- 1Department of Radiology, The Second People’s Hospital of Hunan Province/Brain Hospital of Hunan Province, Changsha, China
- 2Department of Radiology, Changsha Central Hospital, Changsha, China
- 3Department of Critical Care Medicine, National Clinical Research Center for Geriatric Disorders, Xiangya Hospital, Central South University, Changsha, China
Hepatocellular carcinoma (HCC), a high mortality malignancy, has become a worldwide public health concern. Acquired resistance to the multikinase inhibitor sorafenib challenges its clinical efficacy and the survival benefits it provides to patients with advanced HCC. This study aimed to identify critical genes and pathways associated with sorafenib resistance in HCC using integrated bioinformatics analysis. Differentially expressed genes (DEGs) were identified using four HCC gene expression profiles (including 34 sorafenib-resistant and 29 sorafenib-sensitive samples) based on the robust rank aggregation method and R software. Gene ontology (GO) functional annotation and Kyoto Encyclopedia of Genes and Genomes (KEGG) pathway analysis were performed using the Database for Annotation, Visualization and Integrated Discovery (DAVID) online tool. A protein–protein interaction (PPI) network was constructed using the Search Tool for the Retrieval of Interacting Genes (STRING), and small molecules reversing sorafenib resistance were searched for using the connectivity map (CMAP) database. Pearson correlation and survival analyses of hub genes were performed using cBioPortal and Gene Expression Profiling and Interactive Analysis (GEPIA). Finally, the expression levels of hub genes in sorafenib-resistant HCC cells were verified using quantitative polymerase chain reaction (q-PCR). A total of 165 integrated DEGs (66 upregulated and 99 downregulated in sorafenib resistant samples compared sorafenib sensitive ones) primarily enriched in negative regulation of endopeptidase activity, extracellular exosome, and protease binding were identified. Some pathways were commonly shared between the integrated DEGs. Seven promising therapeutic agents and 13 hub genes were identified. These findings provide a strategy and theoretical basis for overcoming sorafenib resistance in HCC patients.
Introduction
Hepatocellular carcinoma (HCC) is the most prevalent primary liver cancer, ranking sixth among the most common malignant tumors worldwide [1]. With approximately 8,41,000 new cases and 7,80,000 deaths in 2018, the incidence and mortality rates of HCC are increasing [2]. Variations in the incidence rate for HCC globally are attributed to differences in risk factors. In general, the incidence for HCC in developing countries is higher than that in developed countries. The majority of HCC patients (80%) are from East Asia and sub-Saharan Africa, where the major risk factors are the prevalence of chronic Hepatitis B virus and aflatoxin B1 [3], whereas, presence of Hepatitis C virus and alcohol overuse are the primary pathogenic factors for HCC in Europe, America and Japan [4].
Over the past decades, noticeable progress has been achieved towards the prevention, diagnosis, and treatment of HCC. Surgery, locoregional treatment, and systemic therapies have proven effective against HCC. Appropriate treatments for HCC depend on multiple factors, such as the tumor stage of HCC, performance status of patients, etc. [5]. Curative treatments including radiofrequency ablation, liver resection, and liver transplantation are more suitable for treating early or very early-stage cancer [5]. However, most patients with HCC present at an intermediate or advanced stage at the time of diagnosis. Eliminating the possibility of curative treatment, systemic treatment or palliative treatment is essential [4]. An in-depth evaluation of the pathogenesis of HCC has inferred that hepatocellular carcinogenesis and its progression are complex multi-step processes involving persistent genetic mutations. Therefore, research into and application of molecular targeted drugs for dysregulated genes associated with HCC are of great importance.
Since 2007, sorafenib, an effective first-line systemic therapy, has been approved for clinical application in patients with advanced-stage HCC and provides consistent survival benefits [6]. It is an orally active multiple-target tyrosine kinase inhibitor (TKI) that suppresses tumor angiogenesis and progression through various molecular targets, such as RAF/MAPK/ERK pathway, vascular endothelial growth factor receptor tyrosine kinases, platelet-derived growth factor receptor, fibroblast growth factor receptor, myeloid cell leukemia-1, FMS-like tyrosine kinase-3, receptor tyrosine kinase, and shugoshin-like 1 [7–11]. Robust evidence and clinical experiments inferred that sorafenib provides a cornerstone treatment by substantially extending the median overall survival of advanced-stage HCC patients [12,13]. With the approval of several immune-checkpoint inhibitors such as lenvatinib, regorafenib, cabozantinib, ramucirumab [14], targeted therapy provides a new strategy for HCC treatment.
However, acquired drug resistance becomes a vexing problem within 6 months of drug application. Studying the sorafenib resistance mechanism may aid in overcoming drug resistance and improve the targeted drug efficacy. Apart from epigenetic modifications, transport processes, or other mechanisms, gene disorders and signaling pathways are some common phenomena causing sorafenib resistance. Studies to identify critical genes and pathways might aid in reversing sorafenib resistance.
With the rapid development in sequencing technology, huge volumes of gene expression profiling data related to cancer were generated and uploaded to the Gene Expression Omnibus (GEO) database. Meanwhile, various analytical methods were applied for accurate identification of critically differentially expressed genes and pathways involved in cancer. As described previously [15], the robust rank aggregation (RRA) algorithm was used to analyze multiple gene lists based on different data platforms [16]. The statistical model of RRA assumes that all the genes in each dataset are randomly arranged. As a gene ranks higher in all datasets, its p-value gets lowered, which provides it with a greater possibility of getting converted to a differentially expressed gene (DEG).
The present study included 63 samples (34 sorafenib-resistant and 29 sorafenib-sensitive samples) from four datasets. R software was used to identify the DEGs in each dataset and RRA method was applied to obtain integrated DEGs. Subsequently, gene ontology (GO) term enrichment analyses and Kyoto Encyclopedia of Genes and Genomes (KEGG) pathway analyses of DEGs were performed on Database for Annotation, Visualization and Integrated Discovery online tool 6.8 (https://david.ncifcrf.gov/). The connectivity map (CMAP) database was used to search for small-molecule candidates which might reverse sorafenib resistance. Thereafter, the hub genes were inferred from protein–protein interaction (PPI) network of integrated DEGs and survival analysis was performed by generating overall survival curves in the gene expression profiling and interactive analysis (GEPIA). Finally, the expression levels of the top hub genes were verified via quantitative polymerase chain reaction in sorafenib-resistant hepatocellular carcinoma cells.
Materials and Methods
Microarray Data
Gene expression profiles of GSE62813, GSE73571, GSE151412, and GSE140202, were downloaded from the National Center for Biotechnology Information (NCBI) Gene Expression Omnibus (GEO) database (https://www.ncbi.nlm.nih.gov/geo/). GSE62813 and GSE73571 dataset are based on the platform of GPL6244 [HuGene-1_0-st] Affymetrix Human Gene 1.0 ST Array [transcript (gene) version], while the platforms of GSE151412 and GSE140202 dataset are GPL15520 Illumina MiSeq (Homo sapiens) and GPL20795 HiSeq X Ten (Homo sapiens), respectively (Table 1). Gene probe IDs were converted into international standard gene name using A Perl language command, and the gene expression data was normalized by the normalization Between Arrays function in the limma R package (http://www.bioconductor.org/) [17].
Screening for DEGs
The DEGs of each dataset were identified using limma R package V3.5.2 in R software with the cut-off criterion that adjusted p- value < 0.05 and |log2FC| > 1. Four gene lists, arranged according to log2FC value, were merged using the RobustRankAggreg R package (https://cran.rstudio.com/bin/windows/contrib/3.5/RobustRankAggreg_1.1.zip).[16] The integrated DEGs (upregulated and downregulated genes in sorafenib resistant samples compared sorafenib sensitive ones) were selected based on the cut-off criterion that p- value < 0.05 and |log2FC| > 0.5.
Functional and Pathway Enrichment Analysis
As described previously [15], the Database for Annotation, Visualization and Integrated Discovery (DAVID, http://david.ncifcrf.gov) (version 6.8), a public online platform, is available for analyzing large-scale lists of genes or proteins and provides their biological information or characteristics for users. GO and KEGG pathway enrichment analysis were performed using the DAVID online tool. p < 0.05 was considered statistically significant.
PPI Network Analysis
The Search Tool for the Retrieval of Interacting Genes (STRING) (https://string-db.org/) is commonly used to display the direct and indirect relationship of multiple proteins through forming PPI network [18]. The PPI network of integrated DEGs was exported and re-displayed by Cytoscape software (3.7.1), and the significant module was selected through the plug-in Molecular Complex Detection (MCODE) [19] app in Cytoscape software with the criterion that degree cut-off ≥ 2, node score cut-off ≥ 0.2, K-core ≥ 2, and max depth D = 100.
Connectivity Map Analysis
The Connectivity Map (CMAP, http://www.broad.mit.edu/cmap/), a pattern-matching tool, was created to make disease–gene–drug connections [20]. By using the tool, users not only explore the mechanism of drug action, but also discover potential drugs for diseases through comparing its signature to the database to detect similarities [21]. The lists of upregulated and downregulated DEGs obtained from this study were submitted to CMAP to compare with the reference dataset. According to the enrichment of DEGs in the reference gene expression profile, a correlation score (−100∼100) was obtained; a positive number indicates that gene expression trend of the DEGs is similar to the reference gene expression profile. However, a negative number indicates that gene expression trend of the DEGs may be opposite to the reference gene expression profile.
Pearson Correlation and Survival Analyses of Hub Genes
The hub genes were determined with degree ≥12. Pearson correlation analyses of hub genes were performed using the data of mRNA expression z-scores relative to all samples (log RNA Seq V2 RSEM) from the cBioPortal database for Liver Hepatocellular Carcinoma (TCGA, PanCancer Atlas, the genomic profiles including mutations, structural variant, putative copy-number alterations from GISTIC, mRNA expression z-scores relative to diploid samples). In addition, overall survival analysis of the top 13 hub genes was also accomplished on cBioportal. The tumor/normal differential expression analysis and prognostic value of hub genes were analyzed on GEPIA (http://gepia.cancer-pku.cn/), which is an interactive web application for gene expression analysis based on TCGA and GTEx data [22].
Detection of the Expression Levels of Hub Genes in Sorafenib-Resistant Hepatocellular Carcinoma Cells
Sorafenib-resistant HCC cell line Huh7-SOR was generated by treating cells with a series of increasing concentrations ranging from 1 to 10 µM of sorafenib, and the concentration of sorafenib increased by 0.25 µM per cycle. Resistance indexes to sorafenib in Huh7 and Huh7-SOR cells were detected using CCK-8 assay [23]. Huh7-SOR cells were continuously cultured in the presence of 1 μM of sorafenib. RNA isolation and quantitative polymerase chain reaction (qPCR) analysis were conducted as described previously [24], and GAPDH was used as an endogenous expression control for mRNA. The primers of top 13 hub genes were designed and synthesized by Shanghai Bioengineering Co., Ltd. (Table 2).
Results
Identification of Differentially Expressed Genes
In present study, 81 DEGs (20 upregulated and 61 downregulated), 91 DEGs (51 upregulated and 40 downregulated), 49 DEGs (27 upregulated and 22 downregulated), 402 DEGs (239 upregulated and 163 downregulated) were determined from four HCC expression profiles related to sorafenib resistance (Table 3 and Figure 1). After integrated analysis, a total of 165 DEGs (66 upregulated and 99 downregulated) were selected (Supplementary Table S1).
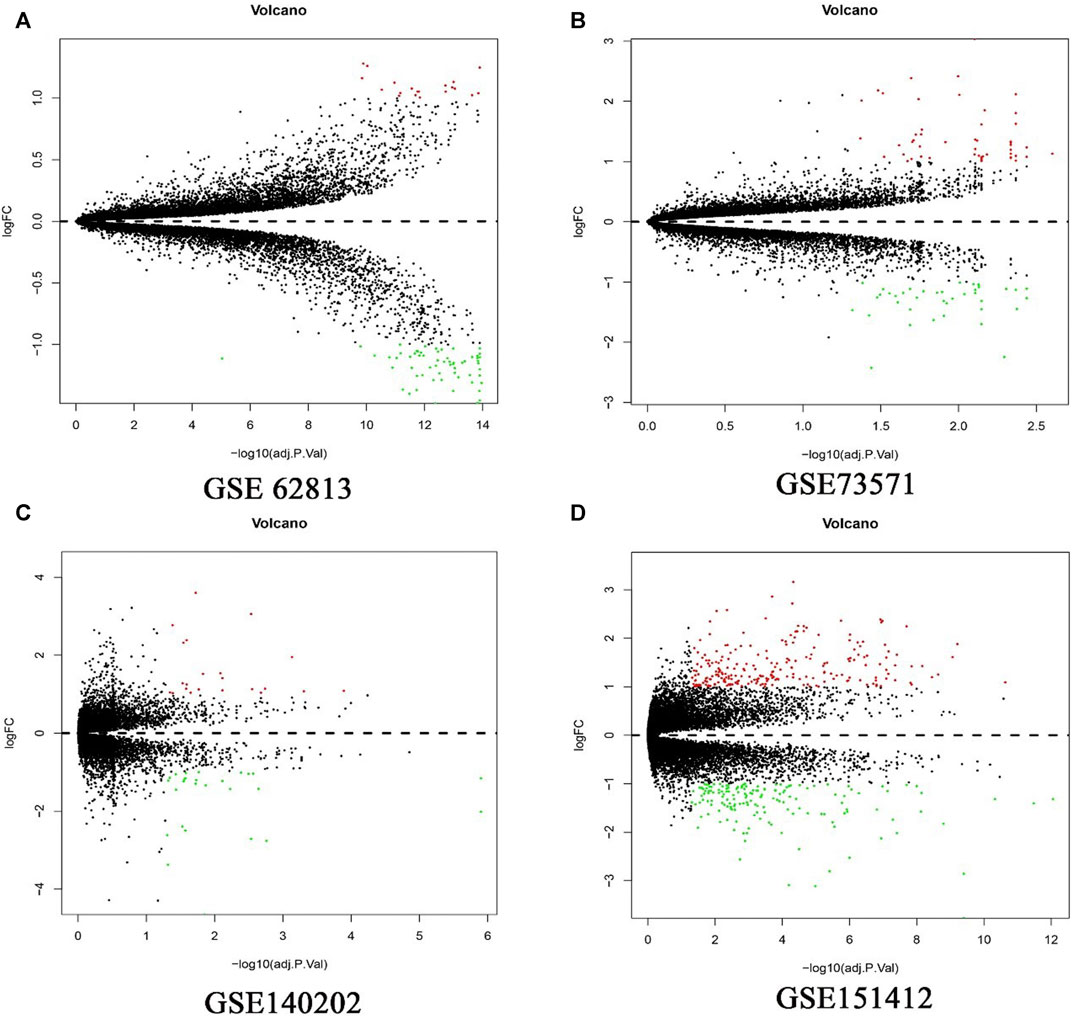
FIGURE 1. Differential expression genes between the two groups of samples in each dataset. (A) GSE62813, (B) GSE73571, (C) GSE140202, (D) GSE151412. The red dots represent the upregulated genes and the green dots represent the downregulated genes; the black spots represent genes with no significant difference in expression level.
Functional and Pathway Enrichment Analysis
In order to systematically and comprehensively assess biological information of the integrated DEGs, GO enrichment analysis and KEGG pathway enrichment analysis were conducted using online database DAVID 6.8. GO enrichment analysis is made up of biological process group (BP), cellular component group (CC), and molecular function group (MF). As shown in Figure 2 and Supplementary Table S2, the 165 DEGs were mainly enriched in 95 biological process terms, 22 cell component terms, 32 molecular function terms, and 9 KEGG pathway terms. Moreover, the DEGs were participated in multiple biological process, especially focusing on negative regulation of endopeptidase activity (11 genes), platelet degranulation (9 genes), acute-phase response (6 genes), response to nutrient (7 genes), and cellular response to tumor necrosis factor (8 gene). For the CC, the DEGs were particularly enriched in extracellular exosome (63 genes), extracellular region (45 genes), extracellular space (40 genes), extracellular matrix (12 genes), and platelet alpha granule lumen (6 genes). For the MF, the DEGs were associated with protease binding (9 genes), integrin binding (7 genes), heparin binding (8 genes), serine-type endopeptidase inhibitor activity (6 genes), and transporter activity (8 genes).
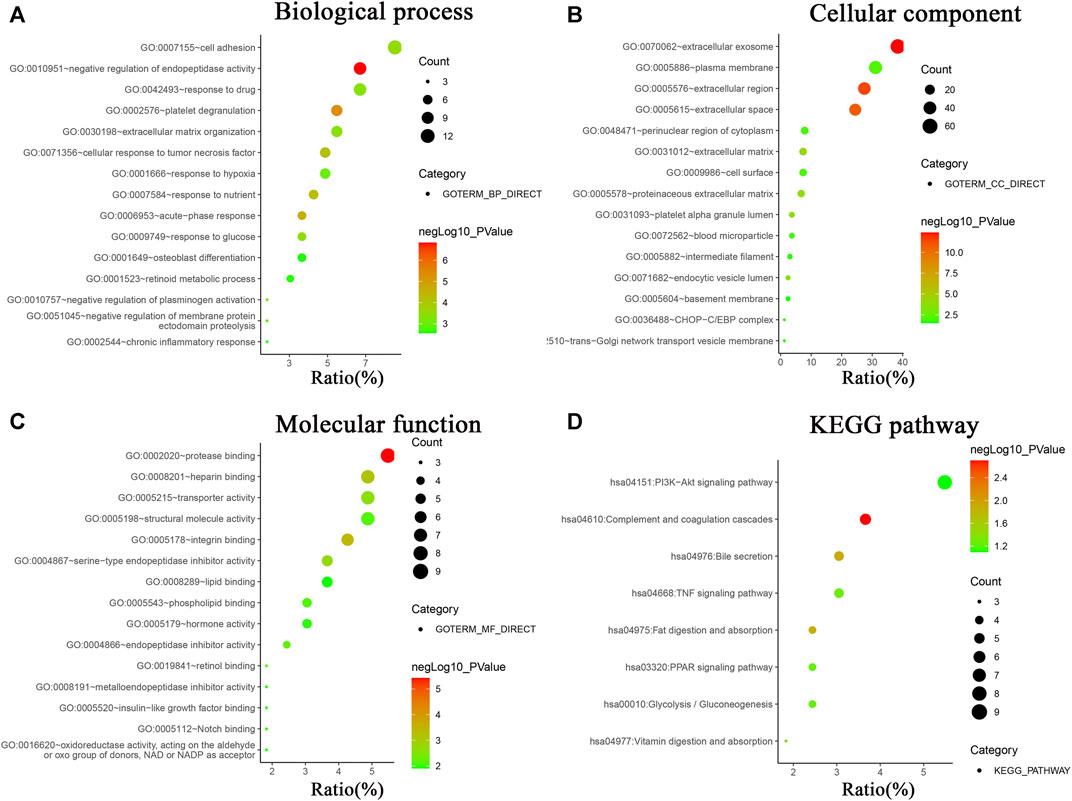
FIGURE 2. GO analysis and KEGG pathway enrichment analysis of DEGs using online database DAVID. (A) Biological processes of GO analysis, (B) cellular components of GO analysis, (C) molecular functions of GO analysis, (D) KEGG pathway enrichment analysis.
KEGG pathway enrichment analysis revealed the DEGs were mainly involved in PI3K-Akt signaling pathway (9 genes), complement and coagulation cascades (6 genes), bile secretion (5 genes), TNF signaling pathway (5 genes), fat digestion and absorption (4 genes), PPAR signaling pathway (4 genes), Glycolysis/Gluconeogenesis (4 genes), and vitamin digestion and absorption (3 genes).
Specific Drug Screening From CMAP Database to Relieve Sorafenib Resistance
In order to discover small molecules that have the potential to reverse the resistance of sorafenib, the 165 DEGs were submitted to CMAP for analysis with the cut-off criterion that the number of repeat experiment times≥ 4, the mean ≤−0.4 and p-value < 0.05. Seven small-molecule candidates were identified, including pentetrazol, hesperidin, digoxin, mebeverine, cinnarizine, sulfadiazine, sulfametoxydiazine (Table 4).

TABLE 4. Small molecule agents with potential abilities to overcome sorafenib resistance of HCC were identified by CMAP database.
PPI Network Analysis to Identify Hub Genes
After analysis, 36 genes were filtered out, and the remaining 129 genes (53 upregulated and 76 downregulated) formed the PPI network, which contained 129 nodes and 401 edges (Supplementary Figure S1, Supplementary Tables S3, S4). Using MCODE for automatic screening, 13 central node genes with 12° (i.e., each node has more than 12 connections/interactions) or more were recognized as hub genes. The top 13 hub genes were as follows: SERPINA1, IGFBP1, KNG1, TIMP1, APOA1, SPP1, IGFBP3, FBN1, VCAN, MATN3, STC2, SERPINC1, and APOB(Table 5 and Figure 3A). The expression levels of the top 13 hub genes from 4 datasets were showed in a heatmap. As shown in Figure 3B, IGFBP1 and VNAN were downregulated in sorafenib resistant samples of 4 datasets, TIMP1, SPP1, IGFBP3, MANT3, and APOB were downregulated in sorafenib resistant samples of three datasets. However, KNG1, APOA1, and SERPINC1 were upregulated in sorafenib resistant samples of three datasets.
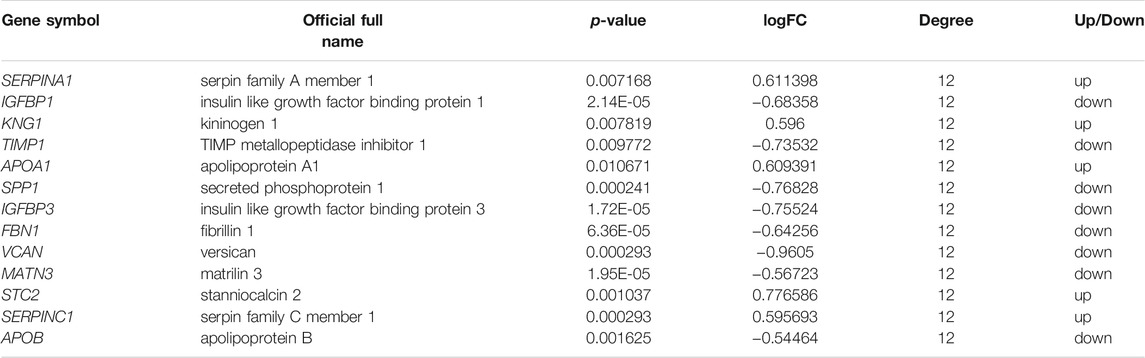
TABLE 5. The top 13 most degree values hub genes between sorafenib sensitive and acquired sorafenib resistant HCC cells.
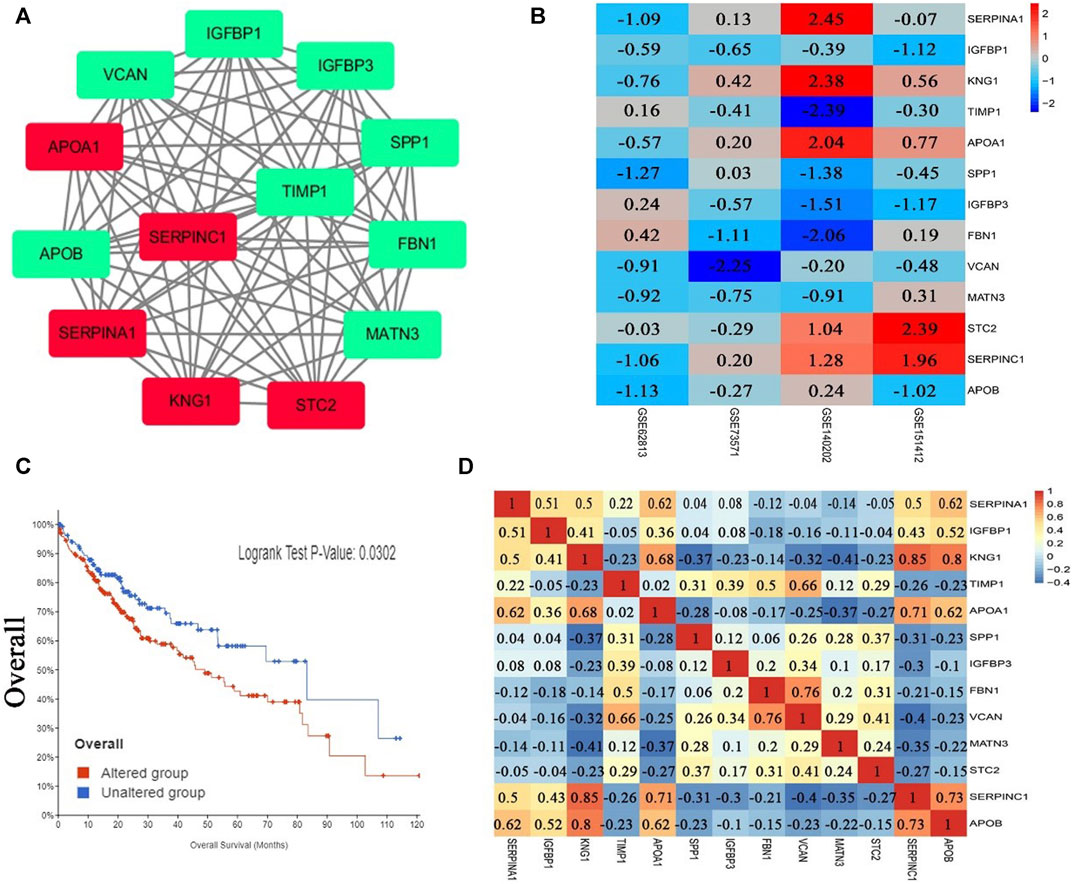
FIGURE 3. PPI network, Pearson correlation and overall survival analysis of top 13 hub genes. (A) The most significant module of hub genes from PPI network (the red represents log FC > 0, the green represents log FC < 0). (B) Heatmap of the expression levels of top 13 genes in 4 datasets. (C) Overall survival analysis of top 13 hub genes (altered group = 227, unaltered group = 144). (D) Pearson correlation analysis of top 13 hub genes.
Pearson Correlation and Overall Survival Analysis of Hub Genes
The results of Pearson correlation analysis indicated that strong positive correlations were observed in the following hub genes (Figure 3D): SERPINA1 with APOA1, SERPINA1 with APOB, KNG1 with APOA1, KNG1 with SERPINC1, KNG1 with APOB, TIMP1 with VCAN, APOA1 with SERPINC1, APOA1 with APOB, FBN1 with VCAN, and SERPINC1 with APOB. Moderate positive correlations were observed in the following hub genes: SERPINA1 with IGFBP1, SERPINA1 with KNG1, SERPINA1 with SERPINC1, IGFBP1 with KNG1, IGFBP1 with SERPINC1, IGFBP1 with APOB, TIMP1 with FBN1, and STC2 with VCAN. However, moderate negative correlations were observed for KNG1 with MATN3, and FBN1 with SERPINC1. In addition, significant difference was observed between groups of cases with and without alteration(s) in top 13 hub genes (logrank test p-value, 0.0302) from the overall survival analysis generated from the cBioPortal database for Liver Hepatocellular Carcinoma (TCGA, PanCancer Atlas, the genomic profiles including mutations, structural variant, putative copy-number alterations from GISTIC, mRNA expression z-scores relative to diploid samples) (Figure 3C).
The Expression Levels of Hub Genes in Liver Hepatocellular Carcinoma Patients and its Potential Prognostic Efficacy
369 liver cancer and 160 normal tissues from TCGA/GTEx datasets were included tumor/normal differential expression analysis using the GEPIA. Compared with normal tissues, the expression levels of TIMP1 and SPP1 in liver hepatocellular carcinoma tissues (LIHC) were significantly upregulated, while IGFBP3 was significantly downregulated (Figure 4). Prognostic value of single hub gene was assessed through survival plots of the overall survival generated from the GEPIA. As shown in Figures 5A–F, HCC patients with STC2, MATN3, SPP1, IGFBP3, and VCAN up-regulation showed worse overall survival. Nonetheless, patients with KNG1 down-regulation showed worse overall survival. HCC patients with SERPINC1 down-regulation showed worse disease free survival (Figure 5G).
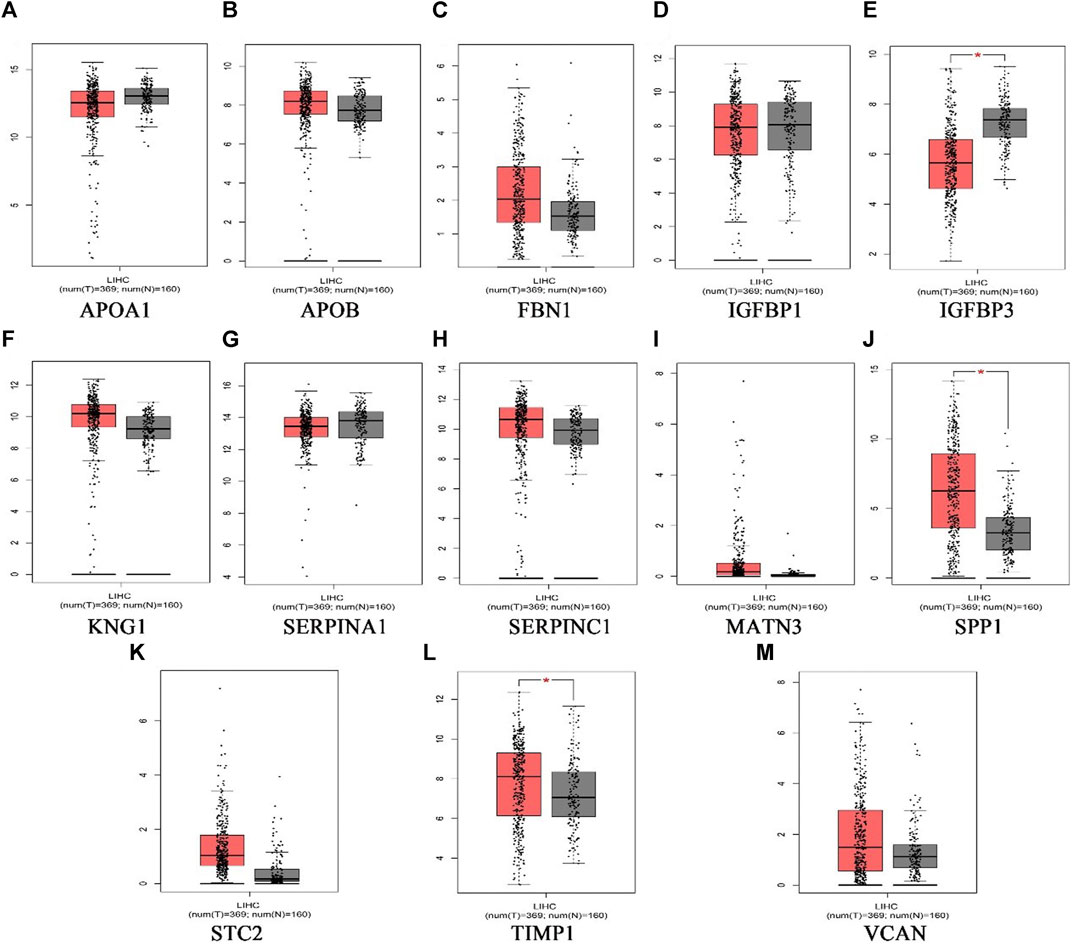
FIGURE 4. The expression level of hub genes in liver hepatocellular carcinoma patients. (A)APOA1, (B)APOB, (C)FBN1, (D)IGFBP1, (E)IGFBP3, (F)KNG1, (G) SERPINA1, (H) SERPINC1, (I) MATN3, (J) SPP1, (K) STC2, (L) TIMP1, (M) VCAN. p < 0.05 (*). (the red represents tumor group, the grey represents normal group).
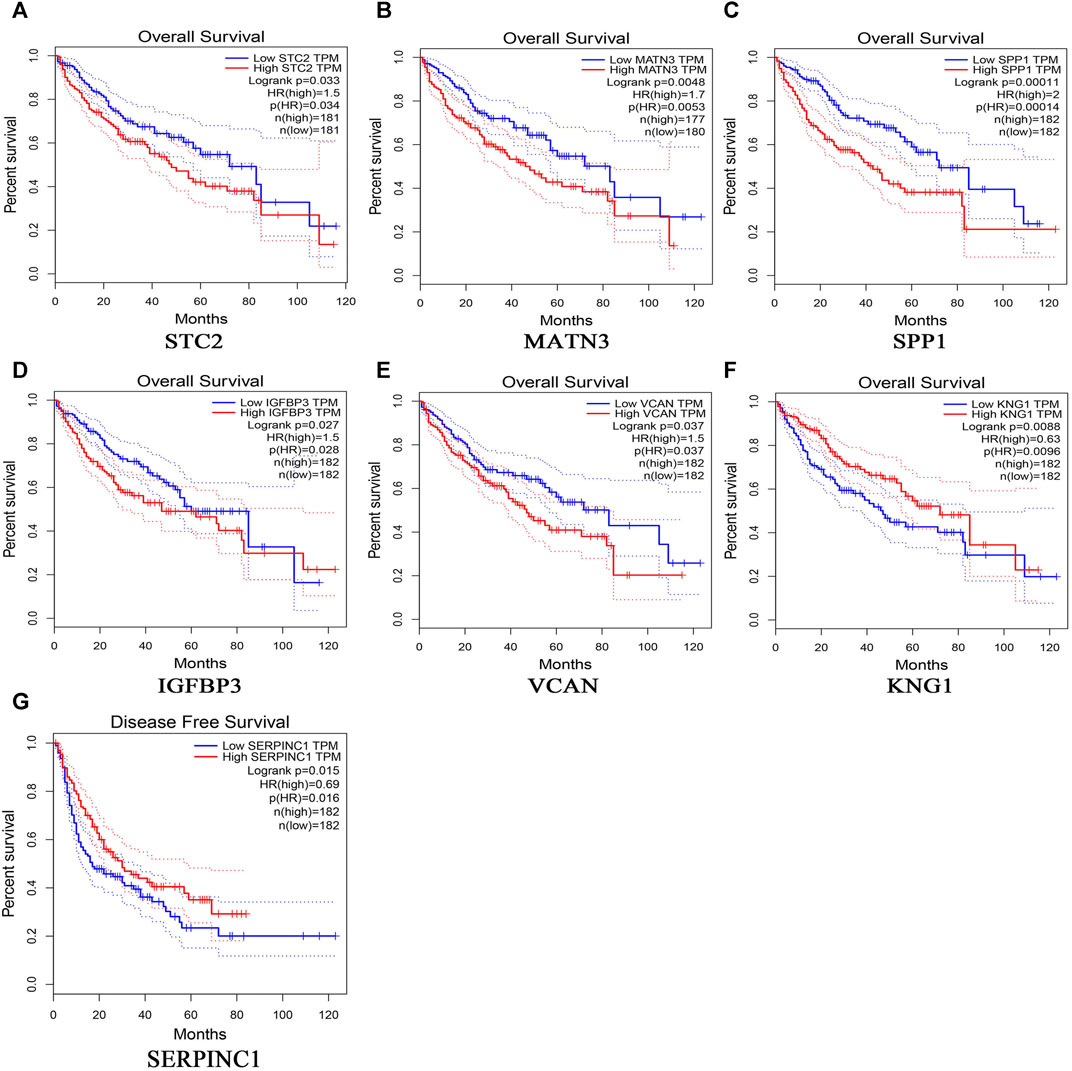
FIGURE 5. Overall survival analyses and disease free survival of single hub genes were generated from the GEPIA. Overall survival analyses: (A) STC2, (B) MATN3, (C) SPP1, (D) IGFBP3, (E) VCAN, (F) KNG1; Disease free survival, (G)SERPINC1.
The Expression Levels of Hub Genes in Sorafenib-Resistant Hepatocellular Carcinoma Cells
After cells were treated by sorafenib, the IC50 of sorafenib in Huh7-SOR cells was signifcantly higher than that of sorafenib in Huh7 cells (12.9 ± 1.4 μM vs. 7.1 ± 1.6 μM). The expression levels of 13 hub genes were measured by PCR in Huh7 and Huh7-SOR cells. Figure 6 and Supplementary Table S5 showed that six genes (SERPINA1, IGFBP1, SPP1, IGFBP3, VCAN, and APOB) were significantly increased or decreased between Huh7 and Huh7-SOR cells (p ≤ 0.05), whereas the remaining genes were moderately changed.
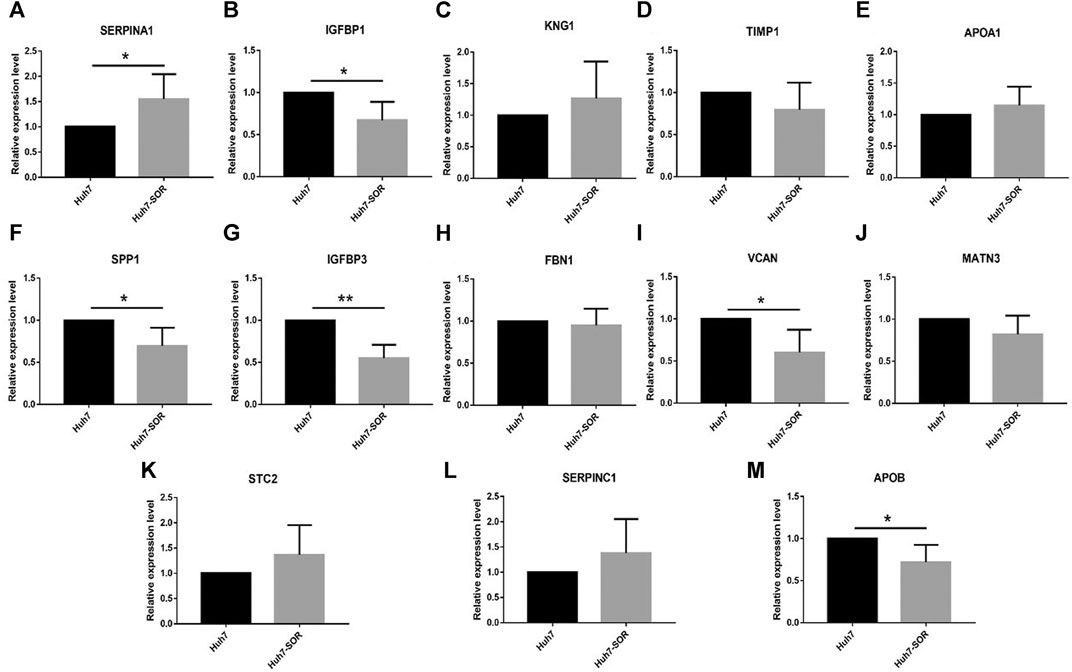
FIGURE 6. Detection of hub genes in sorafenib-resistant hepatocellular carcinoma cells by qRT-PCR. (A) SERPINA1, (B) IGFBP1, (C) KNG1, (D) TIMP1, (E) APOA1, (F) SPP1,(G) IGFBP3, (H) FBN1, (I) VCAN, (J) MATN3, (K) STC2, (L) SERPINC1, (M) APOB. p < 0.05 (*), p < 0.01 (**).
Discussion
In this study, we identified 165 sorafenib resistance-related DEGs in 63 samples (29 sorafenib-sensitive samples and 34 sorafenib-resistant) from four datasets using RRA statistical model. GO and KEGG pathway enrichment analysis revealed that DEGs were intricately involved in several biological processes and pathways that play a vital role in drug resistance in HCC. For the CC, the DEGs of present study were particularly enriched in extracellular. Extracellular matrix, an important component of tumor microenvironment, is a complex network surrounding the cells [25]. Exosomes, released by multiple cells types, contain various types of protein, lipids, nucleic acids (DNA, mRNA, and miRNA) and other molecules [26]. Emerging evidence suggests ECM and exosomes have great potential to support the development of drug resistance in HCC cells [27,28]. In additon, inhibition of the PI3K/Akt signaling pathway, TNF-α, PPARγ, and PPARδ reverses sorafenib resistance in HCC [29–35]. Elevated glycolysis was observed in sorafenib-resistant HCC cells, and downregulating the key glycolytic enzymes 6-phosphofructo-2-kinase/fructose-2,6-biphosphatase (encoded by PFKFB3) or pyruvate kinase muscle isozyme M2 re-sensitizes HCC cells to sorafenib [31,36,37].
Using the CMAP database, seven small-molecule candidates were identified as the most promising therapeutic agents which have the potential to reverse sorafenib resistance. Evidence shows that pentetrazol, hesperidin, and digoxin have the ability to change the sensitivity of tumor cells towards chemotherapeutic drugs. Targeting exosome biogenesis and release have important clinical significance in cancer treatment. Pentetrazol was identified as an activator of exosome biogenesis and/or secretion in prostate cancer cells [38]. Hesperidin sensitized Ramos cells to doxorubicin-induced apoptosis [39], and co-chemotherapy of doxorubicin and hesperidin showed the ability to overcome resistance of doxorubicin by suppressing P-glycoprotein expression in Michigan Cancer Foundation-7 (MCF-7)-resistant doxorubicin cells [40]. Co-administration of hypoxia inducible factor inhibitor and cytotoxic chemotherapy overcame the resistance of breast cancer stem cells to paclitaxel or gemcitabine [41]. There is no evidence showing that mebeverine, cinnarizine, sulfadiazine, and sulfamethoxydiazine were involved in regulating the sensitivity of chemotherapy resistance. Nevertheless, further in vivo and in vitro experiments are still needed to validate their activity in HCC sorafenib resistance and to explore additional potential molecular mechanisms.
The most significant module was selected from the PPI network and 13 hub genes were identified. After analysis in the GEPIA, TIMP1, SPP1, IGFBP3 were dysregulated in liver hepatocellular carcinoma patients. In addition, HCC patients with STC2, MATN3, SPP1, IGFBP3, VCAN, and KNG1 dysregulation showed worse overall survival. Moreover, SERPINA1, IGFBP1, SPP1, IGFBP3, VCAN, and APOB were significantly increased or decreased between Huh7 and Huh7-SOR cells in sorafenib-resistant HCC cells. SPP1 and STC2 have been found to be involved in chemotherapy resistance in HCC [42,43]. In another bioinformatics analysis, SERPINA1 was confirmed as the key gene from GSE109211 in sorafenib-resistant HCC cells [44].
The involvement of IGFBP1, TIMP1, SPP1, IGFBP3, and STC2 in mediating chemotherapy resistance in various cancers have been extensively studied. Through a genome-wide RNA interference screen, IGFBP1 was identified as one of the novel genes causing cellular resistance to neratinib [45]. Molecular mechanistic understanding has revealed that IGFBP1 is a key component of G protein-coupled estrogen receptor 1 (encoded by GPER1) which regulated the sensitivity of breast cancer cells to tamoxifen [46,47], and the resistance induced by RG7388 (MDM2 inhibitor) in glioblastoma [48]. Another study showed that reduction in insulin-like growth factor I (encoded by IGF-I) mediated part of the starvation-dependent differential stress resistance [49].
Elevated tumor tissue TIMP1 levels were significantly associated with a poor response to chemotherapy [50–52]. TIMP1 deficiency increases either tumor cell sensitivity to chemotherapy or TNF-α-induced apoptosis [53–55]. A change in TIMP1 expression could mediate resistance to gemcitabine in pancreatic cancer [56,57], to fulvestrant in MCF-7 human breast cancer cells [58], to platinum in epithelial ovarian cancer [59], to carboplatin in lung cancer [60], and to antiestrogen in breast cancer [61]. Furthermore, recombinant fusion protein linking TIMP1 to glycosylphosphatidylinositol anchor enhances tumor sensitivity to doxorubicin [62]. Moreover, another study using bioinformatics analysis revealed that TIMP1 has an association with lapatinib resistance [63].
Osteopontin (OPN), also known as SPP1, was identified as a candidate drug resistance biomarker in ovarian [64], pancreatic [65], and metastatic castration-resistant prostate cancers [66]. Upregulation of OPN expression contributed to cisplatin (DDP) resistance in cervical cancer cells [67,68], cetuximab-resistance in head and neck squamous cell carcinoma [69], second-generation epidermal growth factor receptor (EGFR)-TKI resistance in lung cancer [70], leukemic stem cell chemoresistance in acute myeloid leukemia [71], chemoresistance of HCC via autophagy [42], and chemotherapy resistance of mouse WAP-SVT/t breast cancer cells [72]. Disruption of OPN sensitized chemotherapy in experimental mammary tumors and metastatic breast cancer [73], and OPN knockdown reduced resistance to some drugs as manifested via increase in cell death [74]. In clinical trials, OPN expression was associated with the efficiency of neoadjuvant chemotherapy (NACT) in breast cancer treatment [75].
IGFBP3 is a specific biomarker associated with drug resistance of neuroblastoma cells to doxorubicin [76], gastric cancer cells to cisplatin [77], human epidermal growth factor receptor 2 (HER2) positive breast cancer to trastuzumab [78], lung adenocarcinoma harboring an EGFR mutation to afatinib [79], ovarian cancer to cisplatin [80], and non-small cell lung cancer (NSCLC) cells to docetaxel or gemcitabine [81]. Loss in IGFBP3 expression increased the response of the U251 human glioblastoma cell line to CA 125 [82], and enhanced antitumor action of DZ-50 in prostate cancer [83]. However, the downregulated expression of IGFBP-3 mediated the resistance to gefitinib in A431 squamous cancer cells [84], reduced the apoptosis of antiestrogen-resistant breast cancer cells to ICI 182,780 [85], increased the resistance to trastuzumab therapy in HER2 positive breast cancer [86], and reduced tumor sensitivity to molecular-targeted therapies in NSCLC [87]. In addition, growth factor sequestration by engineered IGFBP-3 enhanced the activity of EGFR inhibitors [88].
Upregulated STC2 was involved in drug resistance in HCC by increasing P-glycoprotein and B-cell lymphoma 2 protein expression levels [43], and it imparted resistance against EGFR tyrosine kinase inhibitors in lung cancer [89], resistance of cervical cancer cells to cisplatin [90], and induced oxaliplatin resistance in colorectal cancer cells [91]. Increased STC2 expression induced by anti-vascular endothelial growth factor antibody therapy was observed in colon cancer, whereas the role of STC2 is still unclear [92]. Patients who received first-line endocrine therapy with low-level expression of STC2 showed poor outcome [93].
Several studies have showed that SERPINA1, APOA1, VCAN, and APOB mediated the development of cancer chemotherapy resistance. The differential expression of SERPINA1 has been associated with platinum resistance in human epithelial ovarian cancer [94], CDDP resistance in gastric cancer (GC) [95], and tamoxifen resistance in breast cancer [96]. APOA1 was identified as a candidate drug resistance biomarker for ovarian cancer via 2D-gel proteomics [97]. APOA1 was found to be upregulated in the drug respondent group when compared to the drug-resistant group of HIV-1 patients treated with first-line antiretroviral therapy [98]. VCAN was upregulated in spiky (CRC cell line) that was resistant to growth inhibition of cetuximab, and VCAN staining strongly correlated with reduced survival in colorectal cancer [99]. Versican V1 overexpression in lymphoid cell lines enhanced their sensitivity to doxorubicin and gemcitabine [100]. Serum levels of APOB could predict responses to NACT and relapse-free survival in advanced breast cancers patients [101]. However, no research has shown that KNG1, FBN1, MATN3, and SERPINC1 play an important role in cancer chemotherapy resistance.
In accordance with our findings, the identification of genes and signaling pathways related to sorafenib resistance has also been found using bioinformatics methods in several studies[44,102,103]. Using the web tool GEO2R, GSE73571, and GSE109211 datasetwere analyzed. Sorafenib resistance-related DEGs (1,319 in total; 593 upregulated and 726 downregulated in sorafenib resistant samples compared sorafenib sensitive ones) were obtained, and the eight hub genes were identified from the GSE73571 dataset [103]. 164 sorafenib resistance-related DEGs (121 upregulated and 43 downregulated in sorafenib resistant samples compared sorafenib sensitive ones) were identified and nine hub genes were confirmed as key genes from the GSE109211 dataset [44]. In another study on the identification of microRNAs and transcription factors related to sorafenib resistance, GSE73571 was used and 827 significant DEGs were obtained using the “limma” package of R language [102].
Compared to the previous three studies, four GEO datasets comprising 61 samples were integrated and analyzed to obtain DEGs using the RRA method in present study. These four datasets included three HCC lines (Huh7, Hep3B, and HepG2) and hepatospheres generated from tumors (Huh7 cells) based on three different platforms (GPL6244, GPL20795, and GPL15520). Therefore, the hub genes identified in the present study are more reliable and comprehensive. Furthermore, we have also identified some small-molecule candidates that may overcome the resistance to sorafenib. However, the number of samples used in present study is relatively small, and the type of sample included is single (only HCC cell samples). The mechanisms and functions of DEGs in the resistance of sorafenib have not been further explained.
Conclusion
In summary, by conducting an integrated bioinformatics analysis using multiple datasets, we identified some hub genes and pathways involved in sorafenib resistance for HCC. In addition, seven small-molecule candidates that may overcome the resistance of sorafenib were identified. Our findings provide a deeper and more comprehensive understanding of the occurrence and development of sorafenib resistance, along with some strategies and directions for improving the clinical efficacy of chemotherapeutic drugs.
Data Availability Statement
The original contributions presented in the study are included in the article/Supplementary Material, further inquiries can be directed to the corresponding author.
Author Contributions
This study was designed by SX, WZ, and XJ. SX, XJ, and LL performed the statistical analysis. SX and XJ finished writing the article. XJ and SX provided supervision and final check. All the authors read the final version of this paper and approved it.
Conflict of Interest
The authors declare that the research was conducted in the absence of any commercial or financial relationships that could be construed as a potential conflict of interest.
Supplementary Material
The Supplementary Material for this article can be found online at: https://www.por-journal.com/articles/10.3389/pore.2021.1609985/full#supplementary-material
Supplementary Figure S1 | PPI Network.
Supplementary Table S1 | Up-regulated and down-regulated genes after integration analysis.
Supplementary Table S2 | GO enrichment analysis of the DEGs.
Supplementary Table S3 | PPI Network Analysis.
Supplementary Table S4 | List of hub genes.
Supplementary Table S5 | Raw data for Figure 6.
References
1. Grandhi, MS, Kim, AK, Ronnekleiv-Kelly, SM, Kamel, IR, Ghasebeh, MA, and Pawlik, TM. Hepatocellular Carcinoma: From Diagnosis to Treatment. Surg Oncol (2016) 25:74–85. doi:10.1016/j.suronc.2016.03.002
2. Bray, F, Ferlay, J, Soerjomataram, I, Siegel, RL, Torre, LA, and Jemal, A. Global Cancer Statistics 2018: Globocan Estimates of Incidence and Mortality Worldwide for 36 Cancers in 185 Countries. CA: A Cancer J Clinicians (2018) 68:394–424. doi:10.3322/caac.21492
3. El-Serag, HB. Epidemiology of Viral Hepatitis and Hepatocellular Carcinoma. Gastroenterology (2012) 142:1264–73. doi:10.1053/j.gastro.2011.12.061
4. Chen, Z, Xie, H, Hu, M, Huang, T, Hu, Y, Sang, N, et al. Recent Progress in Treatment of Hepatocellular Carcinoma. Am J Cancer Res (2020) 10:2993–3036.
5. Couri, T, and Pillai, A. Goals and Targets for Personalized Therapy for HCC. Hepatol Int (2019) 13:125–37. doi:10.1007/s12072-018-9919-1
6. Zhu, Y-J., Zheng, B, Wang, H-Y., and Chen, L. New Knowledge of the Mechanisms of Sorafenib Resistance in Liver Cancer. Acta Pharmacol Sin (2017) 38:614–22. doi:10.1038/aps.2017.5
7. Schlachterman, A, Craft Jr., WW, Hilgenfeldt, E, Mitra, A, and Cabrera, R. Current and Future Treatments for Hepatocellular Carcinoma. World J Gastroenterol (2015) 21:8478–91. doi:10.3748/wjg.v21.i28.8478
8. Dufour, JF, Bargellini, I, De Maria, N, De Simone, P, Goulis, I, and Marinho, RT. Intermediate Hepatocellular Carcinoma: Current Treatments and Future Perspectives. Ann Oncol (2013) 24(Suppl. 2):ii24–9. doi:10.1093/annonc/mdt054
9. Cheng, A-L, Kang, Y-K, Lin, D-Y, Park, J-W, Kudo, M, Qin, S, et al. Sunitinib Versus Sorafenib in Advanced Hepatocellular Cancer: Results of a Randomized Phase III Trial. J. Clin. Oncol. (2013) 31:4067–75. doi:10.1200/jco.2012.45.8372
10. Cervello, M, Bachvarov, D, Lampiasi, N, Cusimano, A, Azzolina, A, McCubrey, JA, et al. Molecular Mechanisms of Sorafenib Action in Liver Cancer Cells. Cell cycle (2012) 11:2843–55. doi:10.4161/cc.21193
11. Sun, W, He, B, Yang, B, Hu, W, Cheng, S, Xiao, H, et al. Genome-Wide CRISPR Screen Reveals SGOL1 as a Druggable Target of Sorafenib-Treated Hepatocellular Carcinoma. Lab Invest (2018) 98:734–44. doi:10.1038/s41374-018-0027-6
12. Llovet, JM, Ricci, S, Mazzaferro, V, Hilgard, P, Gane, E, Blanc, J-F, et al. Sorafenib in Advanced Hepatocellular Carcinoma. N Engl J Med (2008) 359:378–90. doi:10.1056/nejmoa0708857
13. Cheng, A-L, Kang, Y-K, Chen, Z, Tsao, C-J, Qin, S, Kim, JS, et al. Efficacy and Safety of Sorafenib in Patients in the Asia-Pacific Region with Advanced Hepatocellular Carcinoma: A Phase III Randomised, Double-Blind, Placebo-Controlled Trial. Lancet Oncol (2009) 10:25–34. doi:10.1016/s1470-2045(08)70285-7
14. Tang, W, Chen, Z, Zhang, W, Cheng, Y, Zhang, B, Wu, F, et al. The Mechanisms of Sorafenib Resistance in Hepatocellular Carcinoma: Theoretical Basis and Therapeutic Aspects. Sig Transduct Target Ther (2020) 5:87. doi:10.1038/s41392-020-0187-x
15. Xie, S, Jiang, X, Zhang, J, Xie, S, Hua, Y, Wang, R, et al. Identification of Significant Gene and Pathways Involved in HBV-Related Hepatocellular Carcinoma by Bioinformatics Analysis. PeerJ (2019) 7:e7408. doi:10.7717/peerj.7408
16. Kolde, R, Laur, S, Adler, P, and Vilo, J. Robust Rank Aggregation for Gene List Integration and Meta-Analysis. Bioinformatics (2012) 28:573–80. doi:10.1093/bioinformatics/btr709
17. Ritchie, ME, Phipson, B, Wu, D, Hu, Y, Law, CW, Shi, W, et al. Limma powers Differential Expression Analyses for RNA-Sequencing and Microarray Studies. Nucleic Acids Res (2015) 43:e47. doi:10.1093/nar/gkv007
18. Szklarczyk, D, Franceschini, A, Wyder, S, Forslund, K, Heller, D, Huerta-Cepas, J, et al. STRING V10: Protein-Protein Interaction Networks, Integrated over the Tree of Life. Nucleic Acids Res (2015) 43:D447–D452. doi:10.1093/nar/gku1003
19. Bader, GD, and Hogue, CW. An Automated Method for Finding Molecular Complexes in Large Protein Interaction Networks[J]. BMC bioinformatics (2003) 4:2. doi:10.1186/1471-2105-4-2
20. Lamb, J. The Connectivity Map: a New Tool for Biomedical Research. Nat Rev Cancer (2007) 7:54–60. doi:10.1038/nrc2044
21. Lamb, J, Crawford, ED, Peck, D, Modell, JW, Blat, IC, Wrobel, MJ, et al. The Connectivity Map: Using Gene-Expression Signatures to Connect Small Molecules, Genes, and Disease. Science (2006) 313:1929–35. doi:10.1126/science.1132939
22. Tang, Z, Li, C, Kang, B, Gao, G, Li, C, and Zhang, Z. GEPIA: A Web Server for Cancer and normal Gene Expression Profiling and Interactive Analyses. Nucleic Acids Res (2017) 45:W98–W102. doi:10.1093/nar/gkx247
23. Xie, S, Jiang, X, Wang, R, Xie, S, Hua, Y, Zhou, S, et al. Low‐intensity Pulsed Ultrasound Promotes the Proliferation of Human Bone Mesenchymal Stem Cells by Activating PI3K/AKt Signaling Pathways. J Cel Biochem (2019) 120:15823–33. doi:10.1002/jcb.28853
24. Xie, S-C, Zhang, J-Q, Jiang, X-L, Hua, Y-Y, Xie, S-W, Qin, Y-A, et al. LncRNA CRNDE Facilitates Epigenetic Suppression of CELF2 and LATS2 to Promote Proliferation, Migration and Chemoresistance in Hepatocellular Carcinoma. Cell Death Dis (2020) 11:676. doi:10.1038/s41419-020-02853-8
25. Zhao, Y, Zheng, X, Zheng, Y, Chen, Y, Fei, W, Wang, F, et al. Extracellular Matrix: Emerging Roles and Potential Therapeutic Targets for Breast Cancer. Front Oncol (2021) 11:650453. doi:10.3389/fonc.2021.650453
26. Mashouri, L, Yousefi, H, Aref, AR, Ahadi, AM., Molaei, F, and Alahari, SK. Exosomes: Composition, Biogenesis, and Mechanisms in Cancer Metastasis and Drug Resistance. Mol Cancer (2019) 18:75. doi:10.1186/s12943-019-0991-5
27. Kaps, L, and Schuppan, D. Targeting Cancer Associated Fibroblasts in Liver Fibrosis and Liver Cancer Using Nanocarriers. Cells (2020) 9:2027. doi:10.3390/cells9092027
28. Chen, R, Xu, X, Tao, Y, Qian, Z, and Yu, Y. Exosomes in Hepatocellular Carcinoma: A New Horizon. Cell Commun Signal (2019) 17:1. doi:10.1186/s12964-018-0315-1
29. Chen, K-F, Chen, H-L, Tai, W-T, Feng, W-C, Hsu, C-H, Chen, P-J, et al. Activation of Phosphatidylinositol 3-Kinase/Akt Signaling Pathway Mediates Acquired Resistance to Sorafenib in Hepatocellular Carcinoma Cells. J Pharmacol Exp Ther (2011) 337:155–61. doi:10.1124/jpet.110.175786
30. Tan, W, Luo, X, Li, W, Zhong, J, Cao, J, Zhu, S, et al. TNF-α Is a Potential Therapeutic Target to Overcome Sorafenib Resistance in Hepatocellular Carcinoma. EBioMedicine (2019) 40:446–56. doi:10.1016/j.ebiom.2018.12.047
31. Feng, J, Dai, W, Mao, Y, Wu, L, Li, J, Chen, K, et al. Simvastatin Re-Sensitizes Hepatocellular Carcinoma Cells to Sorafenib by Inhibiting HIF-1α/ppar-Γ/pkm2-Mediated Glycolysis. J Exp Clin Cancer Res (2020) 39:24. doi:10.1186/s13046-020-1528-x
32. Kim, M-J, Choi, Y-K, Park, SY, Jang, SY, Lee, JY, Ham, HJ, et al. PPARδ Reprograms Glutamine Metabolism in Sorafenib-Resistant HCC. Mol Cancer Res (2017) 15:1230–42. doi:10.1158/1541-7786.mcr-17-0061
33. Li, W, Dong, X, He, C, Tan, G, Li, Z, Zhai, B, et al. LncRNA SNHG1 Contributes to Sorafenib Resistance by Activating the Akt Pathway and Is Positively Regulated by miR-21 in Hepatocellular Carcinoma Cells. J Exp Clin Cancer Res (2019) 38:183. doi:10.1186/s13046-019-1177-0
34. Gao, Y, Fan, X, Li, N, Du, C, Yang, B, Qin, W, et al. CCL22 Signaling Contributes to Sorafenib Resistance in Hepatitis B Virus-Associated Hepatocellular Carcinoma. Pharmacol Res (2020) 157:104800. doi:10.1016/j.phrs.2020.104800
35. Zhang, P-F, Li, K-S, Shen, Y-H., Gao, P-T, Dong, Z-R, Cai, J-B, et al. Galectin-1 Induces Hepatocellular Carcinoma EMT and Sorafenib Resistance by Activating FAK/PI3K/AKT Signaling. Cel Death Dis (2016) 7, e2201. doi:10.1038/cddis.2015.324
36. Li, S, Dai, W, Mo, W, Li, J, Feng, J, Wu, L, et al. By Inhibiting PFKFB3, Aspirin Overcomes Sorafenib Resistance in Hepatocellular Carcinoma. Int J Cancer (2017) 141:2571–84. doi:10.1002/ijc.31022
37. Feng, J, Li, J, Wu, L, Yu, Q, Ji, J, Wu, J, et al. Emerging Roles and the Regulation of Aerobic Glycolysis in Hepatocellular Carcinoma. J Exp Clin Cancer Res (2020) 39:126. doi:10.1186/s13046-020-01629-4
38. Datta, A, Kim, H, McGee, L, Johnson, AE, Talwar, S, Marugan, J, et al. High-Throughput Screening Identified Selective Inhibitors of Exosome Biogenesis and Secretion: A Drug Repurposing Strategy for Advanced Cancer. Sci Rep (2018) 8:8161. doi:10.1038/s41598-018-26411-7
39. Nazari, M, Ghorbani, A, Hekmat-Doost, A, Jeddi-Tehrani, M, and Zand, H. Inactivation of Nuclear Factor-Κb by Citrus Flavanone Hesperidin Contributes to Apoptosis and Chemo-Sensitizing Effect in Ramos Cells. Eur J Pharmacol (2011) 650:526–33. doi:10.1016/j.ejphar.2010.10.053
40. Febriansah, R, Dyaningtyas, DPP, Sarmoko, , Nurulita, NA, Meiyanto, E, and Nugroho, AE. Hesperidin as a Preventive Resistance Agent in MCF-7 Breast Cancer Cells Line Resistance to Doxorubicin. Asian Pac J Trop Biomed (2014) 4:228–33. doi:10.1016/s2221-1691(14)60236-7
41. Samanta, D, Gilkes, DM, Chaturvedi, P, Xiang, L, and Semenza, GL. Hypoxia-Inducible Factors Are Required for Chemotherapy Resistance of Breast Cancer Stem Cells. Proc Natl Acad Sci USA (2014) 111:E5429–E5438. doi:10.1073/pnas.1421438111
42. Liu, G, Fan, X, Tang, M, Chen, R, Wang, H, Jia, R, et al. Osteopontin Induces Autophagy to Promote Chemo-Resistance in Human Hepatocellular Carcinoma Cells. Cancer Lett (2016) 383:171–82. doi:10.1016/j.canlet.2016.09.033
43. Cheng, H, Wu, Z, Wu, C, Wang, X, Liow, SS, Li, Z, et al. Overcoming STC2 Mediated Drug Resistance through Drug and Gene Co -delivery by PHB-PDMAEMA Cationic Polyester in Liver Cancer Cells. Mater Sci Eng C (2018) 83:210–7. doi:10.1016/j.msec.2017.08.075
44. Liu, J, Qiu, WC, Qiu, W, Shen, X, and Sun, G. Bioinformatics Analysis Revealed Hub Genes and Pathways Involved in Sorafenib Resistance in Hepatocellular Carcinoma. Math Biosci Eng (2019) 16:6319–34. doi:10.3934/mbe.2019315
45. Seyhan, AA, Varadarajan, U, Choe, S, Liu, W, and Ryan, TE. A Genome-wide RNAi Screen Identifies Novel Targets of Neratinib Resistance Leading to Identification of Potential Drug Resistant Genetic Markers. Mol Biosyst (2012) 8:1553–70. doi:10.1039/c2mb05512k
46. Vaziri-Gohar, A, Zheng, Y, and Houston, KD. IGF-1 Receptor Modulates FoxO1-Mediated Tamoxifen Response in Breast Cancer Cells. Mol Cancer Res (2017) 15:489–97. doi:10.1158/1541-7786.mcr-16-0176
47. Zheng, Y, Sowers, JY, and Houston, KD. IGFBP-1 Expression Promotes Tamoxifen Resistance in Breast Cancer Cells via Erk Pathway Activation. Front Endocrinol (2020) 11:233. doi:10.3389/fendo.2020.00233
48. Berberich, A, Kessler, T, Thomé, CM, Pusch, S, Hielscher, T, Sahm, F, et al. Targeting Resistance against the MDM2 Inhibitor RG7388 in Glioblastoma Cells by the MEK Inhibitor Trametinib. Clin Cancer Res (2019) 25:253–65. doi:10.1158/1078-0432.ccr-18-1580
49. Lee, C, Safdie, FM, Raffaghello, L, Wei, M, Madia, F, Parrella, E, et al. Reduced Levels of IGF-I Mediate Differential Protection of Normal and Cancer Cells in Response to Fasting and Improve Chemotherapeutic Index. Cancer Res (2010) 70:1564–72. doi:10.1158/0008-5472.can-09-3228
50. Zhu, D, Zha, X, Hu, M, Tao, A, Zhou, H, Zhou, X, et al. High Expression of TIMP-1 in Human Breast Cancer Tissues Is a Predictive of Resistance to Paclitaxel-Based Chemotherapy. Med Oncol (2012) 29:3207–15. doi:10.1007/s12032-012-0239-3
51. Schrohl, A-S, Meijer-van Gelder, ME, Holten-Andersen, MN, Christensen, IJ, Look, MP, Mouridsen, HT, et al. Primary Tumor Levels of Tissue Inhibitor of Metalloproteinases-1 Are Predictive of Resistance to Chemotherapy in Patients with Metastatic Breast Cancer. Clin Cancer Res (2006) 12:7054–8. doi:10.1158/1078-0432.ccr-06-0950
52. Sorensen, NM, Bystrom, P, Christensen, IJ, Berglund, A, Jørgen Nielsen, H, Brünner, N, et al. Timp-1 Is Significantly Associated with Objective Response and Survival in Metastatic Colorectal Cancer Patients Receiving Combination of Irinotecan, 5-fluorouracil, and Folinic Acid. Clin Cancer Res (2007) 13:4117–22. doi:10.1158/1078-0432.CCR-07-0186
53. Davidsen, ML, Würtz, S, Rømer, MU, Sørensen, NM, Johansen, SK, Christensen, IJ, et al. TIMP-1 Gene Deficiency Increases Tumour Cell Sensitivity to Chemotherapy-Induced Apoptosis. Br J Cancer (2006) 95:1114–20. doi:10.1038/sj.bjc.6603378
54. Wang, T, Lv, J-H, Zhang, X-F, Li, C-J, Han, X, and Sun, Y-J. Tissue Inhibitor of Metalloproteinase-1 Protects MCF-7 Breast Cancer Cells from Paclitaxel-Induced Apoptosis by Decreasing the Stability of Cyclin B1. Int J Cancer (2010) 126:362–70. doi:10.1002/ijc.24753
55. Tsagaraki, I, Tsilibary, EC, and Tzinia, AK. TIMP-1 Interaction with αvβ3 Integrin Confers Resistance to Human Osteosarcoma Cell Line MG-63 against TNF-α-Induced Apoptosis. Cell Tissue Res (2010) 342:87–96. doi:10.1007/s00441-010-1025-1
56. Tan, Y, Li, X, Tian, Z, Chen, S, Zou, J, Lian, G, et al. TIMP1 Down-Regulation Enhances Gemcitabine Sensitivity and Reverses Chemoresistance in Pancreatic Cancer. Biochem Pharmacol (2020) 189:114085. doi:10.1016/j.bcp.2020.114085
57. D'Costa, Z, Jones, K, Azad, A, van Stiphout, R, Lim, SY, Gomes, AL, et al. Gemcitabine-Induced TIMP1 Attenuates Therapy Response and Promotes Tumor Growth and Liver Metastasis in Pancreatic Cancer. Cancer Res (2017) 77:5952–62. doi:10.1158/0008-5472.can-16-2833
58. Bjerre, C, Vinther, L, Belling, KC, Würtz, SØ, Yadav, R, Lademann, U, et al. TIMP1 Overexpression Mediates Resistance of MCF-7 Human Breast Cancer Cells to Fulvestrant and Down-Regulates Progesterone Receptor Expression. Tumor Biol (2013) 34:3839–51. doi:10.1007/s13277-013-0969-7
59. Sonego, M, Poletto, E, Pivetta, E, Nicoloso, MS, Pellicani, R, Vinciguerra, GLR, et al. TIMP-1 Is Overexpressed and Secreted by Platinum Resistant Epithelial Ovarian Cancer Cells. Cells (2019) 9:6. doi:10.3390/cells9010006
60. Chen, J, Lan, T, Hou, J, Zhang, J, An, Y, Tie, L, et al. Atorvastatin Sensitizes Human Non-small Cell Lung Carcinomas to Carboplatin via Suppression of AKT Activation and Upregulation of TIMP-1. Int J Biochem Cel Biol (2012) 44:759–69. doi:10.1016/j.biocel.2012.01.015
61. Pennanen, PT, Sarvilinna, NS, and Ylikomi, TJ. Gene Expression Changes during the Development of Estrogen-Independent and Antiestrogen-Resistant Growth in Breast Cancer Cell Culture Models. Anticancer Drugs (2009) 20:51–8. doi:10.1097/cad.0b013e32831845e1
62. Bao, Q, Niess, H, Djafarzadeh, R, Zhao, Y, Schwarz, B, Angele, MK, et al. Recombinant TIMP-1-GPI Inhibits Growth of Fibrosarcoma and Enhances Tumor Sensitivity to Doxorubicin. Targ Oncol (2014) 9:251–61. doi:10.1007/s11523-013-0294-5
63. Zhang, L, Huang, Y, Zhuo, W, Zhu, Y, Zhu, B, and Chen, Z. Identification and Characterization of Biomarkers and Their Functions for Lapatinib-Resistant Breast Cancer. Med Oncol (2017) 34:89. doi:10.1007/s12032-017-0953-y
64. Januchowski, R, Sterzyńska, K, Zawierucha, P, Ruciński, M, Świerczewska, M, Partyka, M, et al. Microarray-based Detection and Expression Analysis of New Genes Associated with Drug Resistance in Ovarian Cancer Cell Lines. Oncotarget (2017) 8:49944–58. doi:10.18632/oncotarget.18278
65. Zhou, M, Ye, Z, Gu, Y, Tian, B, Wu, B, and Li, J. Genomic Analysis of Drug Resistant Pancreatic Cancer Cell Line by Combining Long Non-Coding RNA and mRNA Expression Profling. Int J Clin Exp Pathol (2015) 8:38–52.
66. Pang, X, Xie, R, Zhang, Z, Liu, Q, Wu, S, and Cui, Y. Identification of SPP1 as an Extracellular Matrix Signature for Metastatic Castration-Resistant Prostate Cancer. Front Oncol (2019) 9:924. doi:10.3389/fonc.2019.00924
67. Xu, X, Jiang, X, Chen, L, Zhao, Y, Huang, Z, Zhou, H, et al. Retracted: MiR-181a Promotes Apoptosis and Reduces Cisplatin Resistance by Inhibiting Osteopontin in Cervical Cancer Cells. Cancer Biother Radiopharm (2019) 34:559–65. doi:10.1089/cbr.2019.2858
68. Chen, X, Xiong, D, Ye, L, Yang, H, Mei, S, Wu, J, et al. SPP1 Inhibition Improves the Cisplatin Chemo-Sensitivity of Cervical Cancer Cell Lines. Cancer Chemother Pharmacol (2019) 83:603–13. doi:10.1007/s00280-018-3759-5
69. Liu, K, Hu, H, Jiang, H, Liu, C, Zhang, H, Gong, S, et al. Upregulation of Secreted Phosphoprotein 1 Affects Malignant Progression, Prognosis, and Resistance to Cetuximab via the KRAS/MEK Pathway in Head and Neck Cancer. Mol carcinogenesis (2020) 59:1147–58. doi:10.1002/mc.23245
70. Wang, X, Zhang, F, Yang, X, Xue, M, Li, X, Gao, Y, et al. Secreted Phosphoprotein 1 (SPP1) Contributes to Second-Generation EGFR Tyrosine Kinase Inhibitor Resistance in Non-Small Cell Lung Cancer. Oncol Res (2019) 27:871–7. doi:10.3727/096504018x15426271404407
71. Mirzaei, A, Mohammadi, S, Ghaffari, SH, Nikbakht, M, Bashash, D, Alimoghaddam, K, et al. Osteopontin B and C Isoforms: Molecular Candidates Associated with Leukemic Stem Cell Chemoresistance in Acute Myeloid Leukemia. Asian Pac J Cancer Prev (2017) 18:1707–15. doi:10.22034/APJCP.2017.18.6.1707
72. Graessmann, M, Berg, B, Fuchs, B, Klein, A, and Graessmann, A. Chemotherapy Resistance of Mouse WAP-SVT/t Breast Cancer Cells Is Mediated by Osteopontin, Inhibiting Apoptosis Downstream of Caspase-3. Oncogene (2007) 26:2840–50. doi:10.1038/sj.onc.1210096
73. Insua-Rodríguez, J, Pein, M, Hongu, T, Meier, J, Descot, A, Lowy, CM, et al. Stress Signaling in Breast Cancer Cells Induces Matrix Components that Promote Chemoresistant Metastasis. EMBO Mol Med (2018) 10. doi:10.15252/emmm.201809003
74. Hsieh, I-S, Huang, W-H, Liou, H-C, Chuang, W-J, Yang, R-S, and Fu, W-M. Upregulation of Drug Transporter Expression by Osteopontin in Prostate Cancer Cells. Mol Pharmacol (2013) 83:968–77. doi:10.1124/mol.112.082339
75. Gu, M, and Zheng, X. Osteopontin and Vasculogenic Mimicry Formation Are Associated with Response to Neoadjuvant Chemotherapy in Advanced Breast Cancer. OncoTargets Ther (2017) 10:4121–7. doi:10.2147/ott.s129414
76. Zheng, X, Naiditch, J, Czurylo, M, Jie, C, Lautz, T, Clark, S, et al. Differential Effect of Long-Term Drug Selection with Doxorubicin and Vorinostat on Neuroblastoma Cells with Cancer Stem Cell Characteristics. Cel Death Dis (2013) 4–e740. doi:10.1038/cddis.2013.264
77. Xie, X-Q, Zhao, Q-H, Wang, H, and Gu, K-S. Dysregulation of mRNA Profile in Cisplatin-Resistant Gastric Cancer Cell Line SGC7901. WJG (2017) 23:1189–202. doi:10.3748/wjg.v23.i7.1189
78. von der Heyde, S, Wagner, S, Czerny, A, Nietert, M, Ludewig, F, Salinas-Riester, G, et al. mRNA Profiling Reveals Determinants of Trastuzumab Efficiency in HER2-Positive Breast Cancer. PloS one (2015) 10:e0117818. doi:10.1371/journal.pone.0117818
79. Yamaoka, T, Ohmori, T, Ohba, M, Arata, S, Murata, Y, Kusumoto, S, et al. Distinct Afatinib Resistance Mechanisms Identified in Lung Adenocarcinoma Harboring an EGFR Mutation. Mol Cancer Res (2017) 15:915–28. doi:10.1158/1541-7786.mcr-16-0482
80. Macleod, K, Mullen, P, Sewell, J, Rabiasz, G, Lawrie, S, Miller, E, et al. Altered ErbB Receptor Signaling and Gene Expression in Cisplatin-Resistant Ovarian Cancer. Cancer Res (2005) 65:6789–800. doi:10.1158/0008-5472.can-04-2684
81. Pasini, A, Paganelli, G, Tesei, A, Zoli, W, Giordano, E, and Calistri, D. Specific Biomarkers Are Associated with Docetaxeland Gemcitabine-Resistant NSCLC Cell Lines. Translational Oncol (2012) 5:461–8. doi:10.1593/tlo.12256
82. Rodvold, JJ, Xian, S, Nussbacher, J, Tsui, B, Cameron Waller, T, Searles, SC, et al. IRE1α and IGF Signaling Predict Resistance to an Endoplasmic Reticulum Stress-Inducing Drug in Glioblastoma Cells. Sci Rep (2020) 10:8348. doi:10.1038/s41598-020-65320-6
83. Hensley, PJ, Cao, Z, Pu, H, Dicken, H, He, D, Zhou, Z, et al. Predictive and Targeting Value of IGFBP-3 in Therapeutically Resistant Prostate Cancer. Am J Clin Exp Urol (2019) 7:188–202.
84. Guix, M, Faber, AC, Wang, SE, Olivares, MG, Song, Y, Qu, S, et al. Acquired Resistance to EGFR Tyrosine Kinase Inhibitors in Cancer Cells Is Mediated by Loss of IGF-Binding Proteins. J Clin Invest (2008) 118:2609–19. doi:10.1172/JCI34588
85. Li, C, Harada, A, and Oh, Y. IGFBP-3 Sensitizes Antiestrogen-Resistant Breast Cancer Cells through Interaction with GRP78. Cancer Lett (2012) 325:200–6. doi:10.1016/j.canlet.2012.07.004
86. Qiu, N, He, Y-F., Zhang, S-M., Zhan, Y-T., Han, G-D., Jiang, M, et al. Cullin7 Enhances Resistance to Trastuzumab Therapy in Her2 Positive Breast Cancer via Degrading IRS-1 and Downregulating IGFBP-3 to Activate the PI3K/AKT Pathway. Cancer Lett (2019) 464:25–36. doi:10.1016/j.canlet.2019.08.008
87. Day, TF, Kallakury, BVS, Ross, JS, Voronel, O, Vaidya, S, Sheehan, CE, et al. Dual Targeting of EGFR and IGF1R in the TNFAIP8 Knockdown Non-Small Cell Lung Cancer Cells. Mol Cancer Res (2019) 17:1207–19. doi:10.1158/1541-7786.mcr-18-0731
88. Wang, EA, Chen, W-Y, and Wong, C-H. Multiple Growth Factor Targeting by Engineered Insulin-like Growth Factor Binding Protein-3 Augments EGF Receptor Tyrosine Kinase Inhibitor Efficacy. Sci Rep (2020) 10:2735. doi:10.1038/s41598-020-59466-6
89. Liu, YN, Tsai, MF, Wu, SG, Chang, TH, Tsai, TH, Gow, CH, et al. Acquired Resistance to EGFR Tyrosine Kinase Inhibitors Is Mediated by the Reactivation of STC2/JUN/AXL Signaling in Lung Cancer. Int J Cancer (2019) 145:1609–24. doi:10.1002/ijc.32487
90. Wang, Y, Gao, Y, Cheng, H, Yang, G, and Tan, W. Stanniocalcin 2 Promotes Cell Proliferation and Cisplatin Resistance in Cervical Cancer. Biochem biophysical Res Commun (2015) 466:362–8. doi:10.1016/j.bbrc.2015.09.029
91. Yuan, Q, Zhan, L, Zhang, L-L, Wang, Q, Liu, J, Jiang, Z-Y, et al. Stanniocalcin 2 Induces Oxaliplatin Resistance in Colorectal Cancer Cells by Upregulating P-Glycoprotein. Can J Physiol Pharmacol (2016) 94:929–35. doi:10.1139/cjpp-2015-0530
92. Miyazaki, S, Kikuchi, H, Iino, I, Uehara, T, Setoguchi, T, Fujita, T, et al. Anti-VEGF Antibody Therapy Induces Tumor Hypoxia and Stanniocalcin 2 Expression and Potentiates Growth of Human colon Cancer Xenografts. Int J Cancer (2014) 135:295–307. doi:10.1002/ijc.28686
93. Jansen, MPHM, Sas, L, Sieuwerts, AM, Van Cauwenberghe, C, Ramirez-Ardila, D, Look, M, et al. Decreased Expression of ABAT and STC2 Hallmarks ER-Positive Inflammatory Breast Cancer and Endocrine Therapy Resistance in Advanced Disease. Mol Oncol (2015) 9:1218–33. doi:10.1016/j.molonc.2015.02.006
94. Wu, W, Wang, Q, Yin, F, Yang, Z, Zhang, W, Gabra, H, et al. Identification of Proteomic and Metabolic Signatures Associated with Chemoresistance of Human Epithelial Ovarian Cancer. Int J Oncol (2016) 49:1651–65. doi:10.3892/ijo.2016.3652
95. Mora-Lagos, B, Cartas-Espinel, I, Riquelme, I, Parker, AC, Piccolo, SR, Viscarra, T, et al. Functional and Transcriptomic Characterization of Cisplatin-Resistant AGS and MKN-28 Gastric Cancer Cell Lines. PloS one (2020) 15:e0228331. doi:10.1371/journal.pone.0228331
96. Hultsch, S, Kankainen, M, Paavolainen, L, Kovanen, R-M, Ikonen, E, Kangaspeska, S, et al. Association of Tamoxifen Resistance and Lipid Reprogramming in Breast Cancer. BMC cancer (2018) 18:850. doi:10.1186/s12885-018-4757-z
97. Cruz, IN, Coley, HM, Kramer, HB, Madhuri, TK, Safuwan, NAM, Angelino, AR, et al. Proteomics Analysis of Ovarian Cancer Cell Lines and Tissues Reveals Drug Resistance-Associated Proteins. Cancer genomics & proteomics (2017) 14:35–52. doi:10.21873/cgp.20017
98. Barik, SK, Mohanty, KK, Mohanty, AK, Rawat, P, Gopal, G, Bisht, D, et al. Identification and Differential Expression of Serotransferrin and Apolipoprotein A-I in the Plasma of HIV-1 Patients Treated with First-Line Antiretroviral Therapy. BMC Infect Dis (2020) 20:898. doi:10.1186/s12879-020-05610-6
99. Li, C, Singh, B, Graves-Deal, R, Ma, H, Starchenko, A, Fry, WH, et al. Three-Dimensional Culture System Identifies a New Mode of Cetuximab Resistance and Disease-Relevant Genes in Colorectal Cancer. Proc Natl Acad Sci USA (2017) 114:E2852–E2861. doi:10.1073/pnas.1618297114
100. Fujii, K, Karpova, MB, Asagoe, K, Georgiev, O, Dummer, R, and Urosevic-Maiwald, M. Versican Upregulation in Sézary Cells Alters Growth, Motility and Resistance to Chemotherapy. Leukemia (2015) 29:2024–32. doi:10.1038/leu.2015.103
101. Hyung, SW, Lee, MY, Yu, JH, Shin, B, Jung, HJ, and Park, JMA Serum Protein Profile Predictive of the Resistance to Neoadjuvant Chemotherapy in Advanced Breast Cancers. Mol Cel Proteomics (2011) 10:M111–011023. doi:10.1074/mcp.M111.011023
102. Lin, H, Zhang, R, Wu, W, and Lei, L. Comprehensive Network Analysis of the Molecular Mechanisms Associated with Sorafenib Resistance in Hepatocellular Carcinoma. Cancer Genet (2020) 245:27–34. doi:10.1016/j.cancergen.2020.04.076
103. Huang, D, Yuan, W, Li, H, Li, S, Chen, Z, and Yang, H. Identification of Key Pathways and Biomarkers in Sorafenib-Resistant Hepatocellular Carcinoma Using Bioinformatics Analysis. Exp Ther Med (2018) 16:1850–8. doi:10.3892/etm.2018.6427
104. van Malenstein, H, Dekervel, J, Verslype, C, Van Cutsem, E, Windmolders, P, Nevens, F, et al. Long-Term Exposure to Sorafenib of Liver Cancer Cells Induces Resistance with Epithelial-to-Mesenchymal Transition, Increased Invasion and Risk of Rebound Growth[J]. Cancer Lett. (2013) 329:74–83.
105. Dekervel, J, Popovic, D, van Malenstein, H, Windmolders, P, Heylen, L, Libbrecht, L, et al. A Global Risk Score (GRS) to Simultaneously Predict Early and Late Tumor Recurrence Risk after Resection of Hepatocellular Carcinoma[j]. Transl Oncol (2016) 9:139–146.
106. Tovar, V, Cornella, H, Moeini, A, Vidal, S, Hoshida, Y, Sia, D, et al. Tumour Initiating Cells and IGF/FGF Signalling Contribute to Sorafenib Resistance in Hepatocellular Carcinoma[j]. Gut (2017) 66:530–540.
Keywords: hepatocellular carcinoma, hub gene, sorafenib resistance, integrated bioinformatics analysis, transcriptomic analysis
Citation: Jiang X, Zhang W, Li L and Xie S (2021) Integrated Transcriptomic Analysis Revealed Hub Genes and Pathways Involved in Sorafenib Resistance in Hepatocellular Carcinoma. Pathol. Oncol. Res. 27:1609985. doi: 10.3389/pore.2021.1609985
Received: 27 July 2021; Accepted: 28 September 2021;
Published: 19 October 2021.
Edited by:
Balint L. Balint, University of Debrecen, HungaryCopyright © 2021 Jiang, Zhang, Li and Xie. This is an open-access article distributed under the terms of the Creative Commons Attribution License (CC BY). The use, distribution or reproduction in other forums is permitted, provided the original author(s) and the copyright owner(s) are credited and that the original publication in this journal is cited, in accordance with accepted academic practice. No use, distribution or reproduction is permitted which does not comply with these terms.
*Correspondence: Shucai Xie, MjgyNzkxNDQ0QHFxLmNvbQ==