- 1College of Chinese Medicine, Shandong University of Traditional Chinese Medicine, Jinan, China
- 2The First Clinical Medical College, Shandong University of Traditional Chinese Medicine, Jinan, China
- 3Columbus Technical College, Columbus, GA, United States
- 4College of Basic Medicine, Qingdao University, Qingdao, China
Background: In view of the high malignancy and poor prognosis of human epidermal growth factor receptor 2 (HER2)-positive breast cancer, we analyzed the RNA expression profiles of HER2-positive breast cancer samples to identify the new prognostic biomarkers.
Methods: The linear fitting method was used to identify the differentially expressed RNAs from the HER2-positive breast cancer RNA expression profiles in the Cancer Genome Atlas (TCGA). Then, a series of methods including univariate Cox, Kaplan-Meier, and random forests, were used to identify the core long non-coding RNAs (lncRNAs) with stable prognostic value for HER2-positive breast cancer. A clinical feature analysis was performed, and a competing endogenous RNA network was constructed to explore the role of these core lncRNAs in HER2-positive breast cancer. In addition, a functional analysis of differentially expressed messenger RNAs in HER-2 positive breast cancer also provided us with some enlightening insights.
Results: The high expression of four core lncRNAs (AC010595.1, AC046168.1, AC069277.1, and AP000904.1) was associated with worse overall survival, while the low expression of LINC00528 and MIR762HG was associated with worse overall survival. The 6-lncRNA model has an especially good predictive power for overall survival (p < 0.0001) and 3-year survival (the area under the curve = 0.980) in HER2-positive breast cancer patients.
Conclusion: This study provides a new efficient prognostic model and biomarkers of HER2-positive breast cancer. Meanwhile, it also provides a new perspective for elucidating the molecular mechanisms underlying HER2-positive breast cancer.
Introduction
About 15–20% of breast cancers are HER2 positive [1]. HER2 positivity, namely HER2 protein overexpression, is an important indicator of strong tumor aggressiveness and poor prognosis [2]. Due to the strong heterogeneity of breast cancer, the prognosis and treatment of HER2-positive breast cancer are significantly different from other breast cancer subgroups [3, 4]. Although new treatments have improved HER2-positive breast cancer prognosis in recent years, its persistently high mortality indicates that the situation is still alarming. Therefore, the identification of new HER2-positive breast cancer specific biomarkers is particularly urgent.
Recent comprehensive transcriptome analysis revealed that there is a specific lncRNA expression pattern in different subtypes of breast cancer [5]. The discovery of lncRNAs, ranging in length from 200 nucleotides to 100 kilobases, has helped scientists break free from their conventional view that gene regulation in biology mainly involves protein-coding genes [6, 7]. LncRNAs can regulate gene expression at the transcriptional, post-transcriptional and epigenetic levels, which in turn affects the clinical course of the disease [8]. Compared with mRNAs and microRNAs (miRNAs), lncRNAs may be a direct indicator of tumor status [9, 10]. Studies have shown that the function of lncRNAs is associated with disease progression and patient survival of cancers, such as non-small cell lung cancer, prostate cancer and gastric cancer [11–13]. Previous studies have established prognostic models for breast cancer through mRNA and alternative splicing [14, 15], but the establishment of a lncRNA system to predict HER2 positive breast cancer patients’ prognosis is hardly seen.
In this study, we applied a series of reliable bioinformatics methods to analyze the transcriptome data of HER2-positive breast cancer. Six core lncRNAs associated with stable prognosis value were identified using the randomForestSRC package and a good prognostic model was constructed. To explore the role of these core lncRNAs in HER2-positive breast cancer, we constructed a corresponding ceRNA network and performed a clinical feature analysis. In addition, we performed functional analysis of differentially expressed mRNAs and assessed the prognostic value of the core lncRNAs in various tumors in Gene Expression Profiling Interactive Analysis (GEPIA) for more comprehensive information. Features recognized in this study also helped us gain further understanding of the biological behavior of HER2-positive breast cancer at the molecular level.
Materials and Methods
Downloading of Human Epidermal Growth Factor Receptor 2-Positive Breast Cancer RNA Expression Data and the Corresponding Clinical Data
Highly developed microarrays and high-throughput sequencing technologies have lent powerful support for deciphering cancer genetic code [16]. TCGA provides unprecedented large-scale data for cancer researches, which provide great opportunities for revealing genomic variation in a variety of tumors [17]. RNA expression profile data of HER2-positive breast cancer samples including lncRNA, miRNA, and mRNA sequence information, were downloaded from TCGA (https://portal.gdc.cancer.gov/). These RNA data were derived from 113 HER2-positive breast cancer tissues and 105 tumor-adjacent normal breast tissues. The corresponding clinical data of the 113 HER2-positive breast cancer patients were also downloaded (Supplementary Table S1). This study has strictly followed the TCGA publication guidelines (https://www.cancer.gov/about-nci/organization/ccg/research/structural-genomics/tcga/using-tcga/citing-tcga) and all data are available without restrictions on their use in publications.
Identification of Differentially Expressed RNAs in Human Epidermal Growth Factor Receptor 2-Positive Breast Cancer and the Functional Analysis of Differentially Expressed Messenger RNAs
We used the limma package [18] to identify differentially expressed RNAs (including lncRNAs, miRNAs, and mRNAs) between the 113 HER2-positive breast cancer tissues and 105 tumor-adjacent normal breast tissues. Then we selected the differentially expressed mRNAs for functional enrichment analysis including gene ontology (GO) analysis and Kyoto Encyclopedia of Genes and Genomes (KEGG) analysis in the R language environment [19].
Construction and Analysis of the Protein-Protein Interaction Network
The Search Tool for the Retrieval of Interacting Genes (STRING) database (https://string-db.org) was used to construct a protein-protein interaction (PPI) network [20]. We input the names of differentially expressed mRNAs into the multiple proteins option input box of STRING database in the form of a list and selected “homo” for “species.” The interaction score of nodes was greater than 0.4. The PPI network was downloaded and visualized in Cytoscape. Furthermore, Cytoscape-MCODE was used to perform module analysis on the PPI network [21, 22]. Modules with score >4 and nodes ≥5 were selected for the functional enrichment analysis in the STRING database. Cytoscape-Centiscape was used to analyze the internal connectivity of the PPI network [23]. The statistical criterion for functional enrichment analysis was FDR <0.05.
Identification of Long Non-Coding RNAs With Prognostic Value for Human Epidermal Growth Factor Receptor 2-Positive Breast Cancer
First, we selected lncRNAs with prognostic value for HER2-positive breast cancer patients by applying two methods, including univariate Cox regression analysis and Kaplan-Meier survival analysis (statistical criterion: p < 0.05). To achieve that, the survival package was used [24]. Then the overlap of the prognostic lncRNAs resulted from the above two methods was obtained. Next, the randomForestSRC package [25] was used to perform the high-dimensional variable selection for survival data on the overlapped lncRNAs, and lncRNAs with stable prognostic value for HER2-positive breast cancer were selected as core lncRNAs for subsequent analysis. Random forests is one of the classical methods for dimensionality reduction, and its results are highly reliable.
Construction of the Prognostic Model for Human Epidermal Growth Factor Receptor 2-Positive Breast Cancer
The multiCox model was constructed using the core lncRNAs. This model was used to predict the survival risk of HER2-positive patients. The 113 HER2-positive breast cancer samples from TCGA were classified into high-risk and low-risk groups, with the median signature risk score as the cut-off value. Furthermore, we used the receiver operating characteristic (ROC) curve to evaluate the predictability of the 6-lncRNA model for the 3-year survival rate for HER2-positive breast cancer. To achieve that, the survivalROC package was used [26]. The results of the grouping, according to the classification, were compared with the actual 3-year survival status of HER2-positive breast cancer patients to obtain a false positive rate and a true positive rate. These rates were respectively represented on the abscissa (X axis) and the ordinate (Y axis) to plot the ROC curves. AUC, the area under the curve, was calculated as a measure of prognostic ability. The greater the AUC, the better the predictive ability. The risk score is the sum of the Cox regression coefficient multiplied by the expression value of each core lncRNA. The calculation formula is as follows:
Construction of the Competing Endogenous RNA Network of Core Long Non-Coding RNAs Based on Weighted Gene Co-expression Network Analysis
The weighted gene co-expression network analysis (WGCNA) package [27] was used to identify the target RNAs of core lncRNAs to construct a ceRNA network [28, 29]. Cytoscape was used to implement visualization. The edge threshold was set to 0.02. Functional enrichment analysis (GO and KEGG analysis) was performed on the target mRNAs of each core lncRNA. A test result with adjusted p < 0.05 was considered statistically significant.
Clinical Feature Analysis of Core Long Non-Coding RNAs and Their Prognostic Value Evaluation in Various Tumors
Currently reports on these core lncRNAs are almost non-existent. To obtain more information about the role of core lncRNAs, differences in the expression of core lncRNAs between different clinical groups were evaluated by Student’s t test. The data source is the 113 HER2-positive breast cancer samples originally downloaded from TCGA. These samples have differences in gender, tumor stage, lymph node metastasis, therapy, etc. An absolute value of t > 2 was considered to be statistically significant. Meanwhile, the prognostic value of the core lncRNAs in various tumors was assessed based on GEPIA. GEPIA (http://gepia.cancer-pku.cn) is an online server based on TCGA data. It can efficiently analyze RNA-Seq datasets of various tumor samples from TCGA [30]. A test result with logrank p < 0.05 was considered statistically significant.
Results
Differentially Expressed RNAs in Human Epidermal Growth Factor Receptor 2-Positive Breast Cancer
By using the limma package, we obtained data concerning the difference in RNA expression levels in HER2-positive breast cancer. When FDR < 0.05 and |log2FC| > 1 were set as the thresholds for the significance of the gene expression difference, we identified 350 differentially expressed lncRNAs, 163 differentially expressed miRNAs, and 1910 differentially expressed mRNAs in those 113 HER2-positive breast cancer samples (Figure 1).
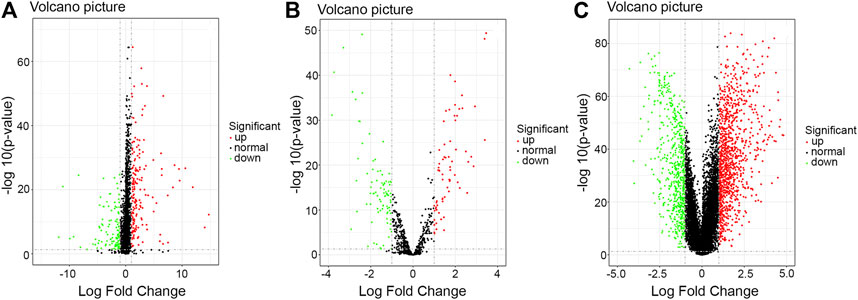
FIGURE 1. Volcano plot of the differentially expressed RNAs between HER2-positive breast cancer tissues and adjacent noncancerous breast tissues. Red: high expression; Green: low expression; Black dots: the lncRNAs with expression of |log2FC| < 1. p < 0.05. (A) lncRNA; (B) mi-RNA; (C) mRNA. Abbreviation: FC, fold change.
Results of the Functional Analysis of Differentially Expressed Messenger RNAs in Human Epidermal Growth Factor Receptor 2-Positive Breast Cancer Patients and the Analysis of the Protein-Protein Interaction Network
The number of mRNAs selected according to the statistical criteria of FDR < 0.05 and |log2FC| > 1 reached 1910. In order to improve the accuracy of this study, from that pool we further selected 411 differentially expressed mRNAs based on two criteria (|log2FC| > 2 and FDR < 0.05) for a separate functional enrichment analysis. Results with the lowest FDR values are shown in Figure 2. The PPI network downloaded from the STRING database showed that there were significant enrichment regions (Supplementary Figure S1). Cytoscape-MCODE module analysis further showed that there were six significant modules (score >4 and nodes ≥5) in the PPI network (Supplementary Figure S2), among which the following three modules were most significant: Module 1 (score = 48.857, nodes = 50), Module 2 (score = 14, nodes = 14), and Module 3 (score = 9.357, nodes = 29). Supplementary Table S2 shows the data of the module analysis downloaded from Cytoscape.
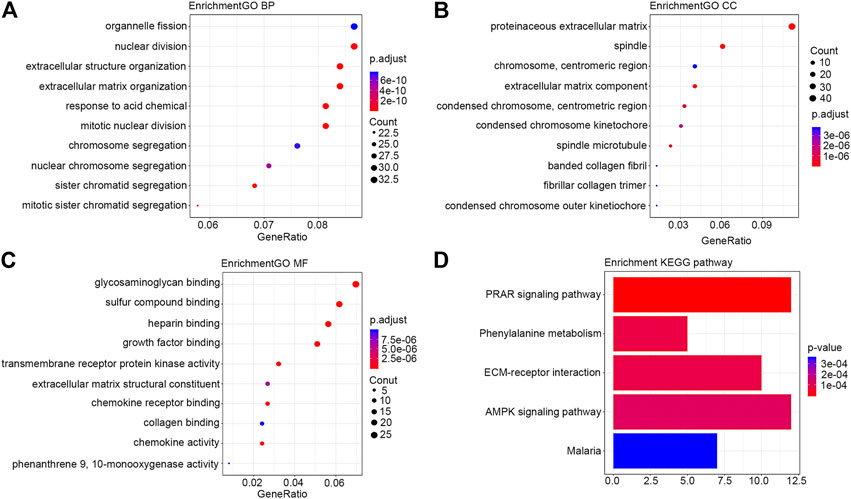
FIGURE 2. Bubble diagram of the differentially expressed mRNAs in HER2-positive breast cancer. Only results with the smallest p value are listed here. (A) BP; (B) CC; (C) MF; (D) KEGG pathway. Abbreviations: GO, gene ontology; KEGG, Kyoto Encyclopedia of Genes and Genomes; BP, biological process; MF, molecular function; CC, cell component.
Module 1 was primarily associated with mitosis. Module 2 was closely associated with immune functions. Module 3 was primarily involved in lipid metabolism. Module 4 was primarily involved in cell differentiation, stratum corneum formation, and signaling by Receptor Tyrosine Kinases. Module 5 was involved in post-translational protein phosphorylation. Module 6 participated in digestion and absorption, and degradation of the extracellular matrix. The results of the modules’ functional analysis are included in Supplementary Table S3. The connectivity analysis by Cytoscape-Centiscape of the PPI network showed that 88 differentially expressed mRNAs had a degree greater than 20, and they mainly concentrated in Module 1 (50), Module 2 (5), Module 3 (15), Module 4 (10), and Module 6 (5). The main results of the connectivity analysis are included in Supplementary Table S4.
The Core Long Non-Coding RNAs With Stable Prognostic Value for Human Epidermal Growth Factor Receptor 2-Positive Breast Cancer
There were 504 lncRNAs with significant prognostic value for HER2-positive breast cancer by the univariate Cox regression analysis. Results from 383 lncRNAs indicated significant prognostic value for HER2-positive breast cancer by Kaplan-Meier survival analysis. The number of the overlap of the lncRNAs identified by the two methods was 126. Figure 3 is plotted with the Venn online software (https://bioinfogp.cnb.csic.es/tools/venny/index.html). After a high-dimensional selection of the survival random variables was performed on the 126 lncRNAs using the randomForestSRC package, and the following six stable survival-related lncRNAs were obtained: AC010595.1, AC046168.1, AC069277.1, AP000904.1, LINC00528, and MIR762HG. In consideration of the differential expression analysis, we found that the expression levels of five core lncRNAs (AC010595.1, AC046168.1, AC069277.1, AP000904.1 and MIR762HG) in HER2-positive breast cancer tissues were higher than those in adjacent normal breast tissues (|t| > 2 and FDR <0.05), while there was no significant difference in the expression of LINC00528. Taking into account the results of clinical feature analysis, this may be because the expression level of LINC00528 in early HER2-positive breast cancer (T1/2) was higher than advanced HER2-positive breast cancer (T3/4) (|t| = 1.930803441, p = 0.060616568). The details of these six core lncRNAs are shown in Tables 1 and 2.
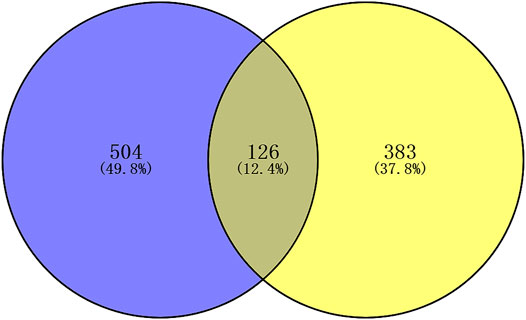
FIGURE 3. Venn plot of the prognostic lncRNAs identified by two methods. Blue: univariate Cox regression analysis; yellow: Kaplan-Meier survival analysis. p < 0.05.
The Prognostic Model of Human Epidermal Growth Factor Receptor 2-Positive Breast Cancer
Each of the six core lncRNAs had a good single-factor prognosis for overall survival and 3-year survival in HER2-positive breast cancer patients (Figure 4: p < 0.05; Figure 5: AUC > 0.7). The multiCox model of the six core lncRNAs (AC010595.1, AC046168.1, AC069277.1, AP000904.1, LINC00528, and MIR762HG) had a high-level capability of risk assessment for HER2-positive breast cancer patients. The formula for determining the risk score is given below:
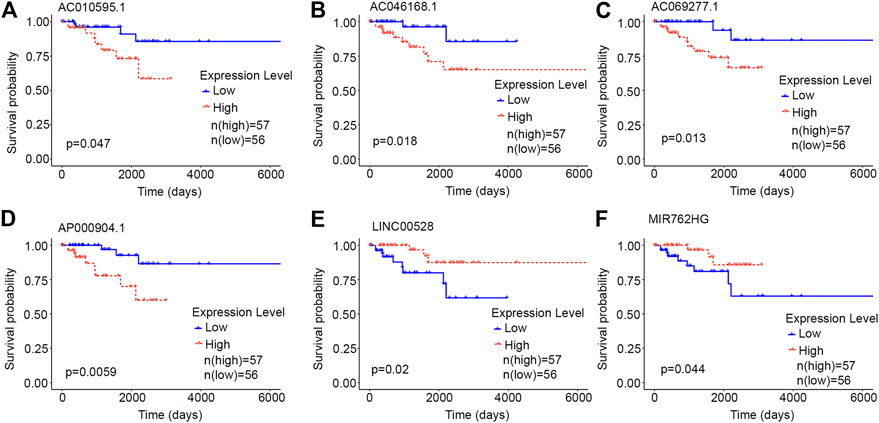
FIGURE 4. Kaplan-Meier curves of the core lncRNAs in HER2-positive breast cancer. The Kaplan-Meier curves show the prognostic significance of the core lncRNAs in patients with HER2-positive breast cancer. The red lines represent patients with a high gene expression, and blue lines represent patients with a low gene expression. (A) AC010595.1; (B) AC046168.1; (C) AC069277.1; (D) AP000904.1; (E) LINC00528; (F) MIR762HG.
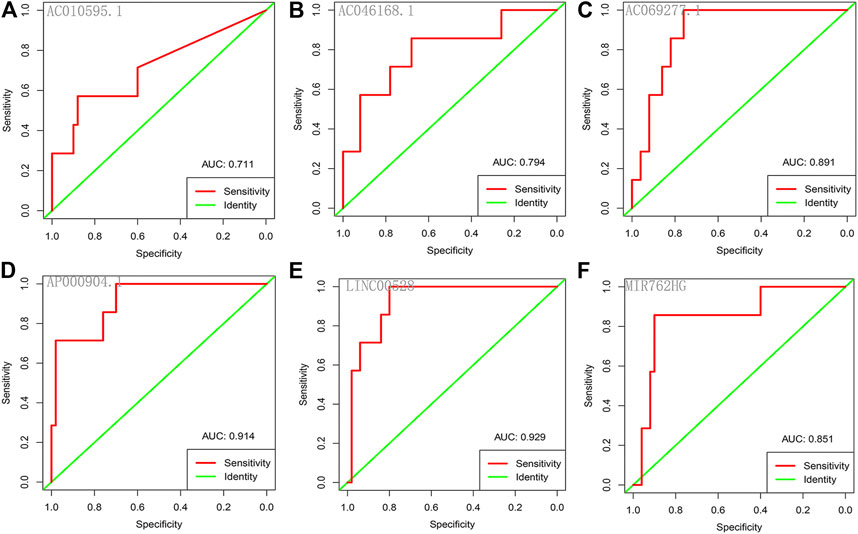
FIGURE 5. ROC curves of the core lncRNAs in HER2-positive breast cancer. The ROC curves show the core lncRNAs’ prognostic ability for 3-year survival in patients with HER2-positive breast cancer. Red: sensitivity. Green: identity. X axis (specificity): false positive rate. Y axis (sensitivity): true positive rate. AUC: area under curve. (A) AC010595.1; (B) AC046168.1; (C) AC069277.1; (D) AP000904.1; (E) LINC00528; (F) MIR762HG.
According to the median signature risk score, the 113 HER2-positive breast cancer samples from TCGA were divided into high-risk and low-risk groups with significant overall survival differences (p < 0.0001), and the ROC curve showed that the 3-year survival rates were predicted with high accuracy (AUC = 0.980) (Figure 6).
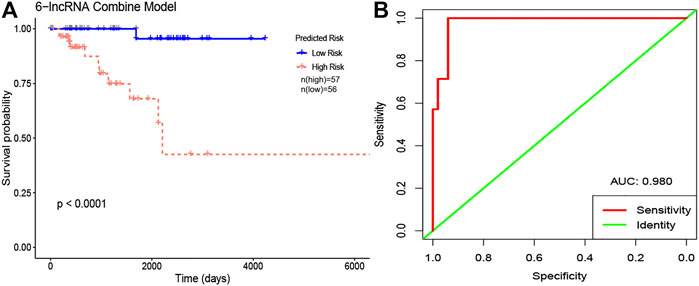
FIGURE 6. The Kaplan-Meier curve and ROC curve of 6-lncRNA combine model in HER2-positive breast cancer. (A) Kaplan-Meier curves. The red lines represent patients with high risk, and blue lines represent patients with low risk. (B) ROC curves. Red: sensitivity. Green: identity. X axis (specificity): false positive rate. Y axis (sensitivity): true positive rate. Abbreviation: AUC, area under curve.
The Competing Endogenous RNA Network of Core Long Non-Coding RNAs and Results of the Functional Enrichment Analysis
Sixteen miRNAs and 129 mRNAs were identified for the construction of the ceRNA network of core lncRNAs. Figure 7 shows the ceRNA network. Three core lncRNAs (AC010595.1, AP000904.1 and MIR762HG) were closely associated in the ceRNA network, and the functional analysis results of their target mRNAs were similar: they were involved in biological processes including muscle organ development, regulation of vasculature development, and angiogenesis. They were enriched in KEGG pathways of proteoglycans in cancer, cGMP-PKG signaling pathway. They were also closely associated with focal adhesion. Notably, the KEGG analysis showed that target mRNAs of AP000904.1 were also enriched in the PI3K-Akt signaling pathway and Ras signaling pathway. Moreover, FGF2 (|log2FC| = 2.60345034144707, FDR = 7.58730025761752E-75), the famous cancer-related gene, was found to be a target mRNA of three core lncRNAs (AC010595.1, AP000904.1 and MIR762HG) in the ceRNA network. Based on the above analysis, we also discovered that FGF2 had a high connectivity in the PPI network and was subordinate to Module 3 (degree = 58).
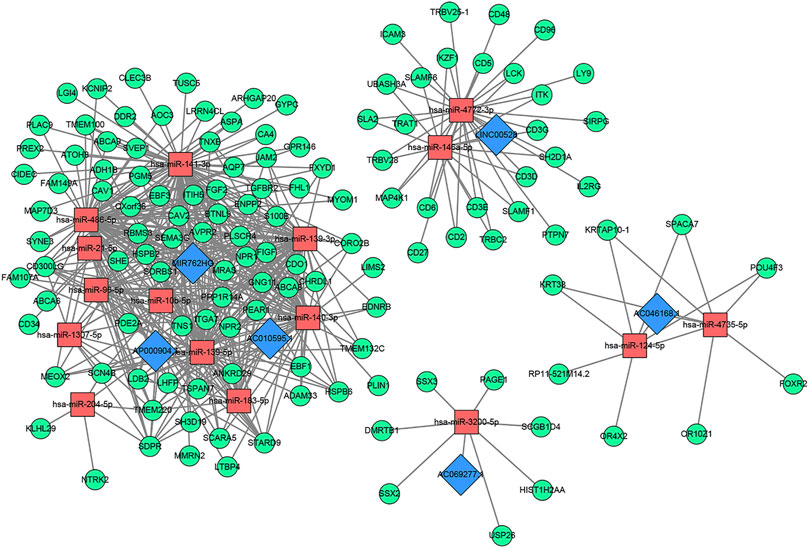
FIGURE 7. The core lncRNA-miRNA-mRNA network based on ceRNA mechanism. Blue: lncRNA; Red: miRNA; Green: mRNA.
The GO analysis showed that the target mRNAs of LINC00528 were involved in biology processes activated by immune cells such as T cells, lymphocytes and leukocytes, and were enriched in positive regulation of leukocyte cell-cell adhesion. The KEGG analysis showed that these target mRNAs were also enriched in hematopoietic cell lineage, besides being involved in primary immunodeficiency, Th1 and Th2 cell differentiation, and T cell receptor signaling pathway.
The functional analysis showed that the target mRNAs of AC046168.1 participated in the biology processes of epidermal cell differentiation, and epidermis development. The target mRNAs of AC069277.1 were associated with epigenetics, and were involved in the biology process of transcription corepressor activity and KEGG pathways of transcriptional mis-regulation in cancer, and necroptosis. Table 3 lists the primary results of the functional enrichment analysis of target mRNAs of each core lncRNA. A Q-value less than 0.05 is considered statistically significant. Supplementary Figures S3–S8 show the bubble diagrams.
Results of the Clinical Feature Analysis of Core Long Non-Coding RNAs and Their Prognostic Value in Various Tumors Based on Gene Expression Profiling Interactive Analysis
The results from the Student’s t-test showed that the six core lncRNAs were associated with some clinical parameters of HER2-positive breast cancer. It is worth noting that the expression level of LINC00528, to a certain extent, can distinguish between patients with early HER2-positive breast cancer and patients with advanced HER2-positive breast cancer: the expression level of LINC00528 in early HER2-positive breast cancer patients (T1/2) was higher than that in advanced HER2-positive breast cancer patients (T3/4) (t = 1.930803441, p = 0.060616568). Compared with non-targeted molecular therapy group, the expression level of LINC00528 was significantly higher in the targeted molecular therapy group (|t| > 2). These results are consistent with the better prognosis in the high expression group of LINC00528. The details are shown in Supplementary Table S5.
At present in GEPIA (http://gepia.cancer-pku.cn/), only relevant information of LINC00528 was available while the data of the other five core lncRNAs were not. We analyzed the prognostic value of LINC00528 for all 31 diseases included by GEPIA and found that the high expression of LINC00528 was associated with better overall survival in breast invasive carcinoma, cervical squamous cell carcinoma and endocervical adenocarcinoma, head and neck squamous cell carcinoma, lung adenocarcinoma and skin cutaneous melanoma, while the high expression of LINC00528 was associated with worse overall survival in acute myeloid leukemia, stomach adenocarcinoma and uveal melanoma (logrank p < 0.05). These results were downloaded from GEPIA. Figure 8 shows the details. The references for analysis platforms and packages were provided in Table 4.
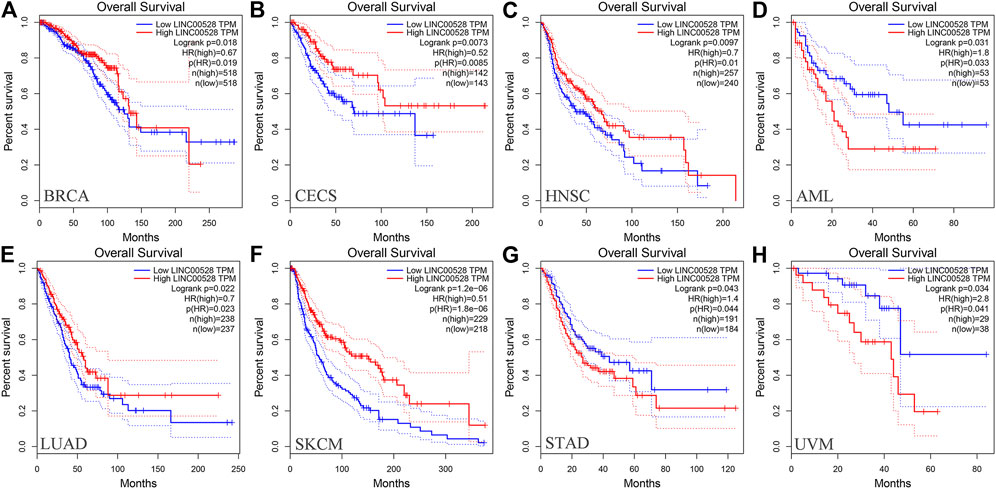
FIGURE 8. Kaplan-Meier curves of the core lncRNA LINC00528 in various cancers based on GEPIA. The Kaplan-Meier curves show the prognostic significance of LINC00528 in a variety of cancers according to the survival analysis based on GEPIA. The red lines represent patients with high gene expression, and blue lines represent patients with a low gene expression. (A) BRCA: breast invasive carcinoma; (B) CECS: cervical squamous cell carcinoma and endocervical adenocarcinoma; (C) HNSC: head and neck squamous cell carcinoma; (D) AML: acute myeloid leukemia; (E) LUAD: lung adenocarcinoma; (F) SKCM: skin cutaneous melanoma; (G) STAD: stomach adenocarcinoma; (H) UVM: uveal melanoma. Abbreviations: GEPIA, Gene Expression Profiling Interactive Analysis.
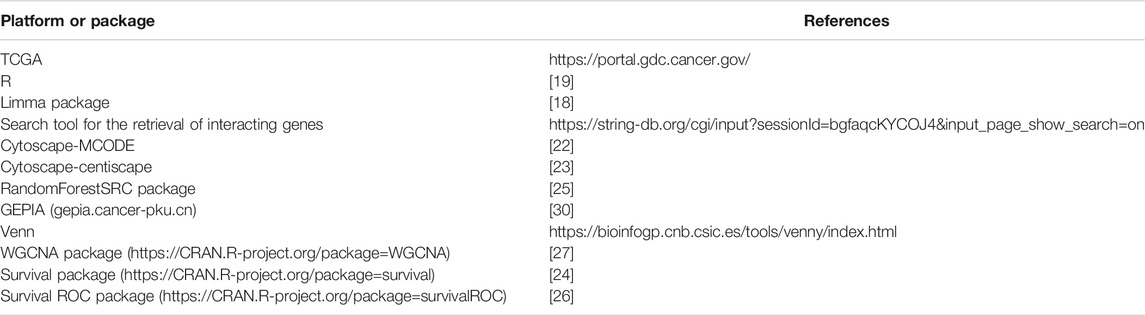
TABLE 4. The summary table with references for analysis platforms and packages used in the methodology.
Discussion
The continuous advancement of molecular oncology has made it possible to identify the genetic characteristics of tumor subtypes. These genetic characteristics are often closely associated with the activity and therapeutic opportunities of oncogenic pathways, hence becoming important biomarkers and drug targets for tumors [31]. In combination with the high heterogeneity of breast cancer genomes, the identification of subtype-specific related gene markers can provide important support for clinical strategies to improve breast cancer subtypes. Currently, advanced bioinformatics tools based on sophisticated algorithms are constantly available, allowing us to identify potential driving events in diseases from massive genomic data [32–34]. Based on transcriptome data, this study identified key biomarkers closely related to the prognosis of HER2-positive breast cancer and explored the molecular pathogenesis of HER2-positive breast cancer.
The potential of lncRNA as a prognostic factor for tumors has been recognized. As a complement to mRNAs and miRNAs, lncRNA-rich transcripts provide us with important materials for obtaining tumor prognostic factors. Through reliable analysis methods such as univariate Cox, Kaplan-Meier and random forests, we eventually identified the following six stable survival-related lncRNAs as core lncRNAs in HER2-positive breast cancer: AC010595.1, AC046168.1, AC069277.1, AP000904.1, LINC00528, and MIR762HG. We associated the high expression of four core lncRNAs (AC010595.1, AC046168.1, AC069277.1, and AP000904.1) with worse overall survival, from which we inferred that they promoted the development of cancer. The low expression of LINC00528 and MIR762HG was associated with worse overall survival, suggesting that they may have been tumor suppressor genes. Each of the core lncRNAs had good predictive power for 3-year survival, with AP000904.1 (AUC = 0.914) and LINC00528 (AUC = 0.929) being optimal.
The multi-factor regression model composed of these six core lncRNAs has a non-negligible advantage. It has a very good risk assessment ability of HER2-positive breast cancer patients (p < 0.05), and a powerful prognostic ability for 3-year survival (AUC = 0.980). This provides an effective classification tool for the clinical prognosis assessment of HER2-positive breast cancer. We also intended to test the prognostic model using an independent data set in Gene Expression Omnibus. Unfortunately, we were not able to find a fully applicable data set. This is partly because there are few related studies on these six core lncRNAs. The lack of large clinical cohorts to validate the model is one of the limitations of this study. However, considering that the six core lncRNAs in this study have shown stable prognostic value for HER2-positive breast cancer in a variety of prognostic analyses, the 6-lncRNA prognostic model is relatively reliable and worthy of further study.
Currently, non-coding regions are not fully annotated and we could hardly find any reports on these six prognostic lncRNAs. Therefore, our understanding of these core lncRNAs is not sufficiently comprehensive. More researches and tests are needed for clinical transformation. Non-coding genes usually function in complex regulatory networks [35]. To explore the functional relevance of core lncRNAs in HER2-positive breast cancer, we constructed a corresponding ceRNA network. Three core lncRNAs (AC010595.1, AP000904.1, and MIR762HG) were found closely related in the ceRNA network. Their target mRNAs were significantly enriched in the biological processes of muscle organ development, regulation of vasculature development, and angiogenesis, and closely associated with focal adhesion. In consideration of the importance of angiogenesis and cell adhesion in the pathogenesis of tumors, it could be inferred that AC010595.1, AP000904.1 and MIR762HG have jointly affected the growth and metastasis of HER2-positive breast cancer through ceRNA mechanisms. Furthermore, the famous cancer-related gene FGF2 was found to be a target mRNA of the three core lncRNAs in the ceRNA network. FGF2, an important member of the tyrosine kinase receptor family, plays a key role in the development of tumors. By reviewing the other analysis results in this study, we also identified that FGF2 was a low expressed mRNA in HER2-positive breast cancer (|log2FC| = 2.60345034144707, FDR = 7.58730025761752E-75) and occupied a core position in the PPI network (degree = 58). These newly discovered relationships between FGF2 and the core lncRNAs are valuable.
In addition, the KEGG analysis also showed AP000904.1 target mRNAs directly participated in the PI3K-Akt signaling pathway as well as the Ras signaling pathway. It is well known that these two pathways are the classical signal transduction pathways activated mainly by HER-2 and other members of the HER/ErBb receptor family after they form heterodimers. They can form cross-talk with other signaling pathways, and participate in biological functions including cell proliferation, migration, infiltration, anti-apoptosis and promotion of angiogenesis [36]. AP000904.1 is likely to be a key regulator for HER2 overexpressing tumors that deserves more attention.
The functional enrichment analysis showed that target mRNAs of LINC00528 were closely associated with immune activity and they participated in biology processes activated by various immune cells such as T cells, lymphocytes and leukocytes. LINC00528 was especially enriched in the biology processes of positive regulation of leukocyte cell-cell adhesion and regulation of leukocyte cell-cell adhesion (including multiple molecules of the CD family). This suggests that LINC00528 is closely associated with tumor cell metastasis. Based on the results of prognostic analysis and clinical characterization, we hypothesized that the low expression level of LINC00528 at the late stage is likely to be an important factor for the late metastasis of HER2-positive breast cancer. Survival analysis based on GEPIA also showed that LINC00528 had a good prognostic value in various tumors (p < 0.05), which suggested that LINC00528 could play an important role in the mechanism of cancer metastasis.
Functional analysis of differentially expressed mRNAs has also provided us with some enlightening insights. Several differentially expressed mRNAs in HER2-positive breast cancer (including CXCL2, CXCL12, CXCL10, CXCL11, CXCL9 and CXCL14) were enriched in the biology processes of chemokine receptor binding and chemokine activity. The result provides a molecular basis for elucidating the metastatic pattern of HER2-positive breast cancer. Interestingly, the differentially expressed mRNAs were significantly enriched in the malaria KEGG pathway (FDR = 0.0149807601977498). Previous studies have shown that anti-malarial drugs can cause cell cycle arrest and apoptosis in HER2-enriched breast cancer cells [37]. Furthermore, the PPI network showed modules which were significant for the exploration of the biological behavior of HER2-positive breast cancer. Module 1 was the most significant and primarily involved in mitosis, which is consistent with the characteristics of HER2 overexpression. Module 2 showed the immune regulation in HER2-positive breast cancer. Notably, Module 3 participated in the Adenosine 5′-monophosphate-activated protein kinase (AMPK) signaling pathway. AMPK as a regulator of cellular energy metabolism is an ideal target for tumor therapy [38, 39].
Conclusion
This study provides a new efficient prognostic model of HER2-positive breast cancer. The 6-lncRNA prognostic model is relatively reliable and worthy of further study. The six core lncRNAs are also potential drug targets. Currently there are almost no reports regarding the six core lncRNAs. This study has thus laid a foundation for a further understanding of the roles of these core lncRNAs and for obtaining the key pathways. Meanwhile, it provides a new perspective for elucidating the molecular mechanisms underlying HER2-positive breast cancer.
Data Availability Statement
Publicly available datasets were analyzed in this study. This data can be found here: https://portal.gdc.cancer.gov/. Code availability: We conducted data analysis in R (3.4.0) language environment. The R packages used are available on the website: https://mirrors.tuna.tsinghua.edu.cn/CRAN/. We also applied GEPIA (http://gepia.cancer-pku.cn/) for further analysis, which has been explained appropriately in the paper.
Author Contributions
XZ and JW were involved in the concept and design of the study. XZ drafted the manuscript. All authors were involved in acquisition, analysis, and interpretation of the data, revised the manuscript, and approved the final version.
Funding
This work is supported by the National Natural Science Foundation of China, Grant Nos. 81673799 and 81703915.
Conflict of Interest
The authors declare that the research was conducted in the absence of any commercial or financial relationships that could be construed as a potential conflict of interest.
Supplementary Material
The Supplementary Material for this article can be found online at: https://www.por-journal.com/articles/10.3389/pore.2021.609083/full#supplementary-material.
Abbreviations
HER2, human epidermal growth factor receptor 2; TCGA, the Cancer Genome Atlas; lncRNA, long non-coding RNA; ceRNA, competing endogenous RNA; mRNA, messenger RNA; ROC, receiver operating characteristic; AUC, area under the curve; miRNA, microRNA; GEPIA, Gene Expression Profiling Interactive Analysis; GO, gene ontology; KEGG, Kyoto Encyclopedia of Genes and Genomes; STRING, search tool for the retrieval of interacting genes; PPI, protein-protein interaction; WGCNA, weighted gene co-expression network analysis; AMPK, Adenosine 5′-monophosphate (AMP)-activated protein kinase
References
1. Wolff, AC, Hammond, MEH, Hicks, DG, Dowsett, M, McShane, LM, Allison, KH, et al. Recommendations for human epidermal growth factor receptor 2 testing in breast cancer: American Society of Clinical Oncology/College of American Pathologists clinical practice guideline update. Arch Pathol Lab Med (2013) 138(2):241–56. doi:10.5858/arpa.2013-0953-sa
2. Sun, Y, Li, W, Li, A-J, Su, H, Yue, J, and Yu, J. Increased systemic immune-inflammation index independently predicts poor survival for hormone receptor-negative, HER2-positive breast cancer patients. Cmar (2019) 11:3153. doi:10.2147/cmar.s190335
3. Badache, A, and Gonçalves, A. The ErbB2 signaling network as a target for breast cancer therapy. J Mammary Gland Biol Neoplasia (2006) 11(1):13–25. doi:10.1007/s10911-006-9009-1
4. Parise, CA, and Caggiano, V. Breast cancer survival defined by the ER/PR/HER2 subtypes and a surrogate classification according to tumor grade and immunohistochemical biomarkers. J Cancer Epidemiol (2014) 2014:1–11. doi:10.1155/2014/469251
5. Cerk, S, Schwarzenbacher, D, Adiprasito, J, Stotz, M, Hutterer, G, Gerger, A, et al. Current status of long non-coding RNAs in human breast cancer. Ijms (2016) 17(9):1485. doi:10.3390/ijms17091485
6. Guttman, M, Amit, I, Garber, M, French, C, Lin, MF, Feldser, D, et al. Chromatin signature reveals over a thousand highly conserved large non-coding RNAs in mammals. Nature (2009) 458(7235):223. doi:10.1038/nature07672
7. Hung, T, and Chang, HY. Long noncoding RNA in genome regulation. RNA Biol (2010) 7(5):582–5. doi:10.4161/rna.7.5.13216
8. Fatica, A, and Bozzoni, I. Long non-coding RNAs: new players in cell differentiation and development. Nat Rev Genet (2014) 15(1):7. doi:10.1038/nrg3606
9. Gutschner, T, and Diederichs, S. The hallmarks of cancer. RNA Biol (2012) 9(6):703–19. doi:10.4161/rna.20481
10. Huarte, M. The emerging role of lncRNAs in cancer. Nat Med (2015) 21(11):1253. doi:10.1038/nm.3981
11. Lipovich, L, Johnson, R, and Lin, C-Y. MacroRNA underdogs in a microRNA world: evolutionary, regulatory, and biomedical significance of mammalian long non-protein-coding RNA. Biochim Biophys Acta (Bba) - Gene Regul Mech (2010) 1799(9):597–615. doi:10.1016/j.bbagrm.2010.10.001
12. Wang, Z, Jin, Y, Ren, H, Ma, X, Wang, B, and Wang, Y. Downregulation of the long non-coding RNA TUSC7 promotes NSCLC cell proliferation and correlates with poor prognosis. Am J Transl Res (2016) 8(2):680
13. Zhao, J, Liu, Y, Huang, G, Cui, P, Zhang, W, and Zhang, Y. Long non-coding RNAs in gastric cancer: versatile mechanisms and potential for clinical translation. Am J Cancer Res (2015) 5(3):907–27.
14. Bao, X, Anastasov, N, Wang, Y, and Rosemann, M. A novel epigenetic signature for overall survival prediction in patients with breast cancer. J Transl Med (2019) 17(1):380. doi:10.1186/s12967-019-2126-6
15. Zhang, D, Duan, Y, Cun, J, and Yang, Q. Identification of prognostic alternative splicing signature in breast carcinoma. Front Genet (2019) 10:278. doi:10.3389/fgene.2019.00278
16. Leung, F, Diamandis, EP, and Kulasingam, V. Advances in clinical chemistry, 66. Elsevier (2014), Ovarian cancer biomarkers: current state and future implications from high-throughput technologies. p. 25–77.
17. Gao, C, Li, H, Zhuang, J, Zhang, H, Wang, K, Yang, J, et al. The construction and analysis of ceRNA networks in invasive breast cancer: a study based on the Cancer Genome Atlas. Cancer Manag Res (2019) 11:1. doi:10.2147/CMAR.S182521
18. Ritchie, ME, Phipson, B, Wu, D, Hu, Y, Law, CW, Shi, W, et al. Limma powers differential expression analyses for RNA-sequencing and microarray studies. Nucleic Acids Res (2015) 43(7):e47. doi:10.1093/nar/gkv007
19. Gentleman, R, and Ihaka, R. R: a language and environment for statistical computing. Computing (2011) 1:12–21.
20. Zhang, X, Gao, C, Liu, L, Zhou, C, Liu, C, Li, J, et al. DNA methylation‐based diagnostic and prognostic biomarkers of nonsmoking lung adenocarcinoma patients. J Cell Biochem (2019). 120(8):13520–30. doi:10.1002/jcb.28627
21. Liu, J, Li, H, Sun, L, Wang, Z, Xing, C, and Yuan, Y. Aberrantly methylated-differentially expressed genes and pathways in colorectal cancer. Cancer Cel Int (2017) 17(1):75. doi:10.1186/s12935-017-0444-4
22. Bader, GD, and Hogue, CW. An automated method for finding molecular complexes in large protein interaction networks. BMC bioinformatics (2003) 4:2. doi:10.1186/1471-2105-4-2
23. Sacrdoni, G, Petterlini, M, and Laudanna, C. Analyzing biological network parameters with CentiScaPe. Bioinformatics (Oxford, England) (2009) 25(21):2857–9. 10.1093/bioinformatics/btp517
24. Lin, H, and Zelterman, D. Modeling survival data: extending the Cox model. Technometrics (2000) 44(1):85–6.
25. Ishwaran, H, Kogalur, UB, and Kogalur, MUB. Package ‘randomForestSRC’. Hemant Ishwaran and Udaya B. Kogalur (2019). Available at: https://kogalur.github.io/randomForestSRC/.
26. Heagerty, PJ, and Saha, P. time-dependent ROC curve estimation from censored survival data. Biometrics, (2013).66(4):999-1011. 10.1111/j.1541-0420.2009.01375.x
27. Langfelder, P, and Horvath, S. Fast R functions for robust correlations and hierarchical clustering. J Stat Softw (2012) 46
28. Wang, J-D, Zhou, H-S, Tu, X-X, He, Y, Liu, Q-F, Liu, Q, et al. Prediction of competing endogenous RNA coexpression network as prognostic markers in AML. Aging (2019) 11(10):3333. doi:10.18632/aging.101985
29. Xu, P, Yang, J, Liu, J, Yang, X, Liao, J, Yuan, F, et al. Identification of glioblastoma gene prognosis modules based on weighted gene co-expression network analysis. BMC Med genomics (2018) 11(1):96. doi:10.1186/s12920-018-0407-1
30. Tang, Z, Li, C, Kang, B, Gao, G, Li, C, and Zhang, Z. GEPIA: a web server for cancer and normal gene expression profiling and interactive analyses. Nucleic Acids Res (2017) 45(W1):W98–W102. doi:10.1093/nar/gkx247
31. Hu, X, Stern, HM, Ge, L, O'Brien, C, Haydu, L, Honchell, CD, et al. Genetic alterations and oncogenic pathways associated with breast cancer subtypes. Mol Cancer Res (2009) 7(4):511–22. doi:10.1158/1541-7786.mcr-08-0107
32. Banerji, S, Cibulskis, K, Rangel-Escareno, C, Brown, KK, Carter, SL, Frederick, AM, et al. Sequence analysis of mutations and translocations across breast cancer subtypes. Nature (2012) 486(7403):405. doi:10.1038/nature11154
33. Shah, SP, Roth, A, Goya, R, Oloumi, A, Ha, G, Zhao, Y, et al. The clonal and mutational evolution spectrum of primary triple-negative breast cancers. Nature (2012) 486(7403):395. doi:10.1038/nature10933
34. Stephens, PJ, Tarpey, PS, Tarpey, PS, Davies, H, Van Loo, P, Greenman, C, et al. The landscape of cancer genes and mutational processes in breast cancer. Nature (2012) 486(7403):400. doi:10.1038/nature11017
35. Győrffy, B, Pongor, L, Bottai, G, Li, X, Budczies, J, Szabó, A, et al. An integrative bioinformatics approach reveals coding and non-coding gene variants associated with gene expression profiles and outcome in breast cancer molecular subtypes. Br J Cancer (2018) 118(8):1107. doi:10.1038/s41416-018-0030-0
36. Zeng, P, Sun, S, Li, R, Xiao, Z-X, and Chen, H. HER2 upregulates ATF4 to promote cell migration via activation of ZEB1 and downregulation of E-cadherin. Ijms (2019) 20(9):2223. doi:10.3390/ijms20092223
37. Greenshields, AL, Fernando, W, and Hoskin, DW. The anti-malarial drug artesunate causes cell cycle arrest and apoptosis of triple-negative MDA-MB-468 and HER2-enriched SK-BR-3 breast cancer cells. Exp Mol Pathol (2019) 107:10–22. doi:10.1016/j.yexmp.2019.01.006
38. Garcia, D, and Shaw, RJ. AMPK: mechanisms of cellular energy sensing and restoration of metabolic balance. Mol Cel (2017) 66(6):789–800. doi:10.1016/j.molcel.2017.05.032
Keywords: human epidermal growth factor receptor 2-positive breast cancer, random forests, prognosis, long non-coding RNA, competing endogenous RNA
Citation: Zhang X, Zhang H, Li J, Ma X, He Z, Liu C, Gao C, Li H, Wang X and Wu J (2021) 6-lncRNA Assessment Model for Monitoring and Prognosis of HER2-Positive Breast Cancer: Based on Transcriptome Data. Pathol. Oncol. Res. 27:609083. doi: 10.3389/pore.2021.609083
Received: 22 September 2020; Accepted: 01 February 2021;
Published: 13 April 2021.
Edited by:
Andrea Ladányi, National Institute of Oncology, HungaryCopyright © 2021 Zhang, Zhang, Li, Ma, He, Liu, Gao, Li, Wang and Wu. This is an open-access article distributed under the terms of the Creative Commons Attribution License (CC BY). The use, distribution or reproduction in other forums is permitted, provided the original author(s) and the copyright owner(s) are credited and that the original publication in this journal is cited, in accordance with accepted academic practice. No use, distribution or reproduction is permitted which does not comply with these terms.
*Correspondence: Jibiao Wu, d3VqaWJpYW8xOTYzQDE2My5jb20=