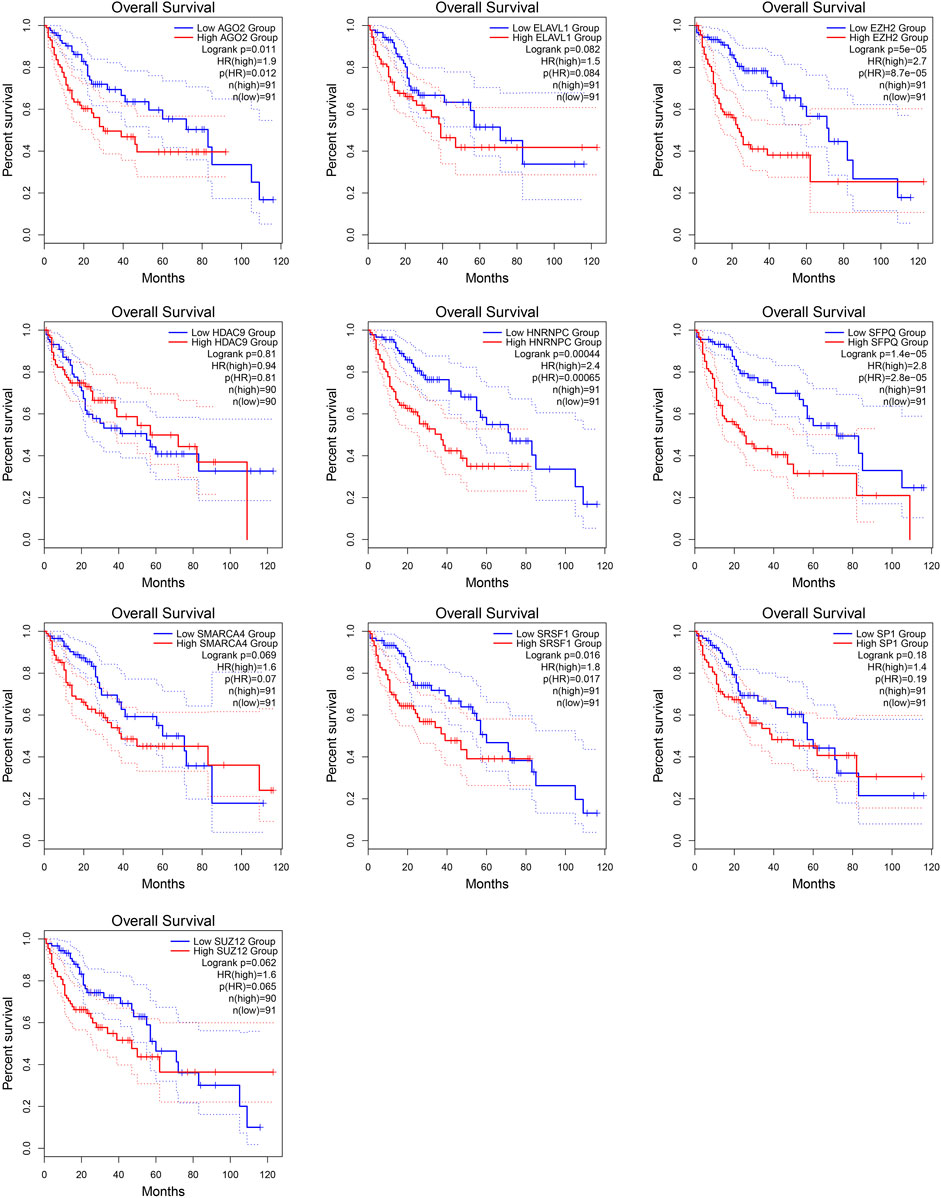
FIGURE 2. Kaplan-Meier survival curves for patients showing high or low expression of genes encoding MALAT1-interacting proteins.
Target genes of MALAT1 in the ceRNA network are associated with prognosis
From the ceRNA network for MALAT1, we obtained 10 target genes: ZBTB6, LCOR, PDE7A, SORL1, RPL37, RPL7L1, SREK1IP1, DIS3, PPP1R3C, CAMK2G. All these genes may competitively bind to the same miRNAs as MALAT1 (Figure 3). Higher expression of LCOR, PDE7A, RPL7L1, RPL37, and SREK1IP1 was associated with worse survival of HCC patients (log-rank p < 0.05; Figure 4).
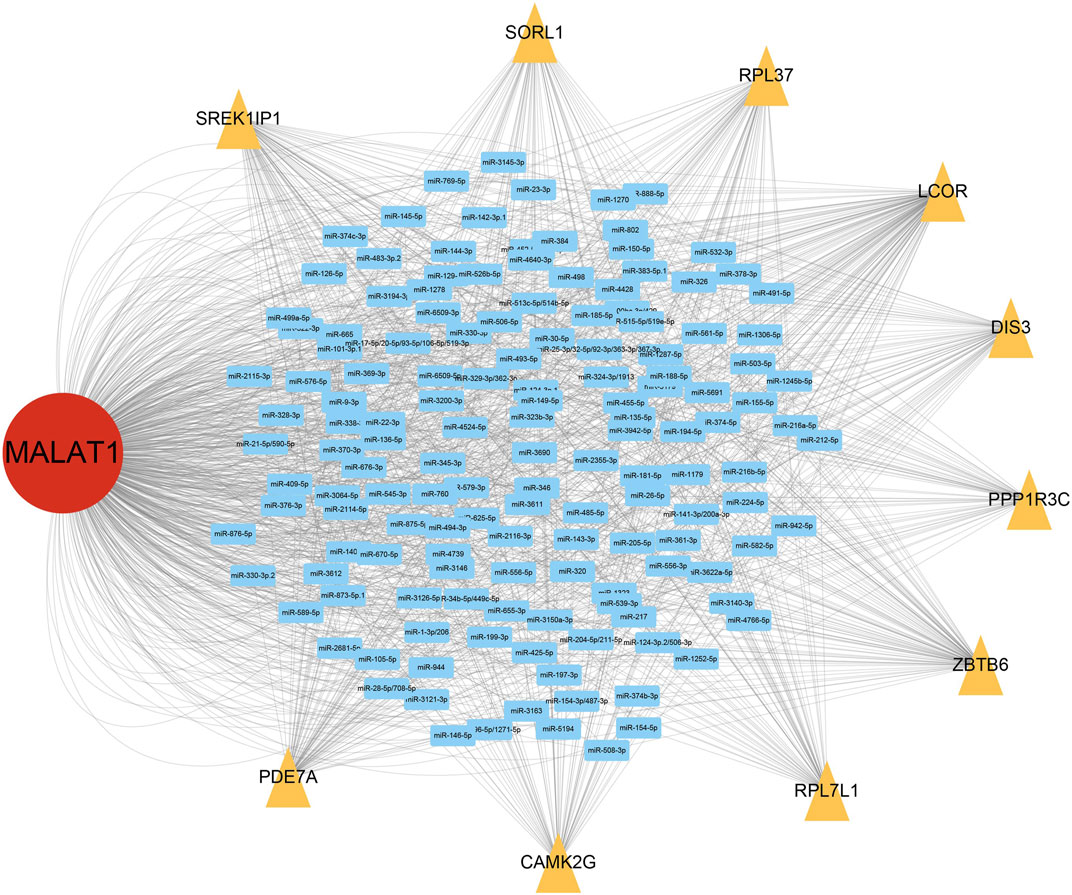
FIGURE 3. MALAT1-associated network of competing endogenous RNAs (ceRNAs). Blue rectangles represent microRNAs (miRNAs) that can bind to MALAT1; yellow triangles represent target genes that compete with MALAT1 for binding to miRNAs.
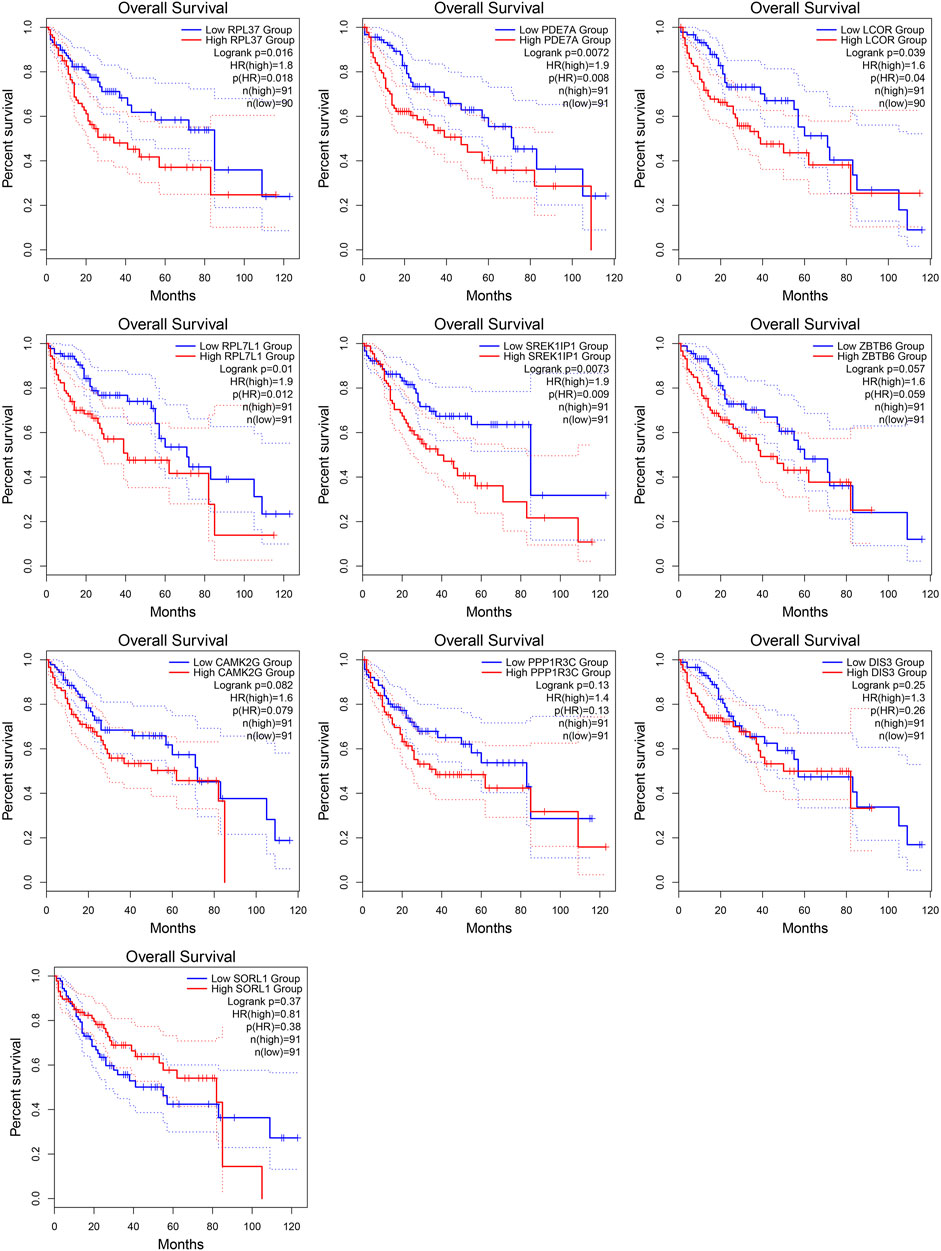
FIGURE 4. Kaplan-Meier survival curves for patients showing high or low expression of MALAT1 target genes.
MALAT1 expression is associated with genetic mutations in HCC
Supplementary Figure S1 showed the mutation profiles of the samples. Samples expressing high or low MALAT1 were compared using Fisher’s exact test to identify 10 differentially mutated genes (Figures 5A, B). Eight mutated genes were enriched in samples expressing high MALAT1 and 13 mutated genes were enriched in samples expressing low MALAT1 (Figure 5C). Patients with higher MALAT1 expression showed more mutations in IRX1 or TP53 and mutations in IRX1 and TP53 indicated worse survival than those with the corresponding wild-type alleles (log-rank p < 0.05; Figure 6). Patients with lower MALAT1 expression were associated with more LRP1B mutations and LRP1B mutations indicated worse survival (log-rank p < 0.05; Figure 6). Mutations in the 10 differentially mutated genes altered the protein sequences (Supplementary Figure S2).
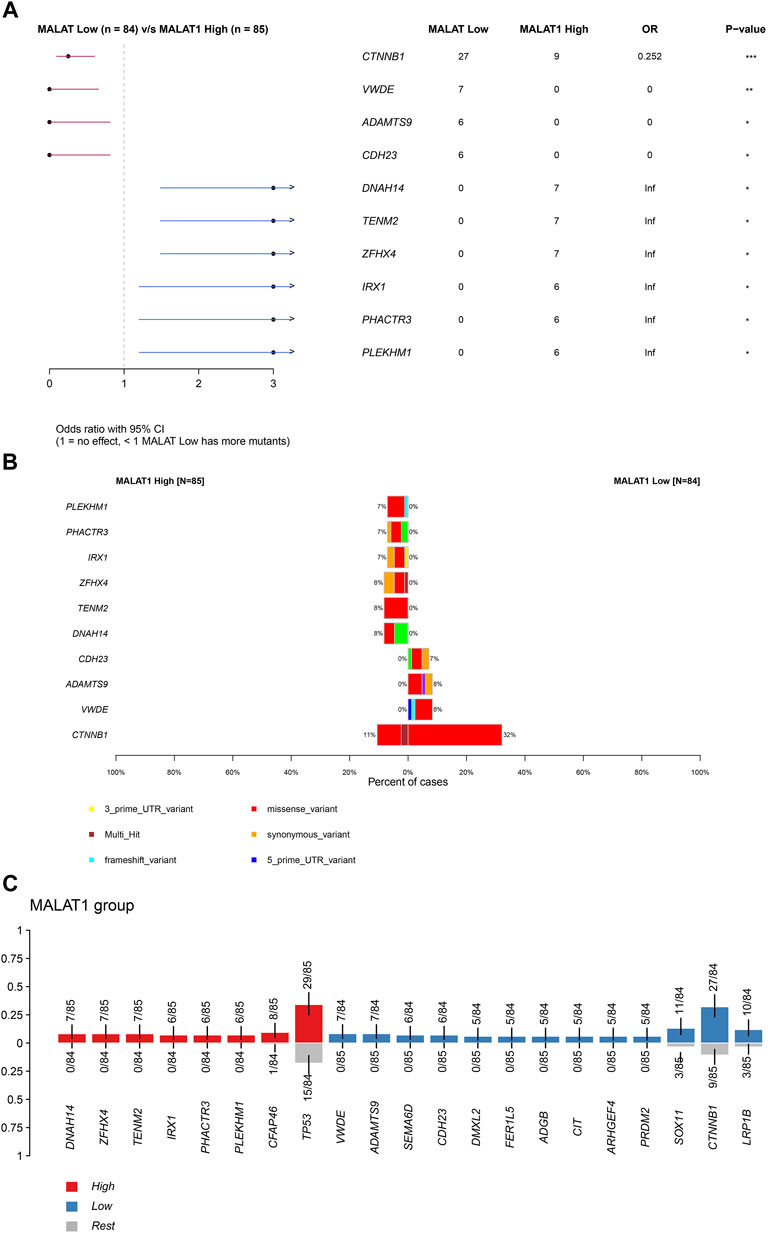
FIGURE 5. Differentially mutated genes. (A) Forest plot of Fisher testing to identify differentially mutated genes. (B) Proportion of each type of mutation and the overall mutation percentage of differentially mutated genes. The left half of the graph shows samples expressing high MALAT1 and the right half shows samples expressing low MALAT1. (C) Red represents mutated genes enriched in samples expressing high MALAT1. Blue represents mutated genes enriched in samples expressing low MALAT1.
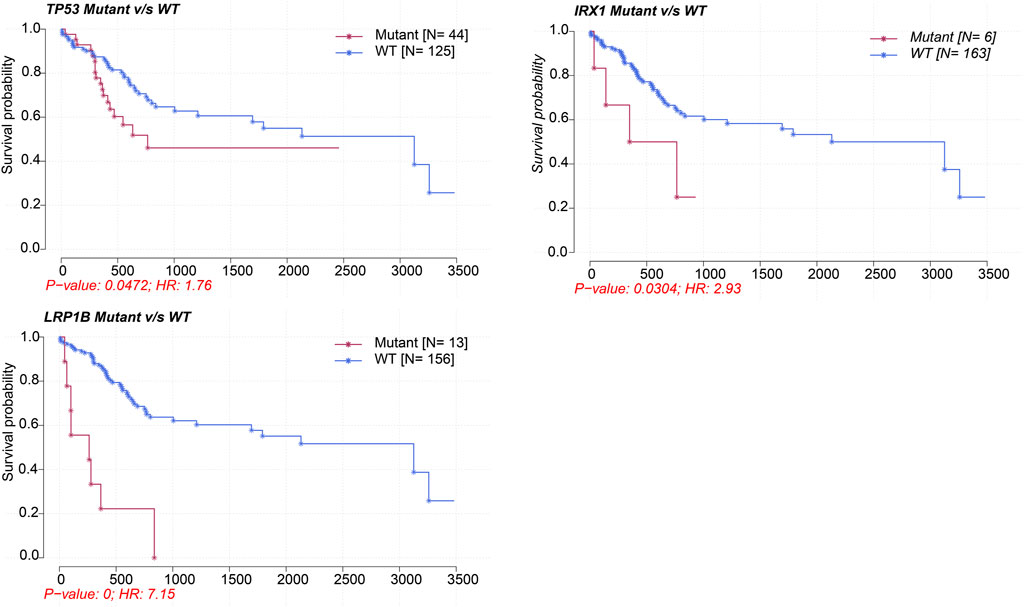
FIGURE 6. Kaplan-Meier survival curves comparing patients carrying mutant or wild-type versions of TP53, IRX1, and LRP1B.
MALAT1 expression is a correlate of DNA methylation in HCC
After normalizing the methylation signal value matrix, samples expressing high or low MALAT1 were analyzed by multidimensional scaling (Supplementary Figure S3A). The beta distributions for each sample showed that most probes were completely unmethylated (beta value ≤ 0.2), and a smaller number of probes were partially methylated (0.4 < beta value < 0.6) or completely methylated (beta value ≥ 0.8) (Supplementary Figure S3B). Sample clustering showed no outliers (Supplementary Figure S3C). The SVD plot showed that several principal components that best explained the methylation variation significantly correlated with MALAT1 (Figure 7). More principal components significantly correlated with MALAT1 than the clinical features of tumor stage and tumor grade, indicating that MALAT1 correlated with methylation variants more strongly than stage or grading did (Figure 7). In samples expressing high MALAT1, hypomethylation occurred in 88 positions and hypermethylation in 250 positions (Figure 8A).
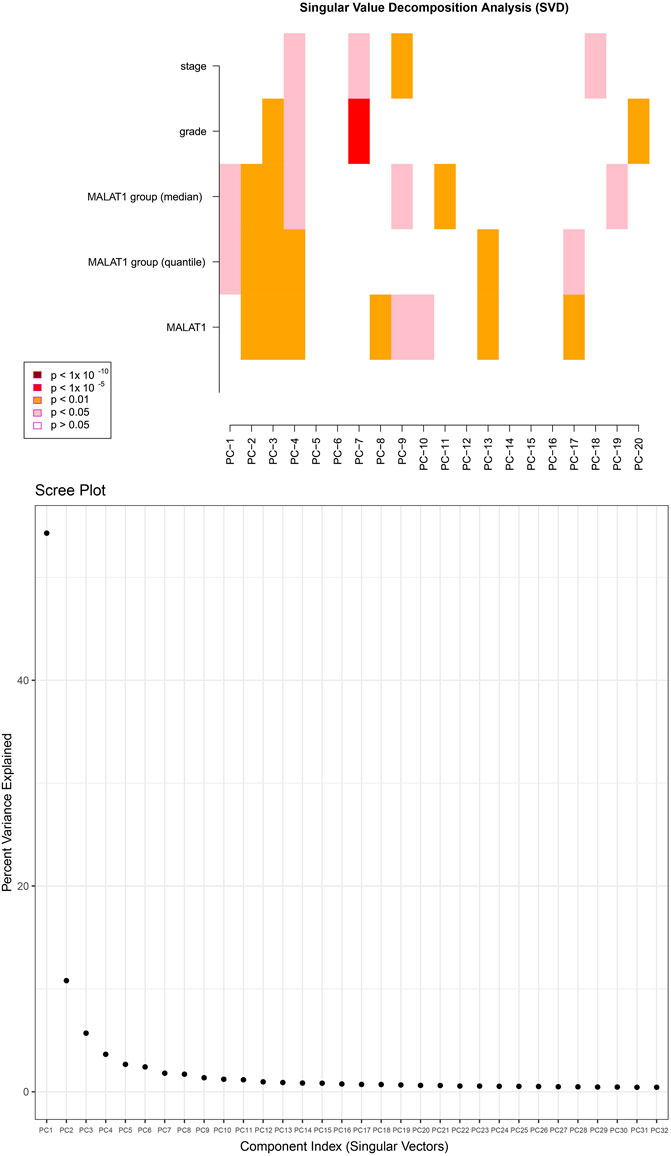
FIGURE 7. The upper plot is a heatmap of the top principal components correlating with MALAT1, tumor stage and tumor grade. “MALAT1” represents continuous variables, while “MALAT1 group (median)” and “MALAT1 group (quantile)” represent categorical variables grouped by median and quartiles. The color represents the degree of significance. The lower plot shows how much of the observed methylation variation was explained by the principal components.
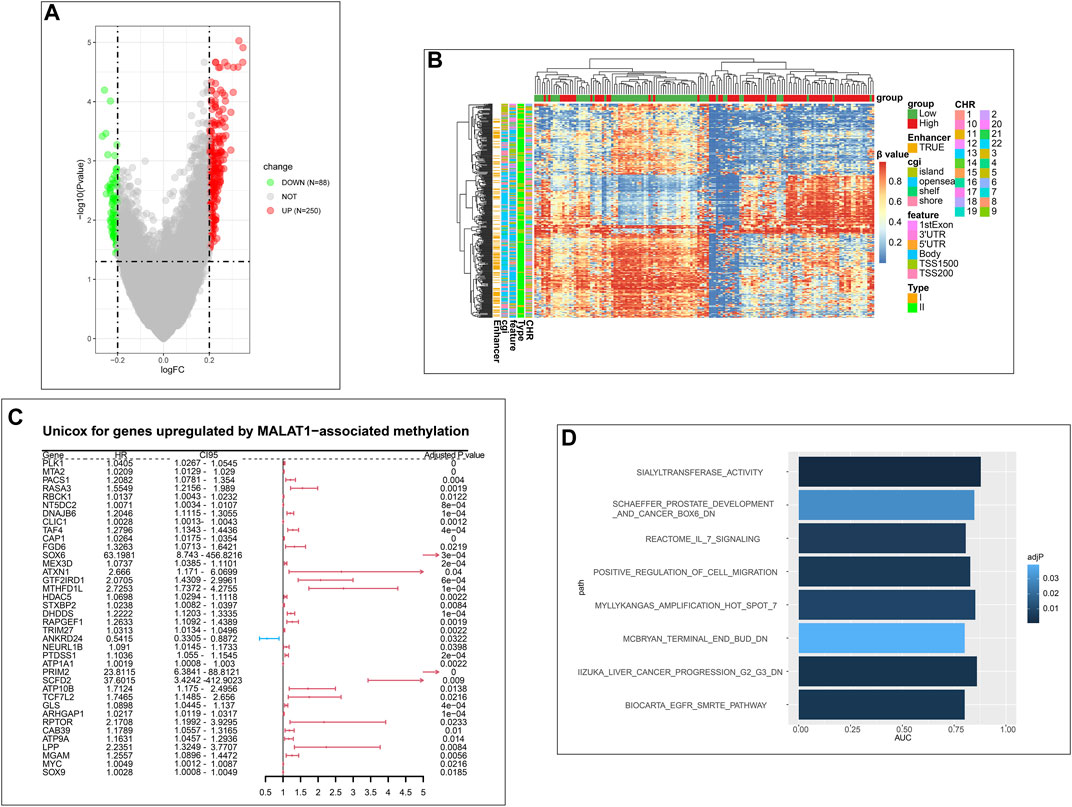
FIGURE 8. Analysis of differentially methylated positions (DMPs). (A) Volcano plot of DMPs. Green dots represent the positions that are hypomethylated. Red dots represent the positions that are hypermethylated. (B) Clustering heatmap of DMPs. Column annotations provide information about samples expressing high or low MALAT1. Row annotations provide information about DMPs. (C) Forest plot showing genes upregulated by MALAT1-associated methylation that were significant in Cox univariate analysis. (D): Bar plot of pathways with areas under curves (AUCs) > 0.75 in the Gene Set Enrichment Analysis (GSEA).
Beta values of DMPs were visualized using a clustering heatmap (Figure 8B). In the case of high MALAT1 expression, 157 DMPs were hypermethylated and located in coding regions of genes, while 28 DMPs were hypomethylated and located in promoters and TSS. These DMPs may positively correlated with gene expression. Conversely, 60 DMPs were hypomethylated and located in coding regions of genes, while 93 DMPs were hypermethylated and located in promoters and TSS. These DMPs therefore may negatively correlated with gene expression (Supplementary Figure S4).
We further analyzed the correlation of these genes with MALAT1 expression at the transcriptome level. We assumed that 87 genes were upregulated by MALAT1 through methylation, and confirmed that their expression positively correlated with that of MALAT1. Conversely, three genes were assumed down-regulated by MALAT1 through methylation, and their expression negatively were confirmed correlated with that of MALAT1 (Supplementary Figure S5).
Univariate Cox regression was performed for 87 up-regulated genes and 3 down-regulated genes, of which 38 were significantly upregulated; all genes emerged as risk factors for poor survival except ANKRD24, which was a protective factor (Figure 8C). Eight terms with AUCs > 0.75 in GSEA were associated with tumors. One of the terms “IIZUKA_LIVER_CANCER_PROGRESSION_G2_G3_DN” was directly related to liver cancer progression (Figure 8D). Therefore, the MALAT1-related methylation probes were associated with HCC progression and survival of patients.
MALAT1 expression is associated with tumor signatures and TILs
In tumor signatures and TIL analysis, we found significant enrichment in some tumor-related signatures scores in samples expressing high MALAT1. These signatures included genes involved in the cell cycle, DNA damage repair (DDR), mismatch repair, homologous recombination, molecular cancer m6A, exosome, and positive regulation of exosomal secretion (Figure 9). In contrast, ferroptosis scores were significantly lower in samples expressing high MALAT1.
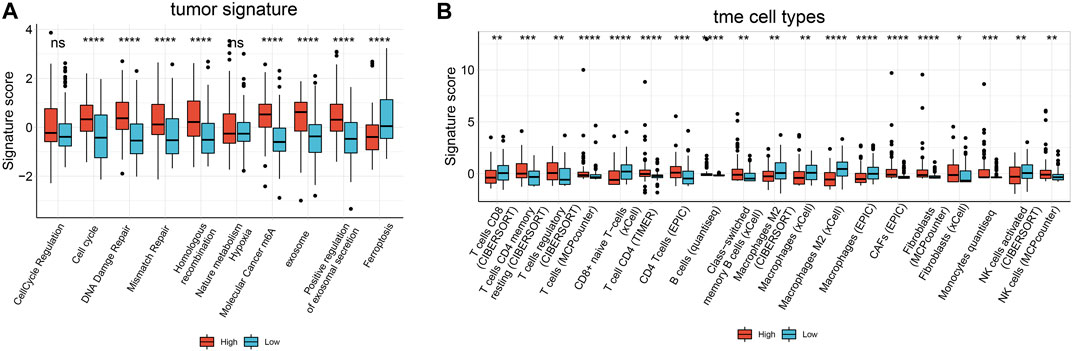
FIGURE 9. Boxplot of the results of Immuno-Oncology-Biological-Research (IOBR) analysis comparing samples expressing high or low MALAT1 in terms of (A) tumor-associated signatures and (B) cells within the tumor microenvironment. *p < 0.05, **p < 0.01, *** < 0.001, ****p < 0.0001.
We used six algorithms to estimate TILs in HCC. Several algorithms showed consistent results for the analysis of macrophages and fibroblasts. Macrophage scores were significantly higher in samples expressing low MALAT1, whereas fibroblasts scores were significantly higher in samples expressing high MALAT1 (p < 0.05; Figure 9). In analyses of other cell types, there were few significant results or different algorithms were inconsistent in predicting the trends (Figure 9).
MALAT1 is associated with HCC progression
Among HCC patients in the TCGA-LIHC cohort, there was no significant difference in OS between those with high or low MALAT1 expression (Figure 10A). PFS was significantly shorter in patients with high MALAT1 expression than in those with low expression (Figure 10B; p = 0.014). In a multivariate Cox regression model for MALAT1 expression and baseline clinical information (age, gender, tumor stage, and tumor grade), MALAT1 independently predicted shorter PFS (Figure 10C; p = 0.023).
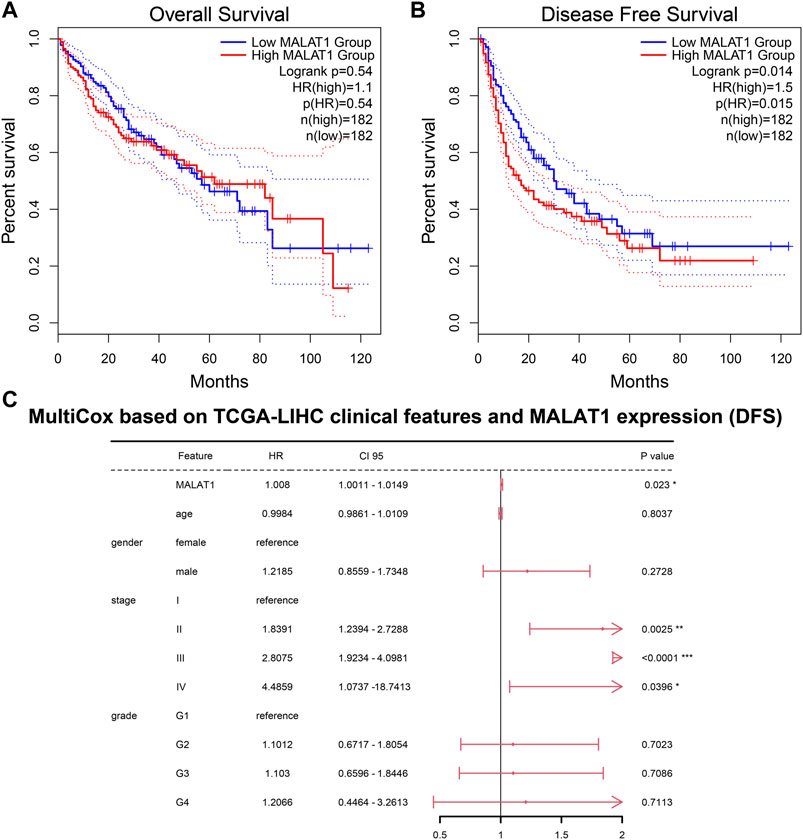
FIGURE 10. Prognosis analysis based on the TCGA LIHC cohort. (A) Overall survival and (B) Disease-free survival. Survival curves of patients with MALAT1 high expression or low expression were compared using the log-rank test. (C) A multivariate Cox regression model for disease-free survival containing MALAT1 and baseline clinical information. *p < 0.05; **p < 0.01; ***p < 0.001.
General characteristics of study subjects
The median age of the 179 HCC patients was 51 years (19–77 years). There were 160 males and 19 females. The median diameter of tumors was 5.5 cm (range, 2–19 cm). The proportion of patients positive for HBsAg was 86.6%, and the proportion with serum AFP was 65.4%. The baseline features of the cases are shown in Table 1.
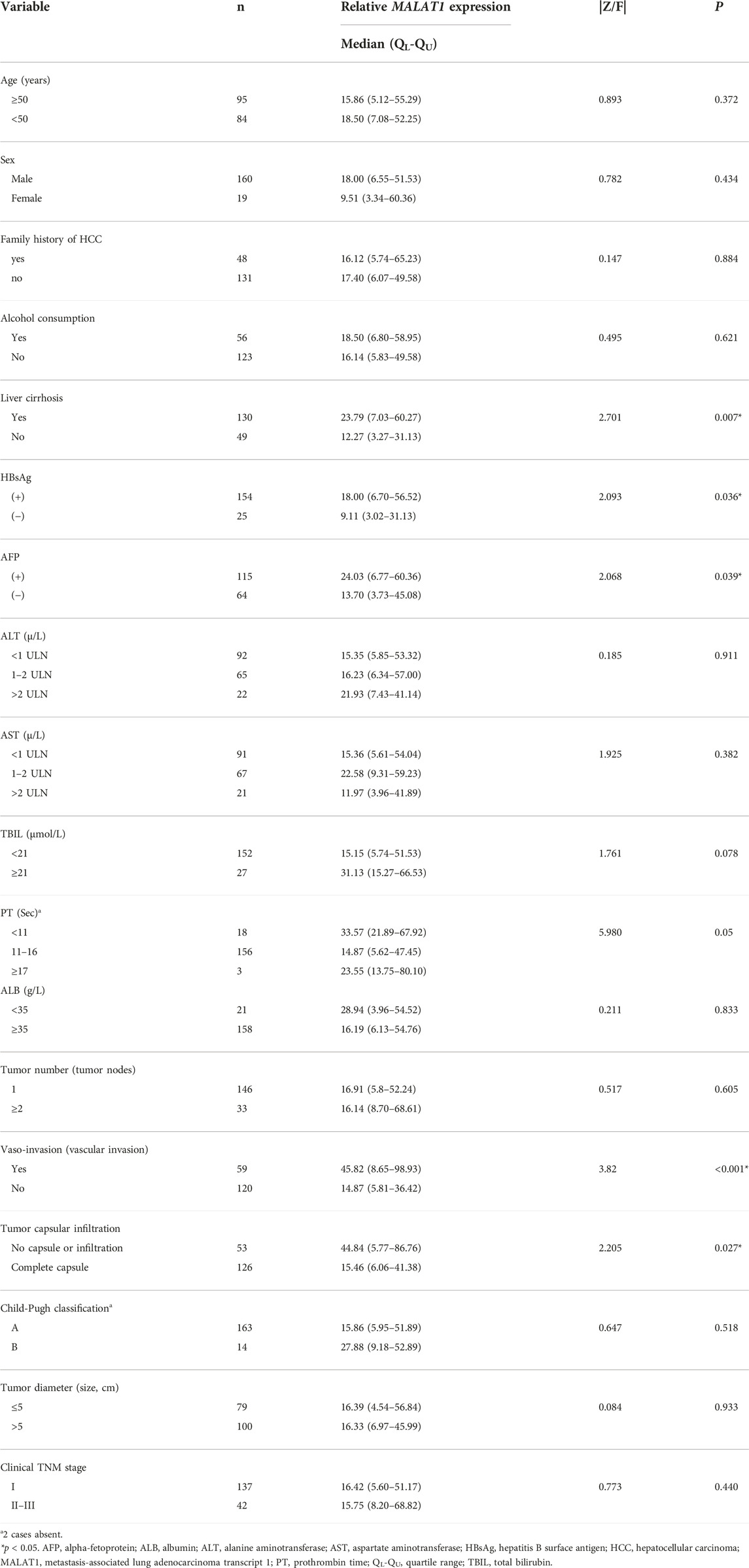
TABLE 1. Association between MALAT1 expression and clinicopathological variables in 179 HCC patients.
MALAT1 expression in HCC tumors and PANTs
Median relative expression of MALAT1 was significantly higher in cancer tissues than in PANTs (5.42 ± 3.84 vs. 0.048 ± 0.032, p < 0.001), confirming that MALAT1 was markedly upregulated in HCC (Figure 11A).
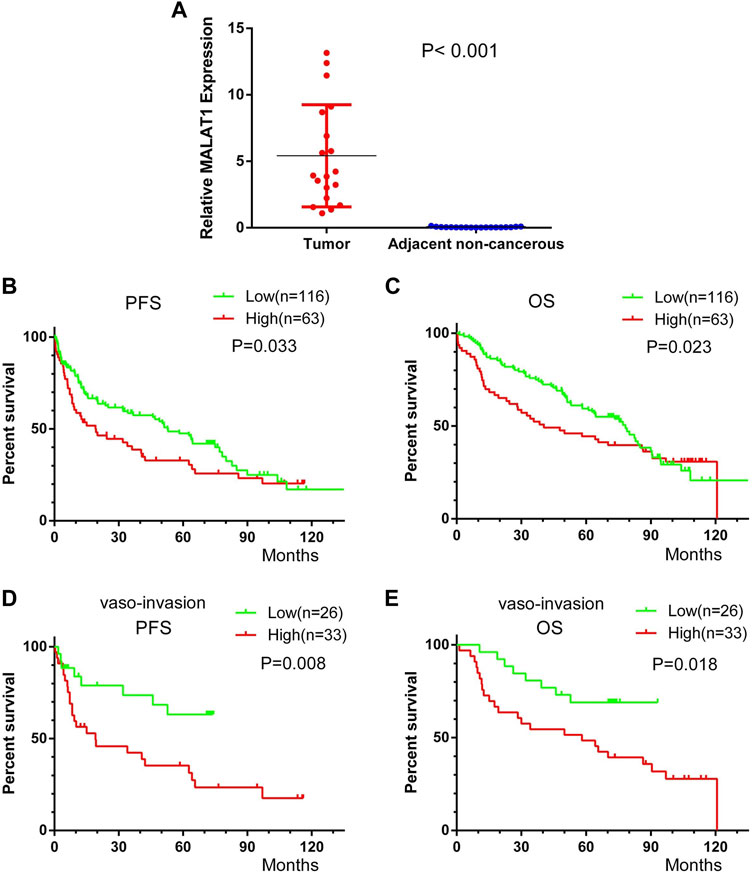
FIGURE 11. Kaplan-Meier survival curves of HCC patients after radical resection, stratified by low or high MALAT1 expression. (A) MALAT1 expression in 19 paired HCC and adjacent non-cancerous tissues from patients who underwent curative resection. (B,C) Progression-free survival (PFS) and overall survival (OS) of all patients. (D,E) PFS and OS of 59 patients with vascular invasion. Curves were compared using the log-rank test.
Correlation between MALAT1 expression and clinicopathological features of HCC patients
Subgroup analysis showed that patients with a history of liver cirrhosis, patients who were positive for HBsAg, patients who were positive for AFP, patients with vascular invasion and patients with tumor capsule infiltration had significantly higher MALAT1 expression than the corresponding patient subgroups without these characteristics (all p < 0.05). Nevertheless, there was no significant correlation between MALAT1 expression and age, sex, tumor diameter or number, Child-Pugh classification, clinical TNM stage, serum level of transaminase, total bilirubin, or albumin (all p ≥ 0.05; Table 1).
Correlation between MALAT1 expression and prognosis of HCC patients
Across all 179 patients, the median follow-up duration was 41 months (range, 23–59 months) for PFS and 70 months (range, 55–85 months) for OS. Patients were divided based on the mean value of MALAT1 relative expression level of 38.76 into a high expression group (n = 63) and low expression group (n = 116).
Patients with high MALAT1 expression were with 19.2 months mPFS and 40.5 months mOS and patients with low MALAT1 expression were with 52.8 months mPFS and 78.3 months mOS. Patients with high MALAT1 expression showed significantly shorter PFS than those with a low MALAT1 expression (p = 0.033, Figure 11B). Similarly, they showed significantly shorter OS (p = 0.023, Figure 11C).
In 59 patients with vascular invasion, the mPFS of patients with MALAT1 overexpression and low expression were 14 months and 49 months, and PFS was significantly shorter among those with high MALAT1 expression than among those with low expression (HR 2.63, 95% CI 1.294–5.346; p = 0.008, Figure 11D). Similarly, the mOS of patients with MALAT1 overexpression and low expression were 58 and 72 months, and OS was significantly shorter among those with high MALAT1 expression (HR 2.416, 95% CI 1.160–5.031; p = 0.018, Figure 11E).
MALAT1 overexpression was associated with shorter PFS and OS in subgroups who were positive for HBsAg or AFP (Table 2). Among 130 patients with liver cirrhosis, high MALAT1 expression was associated with significantly shorter OS than low expression (p = 0.018) and with a tendency toward shorter PFS (p = 0.0566; Table 2). In contrast, the expression level of MALAT1 was not significantly associated with PFS or OS among patients without vascular invasion, without cirrhosis, who were negative for HBsAg or who were negative for AFP (Table 2).
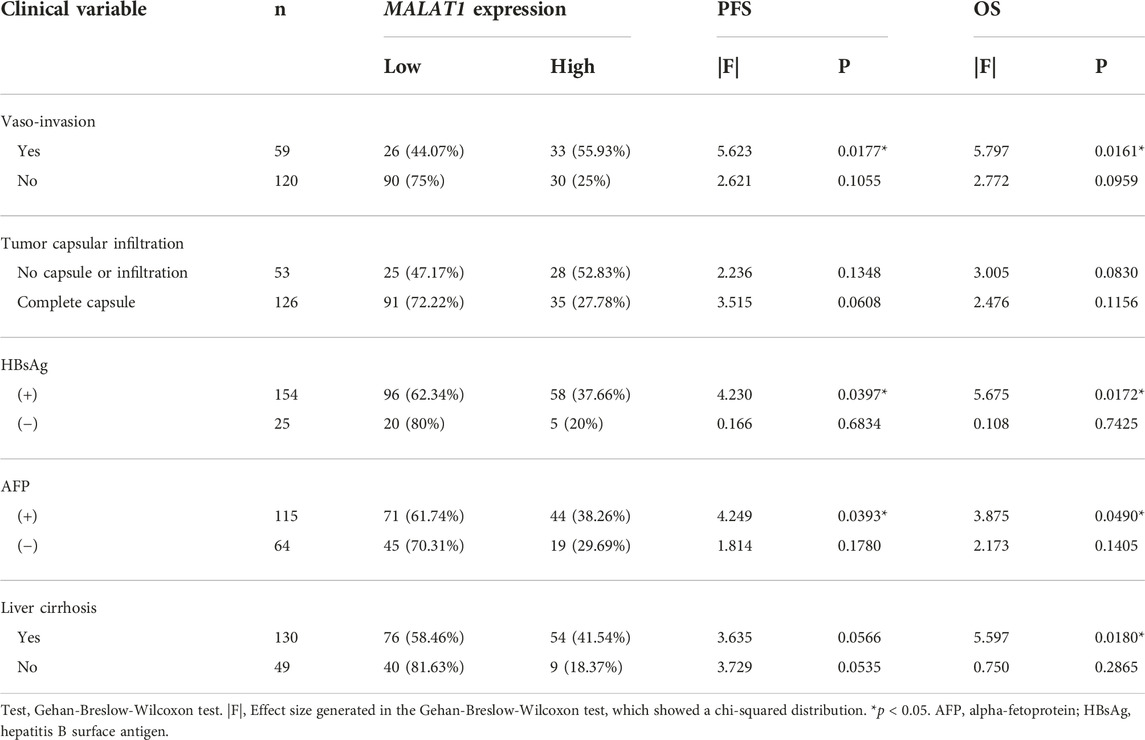
TABLE 2. Univariate analysis to identify factors associated with PFS or OS in different subgroups of HCC patients.
Prognostic factors in patients with HCC after hepatectomy
Univariate analysis showed that sex, HBsAg, tumor number, tumor diameter, TNM stage, and MALAT1 expression were significantly associated with OS and PFS (Table 3).
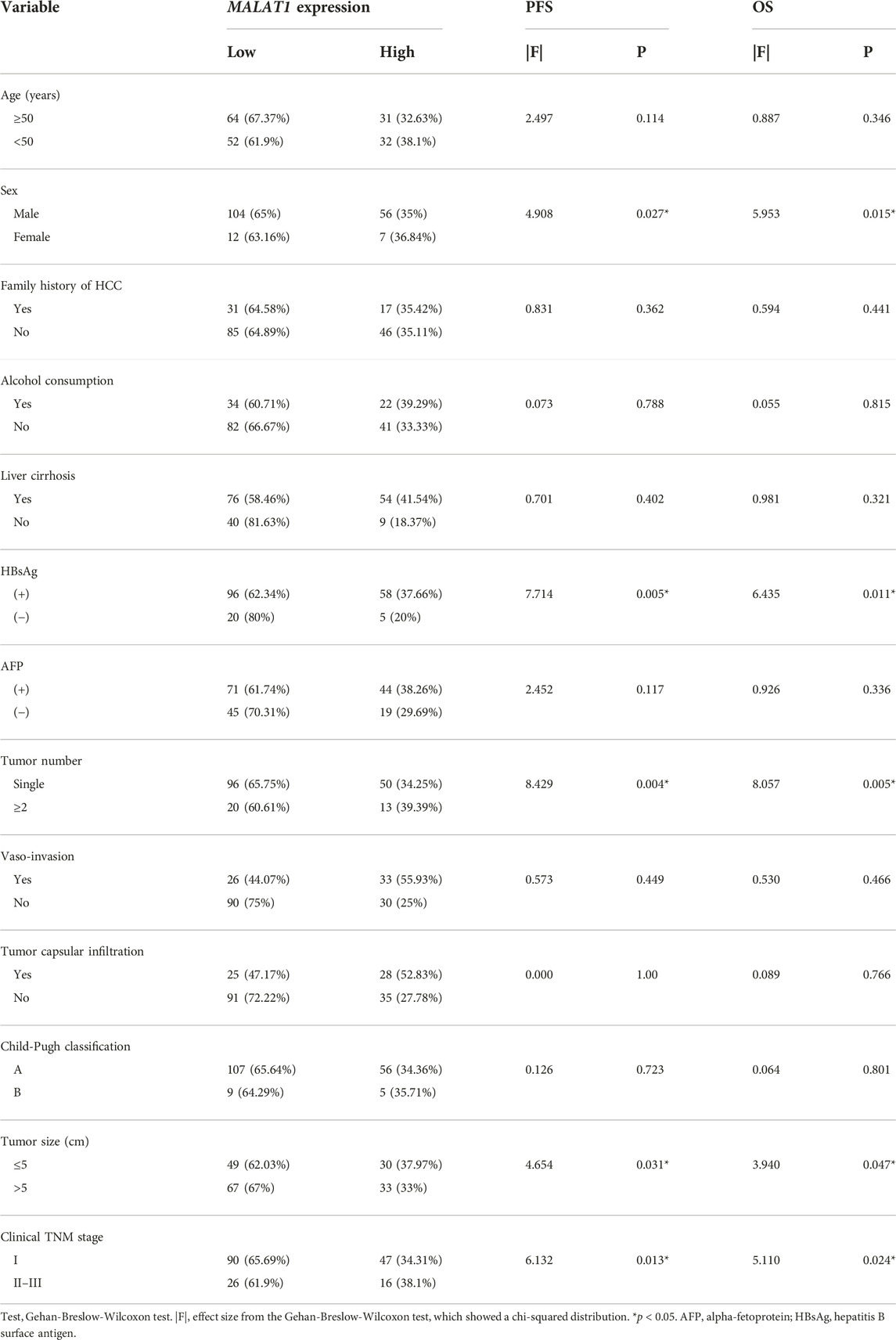
TABLE 3. Comparison of PFS and OS between HCC patients expressing low or high MALAT1 levels, stratified by clinicodemographic variables.
Variables with p < 0.05 in the univariate analysis were included in the multivariate Cox analysis. Furthermore, the results showed that the independent risk factors for OS included sex, HBsAg, and clinical TNM stage (p < 0.05; Figure 12). At the same time, HBsAg and clinical TNM stage were independent risk factors for PFS (p < 0.05; Figure 12). The p-values of tumor size from OS and PFS multivariate Cox analysis was less than 0.1, and the p-value of MALAT1 expression from PFS multivariate Cox analysis was less than 0.1 (Figure 12).
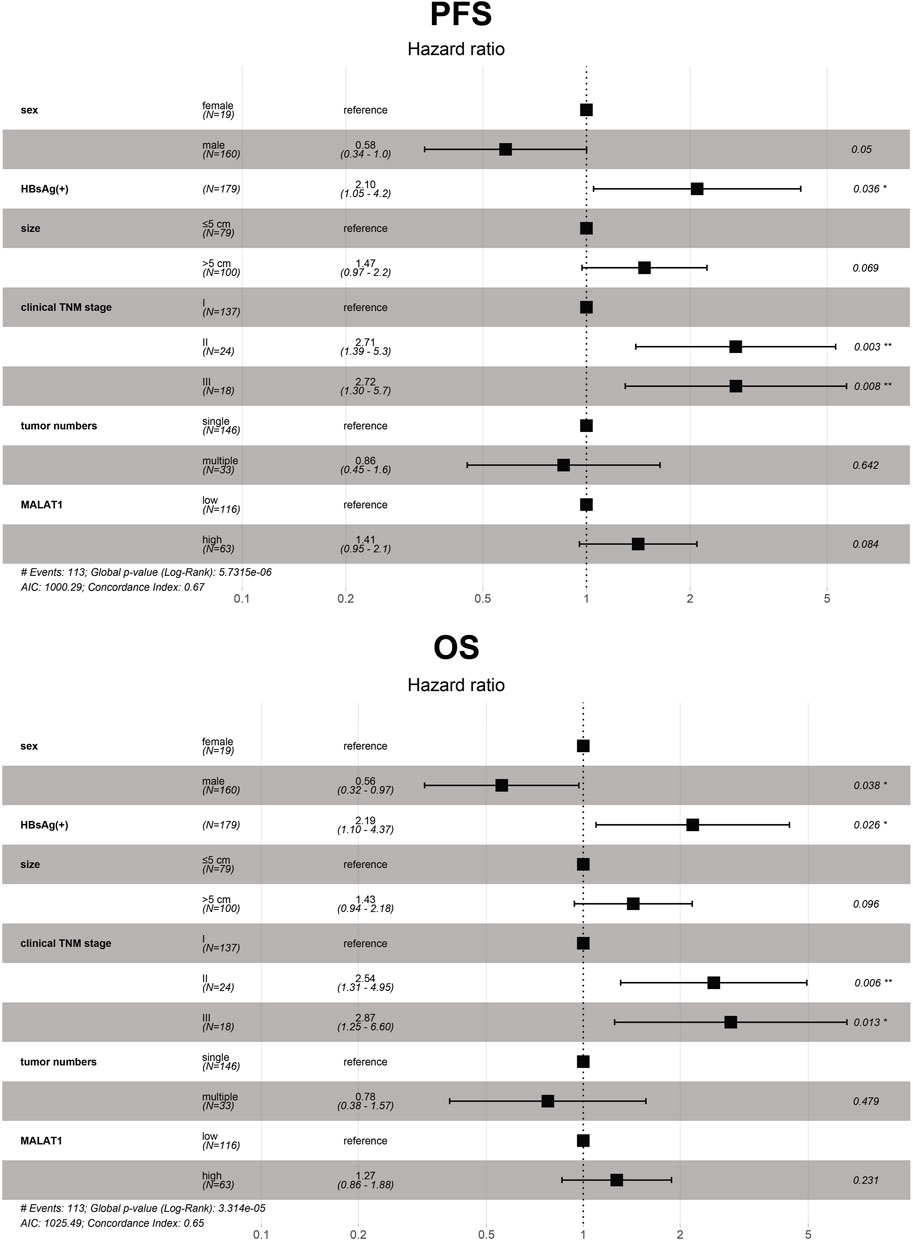
FIGURE 12. Multivariate analysis of 179 HCC patients to identify independent risk factors of progression-free survival and overall survival. The dots and bars represent the HR and 95% CI, respectively. CI, confidence interval; HR, hazard ratio; MALAT1, metastasis-associated lung adenocarcinoma transcript 1; OS, overall survival; PFS, progression-free survival.
A prognostic model based on MALAT1 in combination with other prognostic indicators has moderate predictive power
The multivariate Cox analysis showed that there was strong collinearity between the variable “tumor number” and “clinical TNM stage” and the prognostic value of “sex” was not clear. Therefore, we constructed prediction models based on four promising variables: “MALAT1,” “size,” “clinical TNM stage,” and “HBsAg” (Figures 13A, B). The calibration curves showed that the progression prediction model fit the data well at 1, 2 and 3 years (Figure 13C), while the survival prediction model fit the data moderately well at 1, 3, and 5 years (Figure 13D). Using the progression prediction model, we obtained AUCs of 0.683 for 1-year progression rate, 0.692 for 2-year progression rate, and 0.661 for 3-year progression rate (Figure 14A). Using the survival prediction model, we obtained AUCs of 0.731 for 1-year survival rate, 0.703 for 3-year survival rate, and 0.699 for 5-year survival rate (Figure 14B). Time-dependent AUCs showed that the prediction models outperformed the predictions of individual indicators. Predictive models constructed with other indicators excluding MALAT1 were slightly inferior to MALAT1-based predictive models, for both PFS and OS. The performance of MALAT1 alone was not inferior to that of clinical stage and HBsAg in the prediction of progression, or from that of tumor size and HBsAg in the prediction of survival when data were censored at > 40 months (Figures 14C, D). Decision curves were plotted by depicting the net benefit ratio along the vertical axis and the risk threshold along the horizontal axis (Figures 14E, F). The results showed that the smaller the risk threshold value, the greater the net benefit. Over most threshold intervals, the progression and survival prediction models led to greater net benefit than models based on other independent indicators.
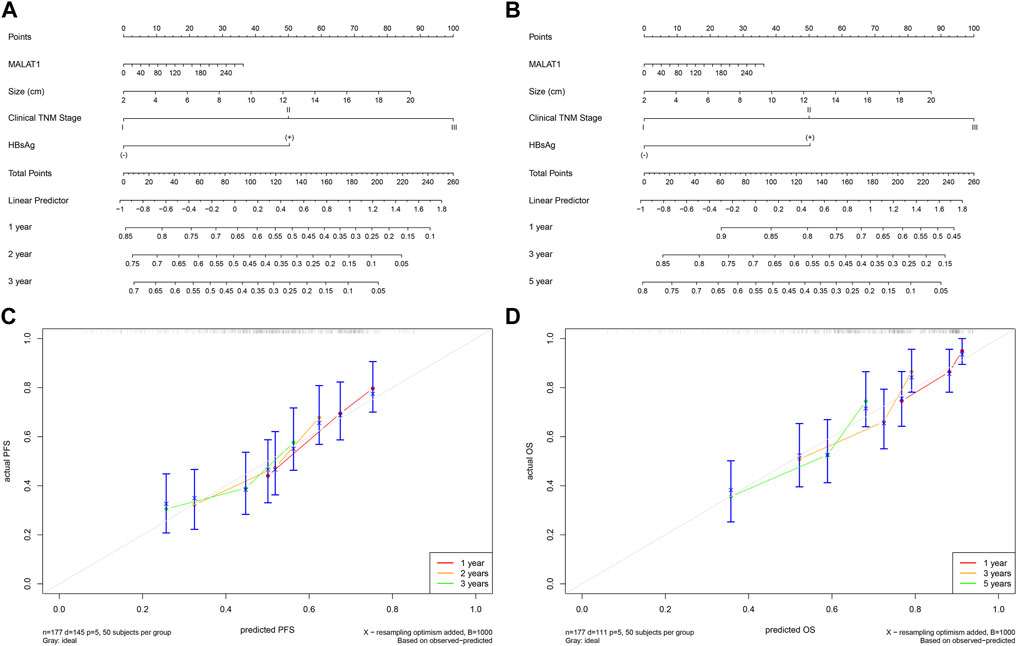
FIGURE 13. Progression and survival prediction models. (A): Nomogram of the progression prediction model. (B): Nomogram of the survival prediction model. (C): Calibration curve for the progression prediction model. (D): Calibration curve for the survival prediction model.
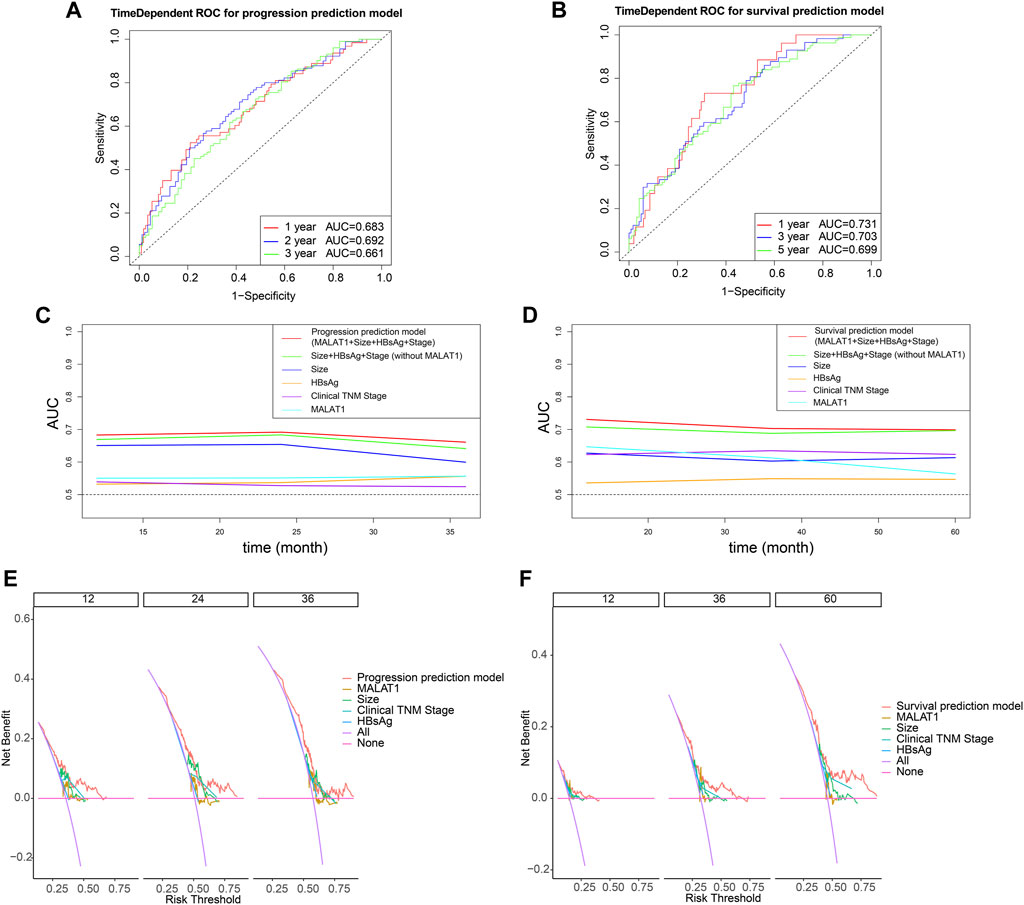
FIGURE 14. Evaluation of model performance using time-dependent receiver operating characteristic curves. (A) Predictive power of the progression prediction model. (B) Predictive power of the survival prediction model. (C) Comparisons of areas under curves (AUCs) for progression prediction model, other prognostic parameters and MALAT1 alone. (D) Comparisons of areas under curves (AUCs) for survival prediction model, other prognostic parameters and MALAT1 alone. (E): Decision curves for the progression prediction model, other prognostic parameters and MALAT1 alone. (F): Decision curves for the survival prediction model, other prognostic parameters and MALAT1 alone.
Diagnostic value of MALAT1 for clinicopathological features
Using an appropriate cut-off value, the AUC of MALAT1 expression level used to distinguish patients with or without liver cirrhosis was 0.631 (cut-off value 3.86, p = 0.006; Table 4; Figure 15A), 0.676 for patients with or without vascular invasion (cut-off value 54.52, p < 0.001; Figure 15B), 0.605 for patients with or without tumor capsule infiltration (cut-off value 54.52, p = 0.039; Figure 15C), and 0.593 for patients positive or negative for AFP (cut-off value 4.23, p = 0.037; Figure 15D).
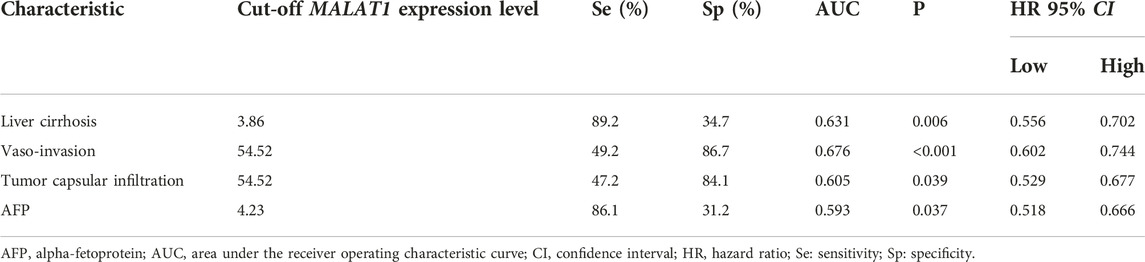
TABLE 4. Assessment of the ability of MALAT1 to diagnose clinicopathological characteristics in HCC patients undergoing radical resection.
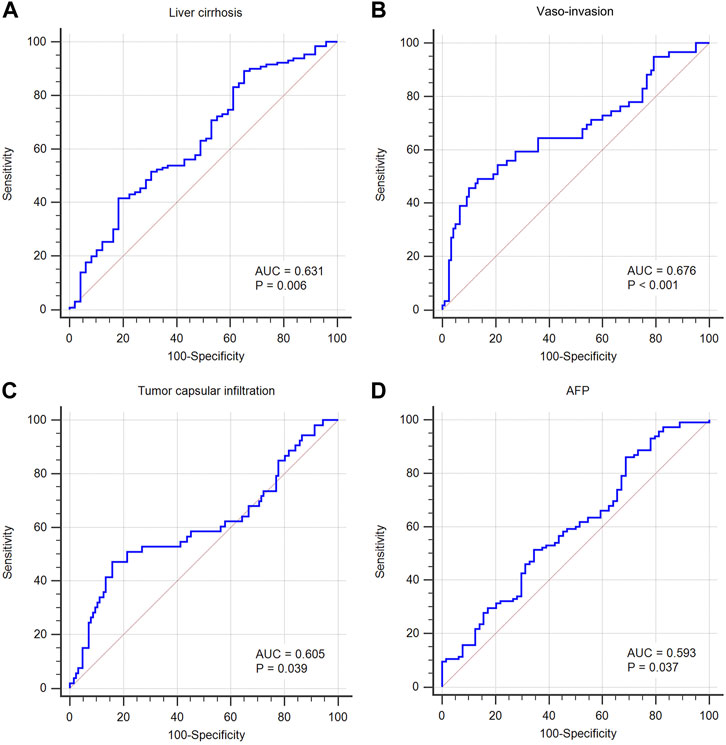
FIGURE 15. Predictive value of MALAT1 for diagnosing clinicopathological characteristics, based on the area under the time-dependent receiver operating characteristic curve (AUC). (A) AUC was 0.631 for cirrhosis (95% CI: 55.6%–70.2%, p = 0.006). (B) AUC was 0.676 for vascular invasion (95% CI: 60.2%–74.4%, p < 0.001). (C) AUC was 0.605 for tumor capsular infiltration (95% CI: 52.9%–67.7%, p = 0.039). (D) AUC was 0.593 for AFP positive (95% CI: 51.8%–66.6%, p = 0.037).
Discussion
MALAT1 was initially reported as a biomarker of metastasis in the early lung adenocarcinoma (21). Increasingly investigations showed that MALAT1 was not only a reliable metastasis-related biomarker but also a vital regulator involved in occurrence, invasiveness, drug resistance, and metastasis of various cancers such as HCC and lung cancer. Studies have revealed that MALAT1 overexpression is significantly related to patient outcomes. For example, upregulated MALAT1 is associated with poor disease-free survival and OS in patients with middle thoracic esophageal squamous cell carcinoma (22) and osteosarcoma (23). Recently, several studies have been conducted to investigate the prognostic value of MALAT1 in HCC, but the results have been inconsistent. Lai et al. (24) found that MALAT1 was overexpressed in 9 liver cancer cell lines and 112 HCC tissues, and survival analysis showed that patients with high level of MALAT1 had a significantly increased risk of recurrence after liver transplantation. Multivariate analysis confirmed that MALAT1 was an independent prognostic factor for predicting the recurrence of HCC (HR 3.280, p = 0.003). However, another study obtained quite different results: Sonohara et al. found increased expression of MALAT1 in HCC and that MALAT1 expression in HCC was associated with better survival (25). Therefore, the prognostic value of MALAT1 in HCC needs to be investigated by a broader range of research methods.
Since MALAT1 is a lncRNA, it is not directly involved in protein coding but instead it regulates the expression of coding genes through mechanisms such as ceRNA (14) and perhaps also methylation and mutation (15, 26). In this study, we investigated the relationships of MALAT1 with protein-coding genes, ceRNAs, methylation profiles, and mutation profiles, thus identifying various ways in which MALAT1 affects the survival of HCC patients. First, we used text mining to find MALAT1-interacting proteins, half of whose coding genes were significantly associated with the poor survival of HCC. Enrichment analysis suggested a high correlation between MALAT1-interacting proteins and HCC. These analyses suggest that MALAT1-interacting proteins may mediate the observed association of MALAT1 with prognosis of HCC patients. The high correlation between MALAT1-interacting proteins and HCC also suggests that MALAT1 has a vital role in the pathogenesis underlying HCC. Indeed, five prognosis-related MALAT1-interacting proteins, AGO2, HNRNPC, EZH2, SFPQ, and SRSF1, have previously been linked to hepatotumorigenesis. AGO promotes cell proliferation and angiogenesis in HCC (27, 28); silencing HNRNPC inhibits proliferation, migration, and invasion of HCC cells (29); EZH2 enhances protein kinase B activation to promote HCC progression (30); SFPQ plays an important role in the enhancement of fatty acid biosynthesis by NONO to promote HCC progression (31); and SRSF1 promotes HCC development, which can be regulated by MALAT1 (32, 33).
The most important link between MALAT1 and HCC pathogenesis appears to be mediated by MALAT1 acting as a ceRNA to affect the target pathways FOXA1/CD24/Src and PI3K/Akt/mTOR, as well as the target genes IAP, ZEB1, and FOXM1. Through these targets, MALAT1 promotes HCC proliferation and angiogenesis, inhibiting apoptosis, and promoting the progression of HCC (34–38). To identify more potential target genes, we constructed a network of MALAT1-related ceRNAs using CLIP-seq data. Interestingly, the predicted target gene CAMK2G has been linked to the proto-oncogene tyrosine-protein kinase Src family, which was previously confirmed by others an MALAT1 target (34). CAMK2G is a subunit of calcium/calmodulin-dependent protein kinase II (CaMKII). The reactome database showed that CaMKII and Src participate together in several serine threonine kinase (RAF)-related pathways or complexes (39), and that both CAMK2G and Src are targets of tyrosine kinase inhibitors (40). These predicted target genes may be linked to confirmed target genes or new, yet-to-explored mechanisms.
Half of the target genes regulated by MALAT1 as a ceRNA were significantly associated with poor survival, suggesting that MALAT1 may influence both HCC pathogenesis and patient prognosis. Among these, LCOR and PDE7A have been shown to play roles in other types of cancer. LCOR activates transcription and can promote the development of breast cancer (41) and the low differentiation of cervical intraepithelial neoplasia (42). Inhibition of PDE7A expression inhibits cancer cell proliferation, migration and invasion in endometrial cancer, while overexpression of PDE7A has the opposite effects (43). The effects of MALAT1 regulation of its target genes and the effect of those genes on HCC should be explored.
MALAT1 expression was also associated with mutational events in our study. Lv et al. found that MALAT1 expression could be used as a marker of EGFR mutation status (44). Badalamenti et al. discovered that MALAT1 expression was associated with c-KIT mutation status (26). Ak et al. revealed that the C228T mutation in the TERT gene was associated MALAT1 expression and with worse prognosis in glioblastoma patients (45). The present study identified a correlation between MALAT1 and more than 20 mutational events in HCC by somatic mutation data analysis. Mutational events associated with high or low MALAT1 expression may explain the poor prognosis in HCC. In addition, we found that mutations in IRX1 and TP53 were more likely to occur when MALAT1 was highly expressed and were associated with worse survival. TP53 mutation, the most well-known cause of cancer, has been associated with adverse outcomes in HCC patients (46). Mutation in LRP1B, which was associated with low MALAT1 expression in HCC, also emerged as a prognostic indicator of worse survival. Therefore, MALAT1-associated mutations may be one of the reasons why dysregulation of MALAT1 expression is accompanied by poor prognosis.
In addition to genetic mutation, epigenetic modification of methylation in the promoter region has been suggested as an important alternative mechanism of tumorigenesis. Methylation of promoter regions of several genes has been associated with the development of HCC (47), and methylation features detected in tissues and serum have been used as biomarkers to predict the prognosis of patients with HCC (48). In our analysis of DNA methylation profiling data, MALAT1 expression significantly correlated with methylation variations, suggesting that MALAT1 as lncRNA may also have been associated with the up-or down-regulation of methylation signals at many methylation positions. Subsequently, we found that MALAT1-associated methylation was associated with poor survival of HCC patients and was enriched for terms associated with liver cancer progression. These findings suggest that MALAT1 may be involved in the progression and prognosis of HCC through its association with DNA methylation. Further work is needed to elucidate the molecular pathways linking MALAT1 expression and DNA methylation.
Based on RNA sequencing data, we transformed the gene expression profile into multiple tumor-associated signatures. MALAT1 is co-expressed with multiple signatures that can promote HCC progression. We were able to observe that high MALAT1 expression was accompanied by high expression of cell cycle-related genes, which is consistent with the finding of several studies that MALAT1 overexpression promotes the cell cycle and inhibits apoptosis (49–51). Similarly, high MALAT1 expression is accompanied by high expression of DNA repair-related genes, and studies have successfully induced DNA damage by targeting MALAT1 (52, 53). We found MALAT1 was positively associated with exosome characteristics, and others found that exosome-mediated intercellular communication allowed the transfer of MALAT1 as a lncRNA to play a regulatory role (54, 55). In contrast, MALAT1 was negatively associated with ferroptosis signature, and one study reported that silencing MALAT1 induced ferroptosis (56), reflecting the possibility that MALAT1 may also contribute to the development of HCC by inhibiting ferroptosis. TIL prediction analysis showed that high MALAT1 expression was associated with several immunosuppressive signatures, such as reduced macrophage infiltration and increased fibroblast infiltration. Wang et al. found that MALAT1 could induce fibroblast activation leading to gastric cancer progression (57). Hou et al. also found that MALAT1 was associated with macrophage differentiation in HCC. They found that MALAT1 negatively correlated with miR-140 and that inhibition of miR-140 polarized macrophages toward the M2 subtype and away from the M1 subtype (58). Further work is needed to clarify how MALAT1 functions in the tumor microenvironment.
We have discussed the multiple potential ways in which MALAT1 may be involved in the progression of HCC and the poor outcomes of HCC patients. To directly confirm the relationship between MALAT1 and the prognosis of HCC patients, we collected tissue samples from patients and conducted RT-PCR assays. The results showed that MALAT1 was significantly upregulated in HCC tissues relative to PANTs. Moreover, MALAT1 overexpression was closely related to the shorter PFS and OS in HCC patients after hepatectomy, especially in patients who were positive with HBsAg, who had a tumor diameter ≥ 5.0 cm, or who had tumor number ≥ 2. Thus, MALAT1 is associated with poor outcomes in HCC. However, different from previous results (24), the present study found that MALAT1 overexpression was not an independent risk factor for the prognosis of HCC patients after Cox multivariate analysis. The reason for this disrepancy may be that the patients in that previous study underwent liver transplantation, whereas our patients underwent radical resection, such that variation in regenerative capacity of residual liver tissue and liver function may have confounded the effects of MALAT1 overexpression on survival.
Molecular mechanisms of signaling pathways have shown that the upregulation of MALAT1 expression significantly promotes the malignant phenotype of HCC cells. By promoting β-catenin expression, MALAT1 activates the canonical Wnt signaling pathway and contributes to the formation of HCC tumor sphere, as well as the increase in CD133+ and CD90+ HCC cell populations (59). MALAT1 is involved in HCC cellular glucose metabolism: it enhances TCF7L2 translation and activates the mTORC1-4EBP1 axis (60). Other researchers found that the interaction of MALAT1 and miR-124 promotes HBx-induced stem cell characteristics in HepG2 cells by regulating PI3K/Akt signaling (61). By cooperating with enhancer of zeste homolog 2 (EZH2), MALAT1 promotes Snail family transcriptional repressor 1 (SNAI1) expression by sponging miR-22 and suppressing E-cadherin expression, ultimately promoting the epithelial-mesenchymal transition in HCC (62). The miR-3064-5p plays an anti-angiogenic role by inhibiting the FOXA1/CD24/Src pathway, and MALAT1 sponges this miRNA to weaken its suppressive effect (34). Overexpression of MALAT1 inhibits Mir-140, leading to overexpression of vascular endothelial growth factor A (VEGF-A) and promoting angiogenesis in human umbilical vein endothelial cells (HUVECs) (58).
Through the analysis of the relationship between MALAT1 and other HCC-associated clinical features, we also revealed that overexpression of MALAT1 was closely associated with clinical features such as liver cirrhosis, vascular invasion, tumor capsular infiltration, AFP positivity, and HBsAg positivity. Further analysis of the AUC curve confirmed that MALAT1 expression was helpful in the differentiation of patients with cirrhosis, vascular invasion, capsule infiltration, and AFP positivity.
In China, chronic infection with hepatitis B virus (HBV) is one of the key risk factors for HCC, and 80% of HCC cases are complicated with liver cirrhosis caused by hepatitis (63). Yuan et al. found that MALAT1 expression level was progressively unregulated as HCC advanced from normal liver to dysplastic liver to cirrhotic liver and finally to HCC (64). These results demonstrate an association between MALAT1 and HCC occurrence. In addition, MALAT1 overexpression induced proliferation and metastasis of cancer cells associated with vascular and capsular invasion in HCC (34, 58–62). The overexpression of MALAT1 was significantly correlated with serum indicators (65). Unlike previous research (65), we found here that the expression of MALAT1 did not significantly correlate with liver function indicators, such as alanine aminotransferase (ALT), aspartate aminotransferase (AST), or total bilirubin. The reason might be related to the differences in the types of the samples analyzed (e.g., tissue or plasma), and the clinicopathological features of the patients enrolled (e.g., type of viral hepatitis, presence or absence of liver cirrhosis). Since MALAT1 did not have covariance with significant prognostic factors including size of tumor, number of tumors, tumor stage, and gender, we constructed prediction models for progression rate and survival rate based on MALAT1 and those factors. The prognostic models showed moderate performance in predicting progression and survival, and the models outperformed individual indicators. These models may be a new method in the clinic to predict the outcome of HCC patients.
The main limitation of this study was also its strength: we investigated aspects of interacting proteins, ceRNAs, somatic mutations, methylation, and tumor-related features based on multi-omics data. While our analyses had a broad reach, they were difficult to integrate together in order to identify deep relationships. Future research should focus on the more promising findings to follow up in mechanistic studies. Secondly, there were some discrepancies between the results of the survival analysis based on TCGA-LIHC in this study and the analysis based on the samples we collected. These could be due to the fact that the patients in our collected sample underwent radical resection, whereas those in the TCGA-LIHC may have undergone a variety of radical or partial surgeries. The two cohorts may also have differed in additional treatments. The discrepancies may also be due to ethnic, environmental, and socioeconomic differences.
Conclusion
MALAT1 expression was significantly higher in hepatocellular carcinoma, and patients with a high expression level had a worse prognosis than those with a low expression level. MALAT1 could serve as a prognostic marker for patients with hepatocellular carcinoma who have undergone liver resection.
Data availability statement
The raw data supporting the conclusion of this article will be made available by the authors, without undue reservation.
Ethics statement
The studies involving human participants were reviewed and approved by the ethics committee at the Affiliated Tumor Hospital of Guangxi Medical University, and verbal informed consent was obtained from all the patients.
Author contributions
Conception/design: WH and WX. Provision of study materials: BL and JC. Collection and/or assembly of data: XL and DL. Analysis: XL, JC, and DL. Manuscript writing: XL, JC, and DL. Supervision and project administration: WH and WX. All authors contributed to the article and approved the submitted version.
Funding
This research was supported by the National Natural Science Foundation of China (Grant Number: 81060169), Guangxi Natural Science Foundation (Grant Number: 2017GXNSFBA198041), Guangxi Science and Technology Research Project (Grant Number: GUIKEGONG 16380215). The Guangxi Medical and Health Appropriate Technology Development and Application Project (Grant No. S2020100). The Self-Raised Funds of Guangxi Health Department (Grant No. Z20210852). The Guangxi Natural Science Foundation (Grant No. 2017GXNSFAA198103).
Conflict of interest
The authors declare that the research was conducted in the absence of any commercial or financial relationships that could be construed as a potential conflict of interest.
Supplementary material
The Supplementary Material for this article can be found online at: https://www.por-journal.com/articles/10.3389/pore.2022.1610808/full#supplementary-material
References
1. Bray, F, Ferlay, J, Soerjomataram, I, Siegel, RL, Torre, LA, and Jemal, A. Global cancer statistics 2018: GLOBOCAN estimates of incidence and mortality worldwide for 36 cancers in 185 countries. CA Cancer J Clin (2018) 68(6):394–424. doi:10.3322/caac.21492
2. Sun, D, Cao, M, Li, H, He, S, and Chen, W. Cancer burden and trends in China: A review and comparison with Japan and South Korea. Chin J Cancer Res (2020) 32(2):129–39. doi:10.21147/j.issn.1000-9604.2020.02.01
3. Chen, LT, Martinelli, E, Cheng, AL, Pentheroudakis, G, Qin, S, Bhattacharyya, GS, et al. Pan-asian adapted ESMO clinical practice guidelines for the management of patients with intermediate and advanced/relapsed hepatocellular carcinoma: A TOS-ESMO initiative endorsed by CSCO, ISMPO, JSMO, KSMO, MOS and SSO. Ann Oncol (2020) 31(3):334–51. doi:10.1016/j.annonc.2019.12.001
4. Marrero, JA, Kulik, LM, Sirlin, CB, Zhu, AX, Finn, RS, Abecassis, MM, et al. Diagnosis, staging, and management of hepatocellular carcinoma: 2018 practice guidance by the American association for the study of liver diseases. Hepatology (2018) 68(2):723–50. doi:10.1002/hep.29913
5. Hilmi, M, Neuzillet, C, Calderaro, J, Lafdil, F, Pawlotsky, JM, and Rousseau, B. Angiogenesis and immune checkpoint inhibitors as therapies for hepatocellular carcinoma: Current knowledge and future research directions. J Immunother Cancer (2019) 7(1):333. doi:10.1186/s40425-019-0824-5
6. Pinter, M, Scheiner, B, and Peck-Radosavljevic, M. Immunotherapy for advanced hepatocellular carcinoma: A focus on special subgroups. Gut (2021) 70(1):204–14. doi:10.1136/gutjnl-2020-321702
7. Makary, MS, Khandpur, U, Cloyd, JM, Mumtaz, K, and Dowell, JD. Locoregional therapy approaches for hepatocellular carcinoma: Recent advances and management strategies. Cancers (Basel) (2020) 12(7):1914. doi:10.3390/cancers12071914
8. Yarchoan, M, Agarwal, P, Villanueva, A, Rao, S, Dawson, LA, Llovet, JM, et al. Recent developments and therapeutic strategies against hepatocellular carcinoma. Cancer Res (2019) 79(17):4326–30. doi:10.1158/0008-5472.Can-19-0803
9. Vogel, A, Cervantes, A, Chau, I, Meyer, T, Llovet, JM, Ricke, J, et al. Correction to: "Hepatocellular carcinoma: ESMO clinical practice guidelines for diagnosis, treatment and follow-up". Ann Oncol (2019) 30(5):871–3. doi:10.1093/annonc/mdy510
10. Piñero, F, Dirchwolf, M, and Pessôa, MG. Biomarkers in hepatocellular carcinoma: Diagnosis, prognosis and treatment response assessment. Cells (2020) 9(6):1370. doi:10.3390/cells9061370
11. Huang, Z, Zhou, JK, Peng, Y, He, W, and Huang, C. The role of long noncoding RNAs in hepatocellular carcinoma. Mol Cancer (2020) 19(1):77. doi:10.1186/s12943-020-01188-4
12. Forrest, ME, and Khalil, AM. Review: Regulation of the cancer epigenome by long non-coding RNAs. Cancer Lett (2017) 407:106–12. doi:10.1016/j.canlet.2017.03.040
13. Lee, JT. Epigenetic regulation by long noncoding RNAs. Science (2012) 338(6113):1435–9. doi:10.1126/science.1231776
14. Salmena, L, Poliseno, L, Tay, Y, Kats, L, and Pandolfi, PP. A ceRNA hypothesis: The rosetta stone of a hidden RNA language? Cell (2011) 146(3):353–8. doi:10.1016/j.cell.2011.07.014
15. Huang, W, Li, H, Yu, Q, Xiao, W, and Wang, DO. LncRNA-mediated DNA methylation: An emerging mechanism in cancer and beyond. J Exp Clin Cancer Res (2022) 41(1):100. doi:10.1186/s13046-022-02319-z
16. He, S, Chen, J, Gao, H, Yang, G, Zhang, F, Ding, Y, et al. Extended transcriptome analysis reveals genome-wide lncRNA-mediated epigenetic dysregulation in colorectal cancer. Comput Struct Biotechnol J (2020) 18:3507–17. doi:10.1016/j.csbj.2020.11.004
17. Sun, Y, and Ma, L. New insights into long non-coding RNA MALAT1 in cancer and metastasis. Cancers (Basel) (2019) 11(2):216. doi:10.3390/cancers11020216
18. Lei, L, Chen, J, Huang, J, Lu, J, Pei, S, Ding, S, et al. Functions and regulatory mechanisms of metastasis-associated lung adenocarcinoma transcript 1. J Cel Physiol (2018) 234(1):134–51. doi:10.1002/jcp.26759
19. Wang, S, and Liu, X. The UCSCXenaTools R package: A toolkit for accessing genomics data from UCSC xena platform, from cancer multi-omics to single-cell RNA-seq. J Open Source Softw (2019) 4(40):1627. doi:10.21105/joss.01627
20. Schmittgen, TD, and Livak, KJ. Analyzing real-time PCR data by the comparative C(T) method. Nat Protoc (2008) 3(6):1101–8. doi:10.1038/nprot.2008.73
21. Ji, P, Diederichs, S, Wang, W, Boing, S, Metzger, R, Schneider, PM, et al. MALAT-1, a novel noncoding RNA, and thymosin beta4 predict metastasis and survival in early-stage non-small cell lung cancer. Oncogene (2003) 22(39):8031–41. doi:10.1038/sj.onc.1206928
22. Cao, X, Zhao, R, Chen, Q, Zhao, Y, Zhang, B, Zhang, Y, et al. MALAT1 might be a predictive marker of poor prognosis in patients who underwent radical resection of middle thoracic esophageal squamous cell carcinoma. Cancer Biomark (2015) 15(6):717–23. doi:10.3233/cbm-150513
23. Liu, M, Yang, P, Mao, G, Deng, J, Peng, G, Ning, X, et al. Long non-coding RNA MALAT1 as a valuable biomarker for prognosis in osteosarcoma: A systematic review and meta-analysis. Int J Surg (2019) 72:206–13. doi:10.1016/j.ijsu.2019.11.004
24. Lai, MC, Yang, Z, Zhou, L, Zhu, Q, Xie, H, Zhang, F, et al. Long non-coding RNA MALAT-1 overexpression predicts tumor recurrence of hepatocellular carcinoma after liver transplantation. Med Oncol (2012) 29(3):1810–6. doi:10.1007/s12032-011-0004-z
25. Sonohara, F, Inokawa, Y, Hayashi, M, Yamada, S, Sugimoto, H, Fujii, T, et al. Prognostic value of long non-coding RNA HULC and MALAT1 following the curative resection of hepatocellular carcinoma. Sci Rep (2017) 237(1):16142. doi:10.1038/s41598-017-16260-1
26. Badalamenti, G, Barraco, N, Incorvaia, L, Galvano, A, Fanale, D, Cabibi, D, et al. Are long noncoding RNAs new potential biomarkers in gastrointestinal stromal tumors (GISTs)? The role of H19 and MALAT1. J Oncol (2019) 2019:5458717. doi:10.1155/2019/5458717
27. Ye, ZL, Huang, Y, Li, LF, Zhu, H, Gao, H, Liu, H, et al. Argonaute 2 promotes angiogenesis via the PTEN/VEGF signaling pathway in human hepatocellular carcinoma. Acta Pharmacol Sin (2015) 36(10):1237–45. doi:10.1038/aps.2015.18
28. Yang, Y, and Mei, Q. Accumulation of AGO2 facilitates tumorigenesis of human hepatocellular carcinoma. Biomed Res Int (2020) 2020:1631843. doi:10.1155/2020/1631843
29. Hu, J, Cai, D, Zhao, Z, Zhong, GC, and Gong, J. Suppression of heterogeneous nuclear ribonucleoprotein C inhibit hepatocellular carcinoma proliferation, migration, and invasion via ras/MAPK signaling pathway. Front Oncol (2021) 11:659676. doi:10.3389/fonc.2021.659676
30. Zhou, J, Che, J, Xu, L, Yang, W, Li, Y, Zhou, W, et al. Enhancer of zeste homolog 2 promotes hepatocellular cancer progression and chemoresistance by enhancing protein kinase B activation through microRNA-381-mediated SET domain bifurcated 1. Bioengineered (2022) 13(3):5737–55. doi:10.1080/21655979.2021.2023792
31. Ding, H, Liu, J, Wang, C, and Su, Y. NONO promotes hepatocellular carcinoma progression by enhancing fatty acids biosynthesis through interacting with ACLY mRNA. Cancer Cel Int (2020) 20:425. doi:10.1186/s12935-020-01520-4
32. Lei, S, Zhang, B, Huang, L, Zheng, Z, Xie, S, Shen, L, et al. SRSF1 promotes the inclusion of exon 3 of SRA1 and the invasion of hepatocellular carcinoma cells by interacting with exon 3 of SRA1pre-mRNA. Cell Death Discov (2021) 7(1):117. doi:10.1038/s41420-021-00498-w
33. Malakar, P, Shilo, A, Mogilevsky, A, Stein, I, Pikarsky, E, Nevo, Y, et al. Long noncoding RNA MALAT1 promotes hepatocellular carcinoma development by SRSF1 upregulation and mTOR activation. Cancer Res (2017) 77(5):1155–67. doi:10.1158/0008-5472.Can-16-1508
34. Zhang, P, Ha, M, Li, L, Huang, X, and Liu, C. MicroRNA-3064-5p sponged by MALAT1 suppresses angiogenesis in human hepatocellular carcinoma by targeting the FOXA1/CD24/Src pathway. Faseb j. (2020) 34(1):66–81. doi:10.1096/fj.201901834R
35. Peng, N, He, J, Li, J, Huang, H, Huang, W, Liao, Y, et al. Long noncoding RNA MALAT1 inhibits the apoptosis and autophagy of hepatocellular carcinoma cell by targeting the microRNA-146a/PI3K/Akt/mTOR axis. Cancer Cel Int (2020) 20:165. doi:10.1186/s12935-020-01231-w
36. Chen, F, Zhong, Z, Tan, HY, Guo, W, Zhang, C, Cheng, CS, et al. Suppression of lncRNA MALAT1 by betulinic acid inhibits hepatocellular carcinoma progression by targeting IAPs via miR-22-3p. Clin Transl Med (2020) 10(6):e190. doi:10.1002/ctm2.190
37. Chen, L, Yao, H, Wang, K, and Liu, X. Long non-coding RNA MALAT1 regulates ZEB1 expression by sponging miR-143-3p and promotes hepatocellular carcinoma progression. J Cel Biochem. (2017) 118(12):4836–43. doi:10.1002/jcb.26158
38. Liu, S, Qiu, J, He, G, Liang, Y, Wang, L, Liu, C, et al. LncRNA MALAT1 acts as a miR-125a-3p sponge to regulate FOXM1 expression and promote hepatocellular carcinoma progression. J Cancer (2019) 10(26):6649–59. doi:10.7150/jca.29213
39. Gillespie, M, Jassal, B, Stephan, R, Milacic, M, Rothfels, K, Senff-Ribeiro, A, et al. The reactome pathway knowledgebase 2022. Nucleic Acids Res (2022) 50(D1):D687–D692. doi:10.1093/nar/gkab1028
40. Varallo-Rodriguez, C, Freyer, CW, Ontiveros, EP, Griffiths, EA, Wang, ES, and Wetzler, M. Bosutinib for the treatment of philadelphia chromosome-positive leukemias. Expert Opin Orphan Drugs (2015) 3(5):599–608. doi:10.1517/21678707.2015.1036027
41. Celià-Terrassa, T, Liu, DD, Choudhury, A, Hang, X, Wei, Y, Zamalloa, J, et al. Normal and cancerous mammary stem cells evade interferon-induced constraint through the miR-199a-LCOR axis. Nat Cel Biol. (2017) 19(6):711–23. doi:10.1038/ncb3533
42. Vogelsang, TLR, Schmoeckel, E, Kuhn, C, Blankenstein, T, Temelkov, M, Heidegger, H, et al. Regulation of LCoR and RIP140 expression in cervical intraepithelial neoplasia and correlation with CIN progression and dedifferentiation. J Cancer Res Clin Oncol (2020) 146(7):1847–55. doi:10.1007/s00432-020-03178-x
43. Yamamoto, N, Nishikawa, R, Chiyomaru, T, Goto, Y, Fukumoto, I, Usui, H, et al. The tumor-suppressive microRNA-1/133a cluster targets PDE7A and inhibits cancer cell migration and invasion in endometrial cancer. Int J Oncol (2015) 47(1):325–34. doi:10.3892/ijo.2015.2986
44. Lv, P, Yang, S, Liu, W, Qin, H, Tang, X, Wu, F, et al. Circulating plasma lncRNAs as novel markers of EGFR mutation status and monitors of epidermal growth factor receptor-tyrosine kinase inhibitor therapy. Thorac Cancer (2020) 11(1):29–40. doi:10.1111/1759-7714.13216
45. Ak Aksoy, S, Mutlu, M, Tunca, B, Kocaeli, H, Taskapilioglu, MO, Bekar, A, et al. Coexistence of TERT C228T mutation and MALAT1 dysregulation in primary glioblastoma: New prognostic and therapeutic targets. Neurol Res (2021) 43(11):916–25. doi:10.1080/01616412.2021.1948738
46. Zhan, P, Ji, YN, and Yu, LK. TP53 mutation is associated with a poor outcome for patients with hepatocellular carcinoma: Evidence from a meta-analysis. Hepatobiliary Surg Nutr (2013) 2(5):260–5. doi:10.3978/j.issn.2304-3881.2013.07.06
47. Tischoff, I, and Tannapfe, A. DNA methylation in hepatocellular carcinoma. World J Gastroenterol (2008) 14(11):1741–8. doi:10.3748/wjg.14.1741
48. Liu, A, Wu, Q, Peng, D, Ares, I, Anadon, A, Lopez-Torres, B, et al. A novel strategy for the diagnosis, prognosis, treatment, and chemoresistance of hepatocellular carcinoma: DNA methylation. Med Res Rev (2020) 40(5):1973–2018. doi:10.1002/med.21696
49. Dai, X, Liu, L, Liang, Z, Guo, K, Xu, S, and Wang, H. Silencing of lncRNA MALAT1 inhibits cell cycle progression via androgen receptor signaling in prostate cancer cells. Pathol Res Pract (2019) 215(4):712–21. doi:10.1016/j.prp.2019.01.011
50. Tripathi, V, Shen, Z, Chakraborty, A, Giri, S, Freier, SM, Wu, X, et al. Long noncoding RNA MALAT1 controls cell cycle progression by regulating the expression of oncogenic transcription factor B-MYB. Plos Genet (2013) 9(3):e1003368. doi:10.1371/journal.pgen.1003368
51. Samimi, H, Haghpanah, V, Irani, S, Arefian, E, Sohi, AN, Fallah, P, et al. Transcript-level regulation of MALAT1-mediated cell cycle and apoptosis genes using dual MEK/Aurora kinase inhibitor "BI-847325" on anaplastic thyroid carcinoma. Daru. (2019) 27(1):1–7. doi:10.1007/s40199-018-0231-3
52. Huang, J, Lin, C, Dong, H, Piao, Z, Jin, C, Han, H, et al. Targeting MALAT1 induces DNA damage and sensitize non-small cell lung cancer cells to cisplatin by repressing BRCA1. Cancer Chemother Pharmacol (2020) 86(5):663–72. doi:10.1007/s00280-020-04152-7
53. Hu, Y, Lin, J, Fang, J, Fang, H, Li, C, Chen, W, et al. Targeting the MALAT1/PARP1/LIG3 complex induces DNA damage and apoptosis in multiple myeloma. Leukemia (2018) 32(10):2250–62. doi:10.1038/s41375-018-0104-2
54. Hardin, H, Helein, H, Meyer, K, Robertson, S, Zhang, R, Zhong, W, et al. Thyroid cancer stem-like cell exosomes: Regulation of EMT via transfer of lncRNAs. Lab Invest (2018) 98(9):1133–42. doi:10.1038/s41374-018-0065-0
55. Klinge, CM. Non-coding RNAs in breast cancer: Intracellular and intercellular communication. Noncoding RNA (2018) 4(4):40. doi:10.3390/ncrna4040040
56. Liang, Z, Wu, Q, Wang, H, Tan, J, Gou, Y, Cao, Y, et al. Silencing of lncRNA MALAT1 facilitates erastin-induced ferroptosis in endometriosis through miR-145-5p/MUC1 signaling. Cel Death Discov (2022) 8(1):190. doi:10.1038/s41420-022-00975-w
57. Wang, Z, Wang, X, Zhang, T, Su, L, Liu, B, Zhu, Z, et al. LncRNA MALAT1 promotes gastric cancer progression via inhibiting autophagic flux and inducing fibroblast activation. Cell Death Dis (2021) 12(4):368. doi:10.1038/s41419-021-03645-4
58. Hou, ZH, Xu, XW, Fu, XY, Zhou, LD, Liu, SP, and Tan, DM. Long non-coding RNA MALAT1 promotes angiogenesis and immunosuppressive properties of HCC cells by sponging miR-140. Am J Physiol Cel Physiol. (2020) 318(3):C649–c663. doi:10.1152/ajpcell.00510.2018
59. Chang, HL, Bamodu, OA, Ong, JR, Lee, WH, Yeh, CT, and Tsai, JT. Targeting the epigenetic non-coding RNA MALAT1/wnt signaling Axis as a therapeutic approach to suppress stemness and metastasis in hepatocellular carcinoma. Cells (2020) 9(4):1020. doi:10.3390/cells9041020
60. Malakar, P, Stein, I, Saragovi, A, Winkler, R, Stern-Ginossar, N, Berger, M, et al. Long noncoding RNA MALAT1 regulates cancer glucose metabolism by enhancing mTOR-mediated translation of TCF7L2. Cancer Res (2019) 79(10):2480–93. doi:10.1158/0008-5472.Can-18-1432
61. He, B, Peng, F, Li, W, and Jiang, Y. Interaction of lncRNA-MALAT1 and miR-124 regulates HBx-induced cancer stem cell properties in HepG2 through PI3K/Akt signaling. J Cel Biochem. (2019) 120(3):2908–18. doi:10.1002/jcb.26823
62. Chen, S, Wang, G, Tao, K, Cai, K, Wu, K, Ye, L, et al. Long noncoding RNA metastasis-associated lung adenocarcinoma transcript 1 cooperates with enhancer of zeste homolog 2 to promote hepatocellular carcinoma development by modulating the microRNA-22/Snail family transcriptional repressor 1 axis. Cancer Sci (2020) 111(5):1582–95. doi:10.1111/cas.14372
63. Fattovich, G, Stroffolini, T, Zagni, I, and Donato, F. Hepatocellular carcinoma in cirrhosis: Incidence and risk factors. Gastroenterology (2004) 127(5):S35–50. doi:10.1053/j.gastro.2004.09.014
64. Yuan, LT, Chang, JH, Lee, HL, Yang, YC, Su, SC, Lin, CL, et al. Genetic variants of lncRNA MALAT1 exert diverse impacts on the risk and clinicopathologic characteristics of patients with hepatocellular carcinoma. J Clin Med (2019) 8(9):1406. doi:10.3390/jcm8091406
Keywords: prognosis, hepatocellular carcinoma, mutation, DNA methylation, metastasis associated lung adenocarcinoma transcript 1 (MALAT1), interacting proteins, competing endogenous RNAs (ceRNAs), multi-omics
Citation: Liao X, Chen J, Luo D, Luo B, Huang W and Xie W (2023) Prognostic value of long non-coding RNA MALAT1 in hepatocellular carcinoma: A study based on multi-omics analysis and RT-PCR validation. Pathol. Oncol. Res. 28:1610808. doi: 10.3389/pore.2022.1610808
Received: 02 September 2022; Accepted: 16 December 2022;
Published: 04 January 2023.
Edited by:
Andrea Ladányi, National Institute of Oncology (NIO), HungaryCopyright © 2023 Liao, Chen, Luo, Luo, Huang and Xie. This is an open-access article distributed under the terms of the Creative Commons Attribution License (CC BY). The use, distribution or reproduction in other forums is permitted, provided the original author(s) and the copyright owner(s) are credited and that the original publication in this journal is cited, in accordance with accepted academic practice. No use, distribution or reproduction is permitted which does not comply with these terms.
*Correspondence: Wenfeng Huang, ZG92ZS13ZW5mZW5nQDE2My5jb20=; Weimin Xie, eGlld2VpbWluQGd4bXUuZWR1LmNu
†These authors have contributed equally to this work